Preface and Acknowledgements
Peter W. Wood
President
National Association of Scholars
This report uses statistical analyses to provide further evidence that implicit bias theory, which radical activists have used to justify discriminatory and repressive “diversity, equity, and inclusion” (DEI) policies at all levels of government and in the private sector, has no scientific foundation. This report adds to and substantiates many previously published criticisms.
The National Association of Scholars (NAS) has been publicizing the dangers of the irreproducibility crisis for years, and now the crisis has played a major role in the imposition of DEI policies on the nation—and, most dangerously, in their attempted imposition on the realm of law and justice. Why does the irreproducibility crisis matter? What, practically, has it affected? Now our Exhibit A is the effects of implicit bias theory.
Before I explain implicit bias theory and its effects, I should explain the nature and the extent of the irreproducibility crisis. The crisis has had an ever more deleterious effect on a vast number of the sciences and social sciences, from epidemiology to education research. What went wrong in social psychology and implicit bias theory has gone wrong in a great many other disciplines.
The irreproducibility crisis is the product of improper research techniques, a lack of accountability, disciplinary and political groupthink, and a scientific culture biased toward producing positive results. Other factors include inadequate or compromised peer review, secrecy, conflicts of interest, ideological commitments, and outright dishonesty.
Science has always had a layer of untrustworthy results published in respectable places, as well as “experts” who were eventually shown to have been sloppy, mistaken, or untruthful in their reported findings. Irreproducibility itself is nothing new. Science advances, in part, by learning how to discard false hypotheses, which sometimes means dismissing reported data that does not stand the test of independent reproduction.
But the irreproducibility crisis is something new. The magnitude of false (or simply irreproducible) results reported as authoritative in journals of record appears to have dramatically increased. “Appears” is a word of caution, since we do not know with any precision how much unreliable reporting occurred in the sciences in previous eras. Today, given the vast scale of modern science, even if the percentage of unreliable reports has remained fairly constant over the decades, the sheer number of irreproducible studies has grown vastly. Moreover, the contemporary practice of science, which depends on a regular flow of large governmental expenditures, means that the public is, in effect, buying a product rife with defects. On top of this, the regulatory state frequently builds both the justification and the substance of its regulations upon the foundation of unproven, unreliable, and, sometimes, false scientific claims.
In short, many supposedly scientific results cannot be reproduced reliably in subsequent investigations and offer no trustworthy insight into the way the world works. A majority of modern research findings in many disciplines may well be wrong.
That was how the National Association of Scholars summarized matters in our report The Irreproducibility Crisis of Modern Science: Causes, Consequences, and the Road to Reform (2018).1 Since then we have continued our work toward reproducibility reform through several different avenues. In February 2020, we cosponsored with the Independent Institute an interdisciplinary conference on Fixing Science: Practical Solutions for the Irreproducibility Crisis, to publicize the irreproducibility crisis, exchange information across disciplinary lines, and canvass (as the title of the conference suggests) practical solutions for the irreproducibility crisis.2 We have also provided a series of public comments in support of the Environmental Protection Agency’s rule Strengthening Transparency in Pivotal Science Underlying Significant Regulatory Actions and Influential Scientific Information.3 Outside of this, we have publicized different aspects of the irreproducibility crisis by way of podcasts and short articles.4
And we have begun work on our Shifting Sands project. In May 2021, we published Keeping Count of Government Science: P-Value Plotting, P-Hacking, and PM2.5 Regulation. In July 2022, we published Flimsy Food Findings: Food Frequency Questionnaires, False Positives, and Fallacious Procedures in Nutritional Epidemiology. In July 2023, we published The Confounded Errors of Public Health Policy Response to the COVID-19 Pandemic.5 This report, Zombie Psychology, Implicit Bias Theory, and the Implicit Association Test, is the fourth of four that we will publish as part of Shifting Sands, each of which will address the role of the irreproducibility crisis in different areas of government policy. In these reports we address a central question that arose after we published The Irreproducibility Crisis:
You’ve shown that a great deal of science hasn’t been reproduced properly and may well be irreproducible. How much government regulation is actually built on irreproducible science? What has been the actual effect on government policy of irreproducible science? How much money has been wasted to comply with regulations that were founded on science that turned out to be junk?
This is the sixty-four-trillion-dollar question. It is not easy to answer. Because the irreproducibility crisis has so many components, each of which could affect the research that is used to inform government policy, we are faced with many possible sources of misdirection.
The authors of Shifting Sands include these just to begin with:
- malleable research plans;
- legally inaccessible datasets;
- opaque methodology and algorithms;
- undocumented data cleansing;
- inadequate or nonexistent data archiving;
- flawed statistical methods, including p-hacking;
- publication bias that hides negative results; and
- political or disciplinary groupthink.
Each of these could have far-reaching effects on government policy—and, for each of these, the critique, if well-argued, would most likely prove that a given piece of research had not been reproduced properly, not that it had actually failed to reproduce. (Studies can be made to “reproduce,” even if they don’t really.) To answer the question thoroughly, one would need to reproduce, multiple times, to modern reproducibility standards, every piece of research that informs governmental policy.
This should be done. But it is not within our means to do so.
What the authors of Shifting Sands did instead was reframe the question more narrowly. Governmental regulation (the focus of the first Shifting Sands reports) is meant to clear a high barrier of proof. Regulations should be based on a very large body of scientific research, the combined evidence of which provides sufficient certainty to justify reducing Americans’ liberty with a governmental regulation. What is at issue is not any particular piece of scientific research but, rather, whether the entire body of research provides so great a degree of certainty as to justify regulation. If the government issues a regulation based on a body of research that has been affected by the irreproducibility crisis so as to create the false impression of collective certainty (or extremely high probability), then, yes, the irreproducibility crisis has affected government policy by providing a spurious level of certainty to a body of research that justifies a governmental regulation.
The justifiers of regulations based on flimsy or inadequate research often cite a version of what is known as the “precautionary principle.” This means that, rather than basing a regulation on science that has withstood rigorous tests of reproducibility, they base the regulation on the possibility that a scientific claim is accurate. They do this with the logic that it is too dangerous to wait for the actual validation of a hypothesis and that a lower standard of reliability is necessary when dealing with matters that might involve severely adverse outcomes if no action is taken.
This report does not deal with the precautionary principle, since the principle summons a conclusiveness that lies beyond the realm of actual science. We note, however, that an invocation of the precautionary principle is not only nonscientific but is also an inducement to accept meretricious scientific practice, and even fraud.
The authors of Shifting Sands addressed the more narrowly framed question posed above. They applied a straightforward statistical test, Multiple Testing and Multiple Modeling (MTMM), and applied it to a body of meta-analyses used to justify government research. MTMM provides a simple way to assess whether any body of research has been affected by publication bias, p-hacking, and/or HARKing (Hypothesizing After the Results are Known)—central components of the irreproducibility crisis.
In this fourth report, the authors applied this MTMM method to assess the validity of the Implicit Association Test (IAT), which is used to measure “implicit bias.” Two technical studies jointly provide further evidence that the IAT can find no significant relationship between IAT measurements and real-world behavior, either for sex or for race. The second study also shows that advocates of implicit bias theory ignore confounders—unexamined variables that affect the analyzed variables and that, when accounted for, alter their putative relationship—which explain real-world behavior far better than implicit bias. The two technical studies together provide strong evidence that any government or private policy that uses the IAT, or that depends on implicit bias theory, is using an instrument and a theory that have no relationship with the way people actually behave in the real world.
Zombie Psychology broadens our critique from federal agencies—the Environmental Protection Agency (EPA), the Food and Drug Administration (FDA), and the Centers for Disease Control and Prevention (CDC)—to all the levels of government, and private enterprises, that draw upon implicit bias theory and the IAT. In my previous introductions, I have written of the economic consequences of the irreproducibility crisis—of the costs, rising to the hundreds of billions annually, of scientifically unfounded federal regulations issued by the EPA and the FDA. I also have written about how activists within the regulatory complex piggyback upon politicized groupthink and false-positive results to create entire scientific subdisciplines and regulatory empires. Further, I have written, particularly with reference to COVID-19 health policy and the CDC, of the deep connection between the irreproducibility crisis and the radical-activist state by means of intervention degrees of freedom—the freedom of radical activists in federal bureaucracies to make policy, unrestrained by law, prudence, consideration of collateral damage, offsetting priorities, our elected representatives, or public opinion.
In Zombie Psychology, the Shifting Sands authors make clear that radical activists do not solely weaponize the irreproducibility crisis via federal regulatory agencies. They also work through state law, city regulation, and private sector policy. They seek to subordinate the operation of our courts to cooked “statistical associations”—and the extension of the irreproducibility crisis to our judicial system bids to be a worse threat to our liberty than the subordination of federal regulatory agencies. The irreproducibility crisis of modern science intermingles with every aspect of the radical campaign to revolutionize our republic.
Zombie Psychology, as its predecessors, suggests a series of policy reforms to address this aspect of the irreproducibility crisis. All of these are well-advised. But I want to pause for a moment, in this last of my Shifting Sands introductions, to consider some of the broader implications of these reports, as well as their connection with the NAS’s mission.
The Shifting Sands reports focus upon how the irreproducibility crisis has distorted public policy and upon public policy solutions to restore good government and protect individual liberty. The NAS’s ideal of virtuous citizenship motivates our broader concern with public policy. More specifically, we want to protect the republic at large from the grave errors of the academy. We believe ourselves morally obliged to alert the public to the role that academic scientists have played in entangling government policy with the irreproducibility crisis.
The recommendations in Shifting Sands have centered on ways to reform the machinery of government to end the politicized weaponization of the irreproducibility crisis. I am keenly aware that another strand of science policy reform centers instead on removing federal money entirely from the conduct of science, as the only effective way to bring about scientific reform—and I am aware not least because other NAS writings champion this strategy.6 Neither the NAS nor I are committed exclusively to either strategy. What I do believe is that American science policy needs drastic reform—and I am delighted that the NAS can present to policymakers and the public more than one option for effecting said reform.
We also published the Shifting Sands reports to persuade the public not to defer too much to scientific authority. It isn’t crazy for laymen to think that experts know their own business and to think that government policymakers ought to listen to experts. But politicized and self-interested scientists have badly abused their so-called expertise for generations, to the detriment of public welfare and public liberty. The public needs the self-confidence to scrutinize so-called “expert judgment.” The Shifting Sands reports provide a tool throughout that any reader can use—a series of simple p-value plots in which a 45-degree line shows that the so-called expert science is bunkum. I am proud that Shifting Sands provides solid science that can be understood and used by every American citizen to hold the cabal of experts to account. I hope this will be a model for all science policy reports—that they will use representations of scientific research that are intuitive and easy to grasp and that serve the cause of American liberty and self-government.
The NAS, ultimately, seeks to reform the academy’s practice of science for its own sake. It isn’t good for scientists to stop searching for the truth and instead to work to change policy and receive grant money. It is a profound corruption. We work to reform the practices of scientists because we want them to return to their better angels—to seek truth, no matter where it leads, rather than to impose policies on their fellow citizens via the machinery of government. The NAS wishes to improve scientific practices as part of its larger goal to depoliticize the academy—which we work toward not least because it is bad for the souls of professors, and the soul of the academy, to seek power instead of truth.
And we do not do so by losing faith in science. The authors of Shifting Sands join the distinguished cadre of “meta-researchers,” and scientists working within their separate disciplines, who work to ameliorate the irreproducibility crisis by reforming the practice of science. Neither the NAS nor I think that science is the only means by which to seek and apprehend the truth. But science does provide a unique means of apprehending the truth, and the NAS and I are at one in our delight that science’s truth-seeking resources are at work to correct the pitfalls into which too many scientists have fallen. The way out of the shifting sands of modern science is not to reject science but to set it on a proper foundation. Shifting Sands has contributed to the great campaign of this generation of scientists, and I am proud that the NAS has been able to support this work.
*
Zombie Psychology puts into layman’s language the results of several technical studies by members of the Shifting Sands team of researchers, S. Stanley Young and Warren Kindzierski. Some of these studies have been accepted by peer-reviewed journals; others have been submitted and are under review. As part of the NAS’s own institutional commitment to reproducibility, Young and Kindzierski pre-registered the methods of their technical studies. And, of course, the NAS’s support for these researchers explicitly guaranteed their scholarly autonomy and the expectation that these scholars would publish freely, according to the demands of data, scientific rigor, and conscience.
Zombie Psychology is the fourth of four scheduled reports, each critiquing different aspects of the scientific foundations of government policy. The NAS intends these four reports, collectively, to provide a substantive, wide-ranging answer to the question What has been the actual effect on government policy of irreproducible science?
I am deeply grateful for the support of many individuals who made Shifting Sands possible. The Arthur N. Rupe Foundation provided the funding for Shifting Sands—and, within the Rupe Foundation, Mark Henrie’s support and goodwill got this project off the ground and kept it flying. David Acevedo copyedited Zombie Psychology with exemplary diligence and skill. David Randall, the NAS’s director of research, provided staff coordination for Shifting Sands—and, of course, Stanley Young has served as director of the Shifting Sands Project. Reports such as these rely on a multitude of individual, extraordinary talents.
Executive Summary
A great deal of modern scientific research uses statistical methods guaranteed to produce flawed statistics that can be disguised as real research. Scientists’ use of flawed statistics and editors’ complaisant practices both contribute to the mass production and publication of irreproducible research in a wide range of scientific disciplines.
This crisis poses serious questions for policymakers. How many federal regulations reflect irreproducible, flawed, and unsound research? How many grant dollars have funded irreproducible research? How widespread are research integrity violations? Most importantly, how many government regulations based on irreproducible science harm the common good?
The National Association of Scholars’s (NAS) project Shifting Sands: Unsound Science and Unsafe Regulation examines how irreproducible science negatively affects select areas of government policy and regulation. We also seek to demonstrate procedures that can detect irreproducible research.
Implicit bias theory is based on the Implicit Association Test (IAT) measurement. This fourth policy paper in the Shifting Sands project had two objectives: (1) to examine implicit bias theory and its use in practice, and (2) to perform two technical studies of the reproducibility and predictability of the IAT measurement in research.
The two technical studies use a method—p-value plotting—as a severe test to assess the validity (and reproducibility) of specific research claims based on IAT measurements. The first technical study focused on assessing a research claim for IAT−real-world behavior correlations relating to racial bias of whites toward blacks in general.
The second technical study focused on assessing a research claim for IAT−real-world behavior correlations relating to sex bias—bias of males toward females—in high-ability careers. The second study also looked at confounders—unexamined variables that affect the analyzed variables and that, when accounted for, alter their alleged relationship—that implicit bias theory should have considered and that further weaken that theory’s evidentiary basis.
Our examination of implicit bias theory and its use in practice finds that a growing number of researchers and legislators believe that policies based on this theory have been at best useless and at worst actively harmful. Furthermore, policies devised to reduce implicit bias seem to be either ineffective or counterproductive.
Our technical studies examining the use of the IAT measurement in research—the tool that supposedly best measures implicit bias—find that the IAT does not appear to measure implicit bias accurately or reliably. Our first technical study finds that there is a lack of correlation between the IAT measurement and real-world behaviors of whites toward blacks in general.
Our second technical study finds that there is a lack of correlation between the IAT measurement and real-world behaviors of males toward females in high-ability careers. The findings of both technical studies reinforce the notion that implicit bias measures have little or no ability to explain race and sex differences.
Policymakers at every level have introduced laws and regulations based on implicit bias theory—federal bureaucrats, governors, state lawmakers, city officials, executives of professional associations, and more. The citizens of a free republic should not allow such policies to rule them. Since implicit bias theory and its works have been revealed to be hollow pseudoscience, policymakers should work at once to remove the infringements of liberty undertaken in its name.
We offer four recommendations that are abstract principles designed to guide policymakers and the public in every venue to right the massive wrongs imposed by laws and regulations based on implicit bias theory. These are described further in the report:
- Rescind all laws, regulations, and programs based on implicit bias theory.
- Establish a federal commission to determine what grounds should be used to cite social science research (i.e., the field of research used to justify implicit bias theory and other like theories).
- Establish federal and state legislative committees to oversee social scientific support for proposed laws and regulations.
- Support education for lawyers and judges on the irreproducibility crisis, social science research, and best legal and judicial practices for assessing social science research and the testimony of expert witnesses.
We have subjected the science underpinning implicit bias theory to serious scrutiny. At present, we believe that policymakers and the public can enact substantial reform if they follow the principle that puts individual responsibility and the rule of the law above the hollow pseudoscience of implicit bias theory.
Governments should use the very best science—whatever the regulatory consequences. Scientists should use the very best research procedures—whatever results they find in the social scientific study of human behavior. Those principles are the twin keynotes of this report. The very best science and the very best research procedures require building evidence on the solid rock of transparent, reproducible, and actually reproduced scientific inquiry, not on shifting sands.
Introduction
Anthony Greenwald and Mahzarin Banaji, creators of the Implicit Association Test (IAT), held a press conference along with Brian Nosek in 1998 to publicize the IAT and announce the launch of the Project Implicit website. At the press conference, they claimed that the race IAT revealed unconscious prejudice that affects “90 to 95 percent of people.” Greenwald and Banaji expressed hope that “the test ultimately can have a positive effect despite its initial negative impact. The same test that reveals these roots of prejudice has the potential to let people learn more about and perhaps overcome these disturbing inclinations.”7 Publicity surrounding the publication of the first IAT study led to stories in Psychology Today, the Associated Press, and the New York Times. These articles generated further articles and further publicity for the IAT. Mitchell judges that “a review of the public record leaves little doubt that the seminal event in the public history of the implicit prejudice construct was the introduction of the IAT in 1998, followed closely by the launching of the Project Implicit website in that same year.”8
Implicit bias theory draws upon a series of pivotal psychology articles in the 1990s, above all the 1995 article by Anthony Greenwald and Mahzarin Banaji that first defined the concept of implicit or unconscious bias.9 These articles argued, drawing upon the broader theory of implicit learning,10 that individuals’ behavior was determined regardless of their individual intent, by “implicit bias” or “unconscious bias.” These biases significantly and pervasively affected individuals’ actions and were irremovable, or very difficult to remove, by conscious intent. Notably, researchers measured such biases in terms of race and sex—the categories of identity politics that fit with radical ideology and that were at issue in contemporary antidiscrimination law.
In 2020, Lee Jussim summarized the real-world context of implicit bias research and the IAT:
- The coiners of implicit bias made public claims far beyond the scientific evidence.
- Activists find implicit bias and implicit bias training politically useful, associated consultants find it financially lucrative, and bureaucrats find it useful as a way to address rhetorical and legal accusations of discriminatory behavior.
- Scientific and activist bias overstates the power and pervasiveness of implicit bias.11
In that same year, Jussim also noted that “defining associations of concepts in memory as ‘bias’ imports a subterranean assumption that there is something wrong with those associations in the absence of empirical evidence demonstrating wrongness.”12 Jussim added that
modern IAT scores cannot possibly explain slavery, Jim Crow, or the long legacy of their ugly aftermaths. Furthermore, there was nothing “implicit” about such blatant laws and norms. IAT scores in the present cannot possibly explain effects of past discrimination, because causality cannot run backwards in time. Thus, to whatever extent past discrimination has produced effects that manifest as modern gaps, modern IAT scores cannot possibly account for such effects. … To the extent that explicit prejudice causes discrimination, it may contribute to racial gaps. However, explicit prejudice is not measured by IAT scores.13
Gregory Mitchell and Philip E. Tetlock also noted in 2017 that
this degree of researcher freedom to make important societal statements about the level of implicit prejudice in American society, with no requirement that those statements be externally validated through some connection to behavior or outcomes, points to the potential mischief that attends a test such as the IAT that employs an arbitrary metric.14
These cautions by Jussim, Mitchell, and Tetlock have not been heeded. Laws and regulations based upon implicit bias theory now pervade American government and society and threaten to become omnipresent.
Reforming Government Regulatory Policy: The Shifting Sands Project
The National Association of Scholars’s (NAS) project Shifting Sands: Unsound Science and Unsafe Regulation examines how irreproducible science negatively affects select areas of government policy and regulation.15 We also aim to demonstrate procedures that can detect irreproducible research. We believe policymakers should incorporate these procedures as they determine what constitutes “best available science”—the standard that judges which research should inform government regulation.16
In Shifting Sands we use the same analysis strategy for all our policy papers⸺p-value plotting (a visual form of Multiple Testing and Multiple Modeling (MTMM) analysis)⸺as a way to demonstrate weaknesses in the government’s use of meta-analyses. MTMM corrects for statistical analysis strategies that produce a large number of false-positive statistically significant results—and, since irreproducible results from base studies produce irreproducible meta-analyses, MTMM allows us to detect these irreproducible meta-analyses.17
In other words, a great deal of modern scientific research uses statistical methods guaranteed to produce statistical hallucinations that can be disguised as real research. P-value plotting provides a means to simply look at the results of a body of research and see whether it is based on these statistical hallucinations.
In general, scientists are at least theoretically aware of the danger of the mass production of false-positive research results, although they have done far too little to correct their professional practices. Methods to adjust for MTMM have existed for decades. The Bonferroni method simply adjusts the p-value by multiplying the p-value by the number of tests. Westfall and Young provide a simulation-based method for correcting an analysis for MTMM.18
In practice, however, far too much “research” simply ignores the danger of the wholesale creation of false-positive results. Researchers can use MTMM until they find an exciting result to submit to the editors and referees of a professional journal—in other words, they can p-hack.19 Editors and referees, eager to publish high-profile, “groundbreaking” research, have an incentive to trust, far too credulously, that researchers have done due statistical diligence, so they can publish exciting papers and have their journal recognized in the mass media.20
Much contemporary psychological research is a component of this larger irreproducibility crisis, which has led to the mass production and publication of irreproducible research.21 Many improper scientific practices contribute to the irreproducibility crisis, including poor applied statistical methodology, incomplete or inaccurate data reporting, publication bias (the skew toward publishing exciting, positive results), fitting the hypotheses to the data after looking at the data, and endemic groupthink.22 Far too many scientists use these and other improper scientific practices, including an unfortunate portion who commit deliberate data falsification.23 The entire incentive structure of the modern complex of scientific research and regulation now promotes the mass production of irreproducible research.24
A large number of scientists themselves have lost overall confidence in the body of claims made in scientific literature.25 The ultimately arbitrary decision to declare p<0.05 as the standard of “statistical significance” has contributed extraordinarily to this crisis. Most cogently, Boos and Stefanski have shown that an initial result likely will not replicate at p<0.05 unless it possesses a p-value below 0.01, or even 0.001.26 Numerous other critiques concerning the p<0.05 problem have been published.27 Many scientists now advocate changing the definition of statistical significance to p<0.005.28 But even here, these authors assume only one statistical test and near-perfect study methods.
Researchers themselves have become increasingly skeptical of the reliability of claims made in contemporary published research.29 A 2016 survey found that 90% of surveyed researchers believed that modern scientific research was subject to either a major (52%) or a minor (38%) crisis in reliability.30 Begley reported in Nature that 47 of 53 research results in experimental biology could not be replicated.31 A coalescing consensus of scientific professionals realizes that a large portion of “statistically significant” claims in scientific publications, perhaps even a majority in some disciplines, is false—and certainly should not be trusted until such claims are reproduced.32
Shifting Sands aims to demonstrate that the irreproducibility crisis has affected so broad a range of government regulation and policy that government agencies (and private institutions and private enterprises) should now thoroughly modernize the procedures by which they judge “best available science.” Agency regulations should address all aspects of irreproducible research, including the inability to reproduce:
- the research processes of investigations;
- the results of investigations; and
- the interpretation of results.33
Our common approach supports a comparative analysis across different subject areas while allowing for a focused examination of one dimension of the effect of the irreproducibility crisis on government agencies’ policies and regulations.
Keeping Count of Government Science: P-Value Plotting, P-Hacking, and PM2.5 Regulation focused on irreproducible research in environmental epidemiology that informs the U.S. Environmental Protection Agency’s policies and regulations.34
Keeping Count of Government Science: Flimsy Food Findings: Food Frequency Questionnaires, False Positives, and Fallacious Procedures in Nutritional Epidemiology focused on irreproducible research in nutritional epidemiology that informs much of the U.S. Food and Drug Administration’s nutrition policy.35
Keeping Count of Government Science: The Confounded Errors of Public Health Policy Response to the COVID-19 Pandemic focused on the failures of the U.S. Centers for Disease Control and Prevention and the National Institutes of Health (NIH) to consider empirical evidence available in the public domain early in the pandemic. These mistakes eventually contributed to a public health policy that imposed substantial economic and social costs on the United States, with little or no public health benefit.36
Zombie Psychology
The first two Shifting Sands reports discussed the economic consequences of the irreproducibility crisis—the costs, rising to the hundreds of billions annually, of scientifically unfounded federal regulations issued by the Environmental Protection Agency (EPA) and the Food and Drug Administration (FDA)—and how activists within the regulatory complex piggyback upon politicized groupthink and false-positive results to create entire scientific subdisciplines and regulatory empires. The third Shifting Sands report brought into focus the deep connection between the irreproducibility crisis and the radical-activist state via intervention degrees of freedom—the freedom of radical activists in federal bureaucracies to make policy, unrestrained by law, prudence, consideration of collateral damage, offsetting priorities, our elected representatives, or public opinion.
This fourth and final Shifting Sands report explores how radical activists have used implicit bias theory as the justification for policies by the federal, state, and local government, and by American private institutions and enterprises, to remake American government and society. Most dangerously, as we shall see below, implicit bias theory is being used to corrupt the realm of law and justice by replacing individual proof of guilt with “proof” by statistical association and to thereby degrade the rule of law, due process, the presumption of innocence, and individual responsibility. It also corrupts the ideal of justice—that the courts shall give to each individual what he is due in law, as an irreducible component of the aspiration to provide justice to all mankind. Implicit bias is the tool of those who act to destroy American law and American justice.
Zombie Psychology provides an overview of the career of implicit bias theory and the IAT measurement, as well as an overview of the critiques of both the theory and the measurement. Zombie Psychology then summarizes two technical studies, which apply Multiple Testing and Multiple Modeling analysis to create p-value plots to assess the validity of the IAT. The first technical study focuses on assessing claims for IAT−real-world behavior correlations relating to race, and the second technical study focuses on assessing claims for IAT−real-world behavior correlations relating to sex. These two studies together provide further evidence that the IAT is insufficient for its two main uses of measuring implicit bias according to race and sex. The second study also highlights confounders—unexamined variables that affect the analyzed variables and that, when accounted for, alter their putative relationship—that implicit bias theory should have considered and that further weaken this theory’s evidentiary basis. We then conclude with recommendations for policy changes, to preserve American government and society—and above all our legal system—from policies based on implicit bias theory.
The Career of Implicit Bias Theory
Origins
“Implicit bias,” or “unconscious bias,” is partly a product of psychological science and partly a product of “antidiscrimination” advocates seeking a work-around on existing constitutional law. The Constitution prohibits individual discrimination; “implicit bias” provides a putatively scientific loophole to justify and to allow American institutions to discriminate.
As noted in the introduction, the psychology of implicit bias draws upon a series of pivotal psychology articles in the 1990s, above all the 1995 article of Anthony Greenwald and Mahzarin Banaji that first defined the concept of implicit or unconscious bias.37 These articles argued, drawing upon the broader theory of implicit learning,38 that individuals’ behavior was determined, regardless of their individual intent, by “implicit bias,” or “unconscious bias.” These biases were significant in their effects on individuals’ actions, pervasive, and irremovable, or very difficult to remove, by conscious intent. Significantly, researchers measured such biases in terms of race and sex—the categories of identity politics that fit with radical ideology and that were at issue in contemporary antidiscrimination law.
Greenwald and Banaji also promoted the Implicit Association Test as a way to measure implicit bias. The IAT is one a series of attempts by psychologists since ca. 1970 to find a way to avoid false self-reports and assess “true” individual bias. The IAT was meant to supersede the known frailties of earlier techniques, although it seems rather to have recapitulated them.39
Mitchell judges that “a review of the public record leaves little doubt that the seminal event in the public history of the implicit prejudice construct was the introduction of the IAT in 1998, followed closely by the launching of the Project Implicit website in that same year.”40 This publicity continued over the decades, notably including Banaji and Greenwald’s popularizing 2013 book, Blindspot: Hidden Biases of Good People.41 Greenwald et al. explicitly have sought to use their research to affect the operations of the law: “The central idea is to use the energy generated by research on unconscious forms of prejudice to understand and challenge the notion of intentionality in the law.”42 These researchers, and others of their colleagues, have acted in legal education, served as expert witnesses, promoted diversity trainings, promoted paid consulting services by Project Implicit, Inc., collected federal grant money, and more. In so doing, they have made bold but unsubstantiated claims about the solidity of implicit bias research and the importance of the effect of implicit bias. Acolytes have disseminated their arguments to audiences including the police, public defenders, human resource advisors, and doctors.43
Laws and regulations based upon implicit bias theory now pervade American government and society and threaten to become omnipresent.
Pervasive Adoption
Law
Radical advocates of implicit bias theory soon imported implicit bias research into the legal arena.44 It was a godsend to lawyers, and then to regulators and politicians, seeking to work around existing constitutional law. Existing antidiscrimination law had chipped away substantially at traditional legal conceptions of individual intent and responsibility, by means of “disparate impact”—the doctrine that a law, policy, or practice that disproportionately affects a protected group of people is illegal, regardless of whether the law, policy, or practice is intended to discriminate or has any other justification. Yet the legal precedents continued to affirm the importance of individual intent in large areas of antidiscrimination law. Federal antidiscrimination law generally requires proof of intent: “In most anti-discrimination cases, the plaintiff has the burden of proving the defendant acted with a purpose to discriminate, essentially eliminating an implicit bias claim.” The Supreme Court generally requires a high evidentiary standard for claims of discrimination.45
Implicit bias provided a new argument—that implicit biases were so strong that the law on individual intent was no longer sufficient. Kang took the evidence of implicit bias to justify a belief that “adding implicit bias to the story explains why even without such explicit racism, the segregation of the past can endure into the future simply by the actions of ‘rational’ individuals pursuing their self-interest with slightly biased perceptions driven by implicit associations we aren’t even aware of.”46 Grine further noted that “the tension between the science of implicit bias and the demands of the intent standard has become more evident in recent years, as social scientists have gained insights into the pervasiveness of implicit biases. … Such unconscious biases could produce discriminatory results in settings including health care, education, housing, and criminal justice.”47
The proposed answer was to change antidiscrimination law by replacing the intent standard with an implicit bias standard:
The intent standard does not arise from the text of the Equal Protection Clause or from the history of its adoption. The Davis Court embraced the standard based largely on a “floodgates” type of rationale: the Court was concerned that a broader understanding of discrimination “would be far-reaching and would raise serious questions about, and perhaps invalidate, [a wide range of laws].” … In adopting the intent standard, the Court effectively required consideration of the mind sciences in order to uphold the guarantee of equal protection under the law. It is therefore necessary to take proper account of the latest research in the mind sciences when interpreting discrimination claims raised under the Equal Protection Clause.48
The implicit bias standard would allow lawyers to seize on the law, stating that a “hostile environment” is an actionable offense under antidiscrimination law. Implicit bias raises any inequity, not least those detected by a statistical study, to be evidence of implicit bias and, hence, a hostile environment. To adopt an implicit bias standard would replace individual intent with statistical associations—disparate impact—in antidiscrimination law.
Such changes already have begun to be recognized in American legal systems. Vermont antidiscrimination law, for example, recognizes “unintentional discrimination,” which includes “microaggressions, unconscious biases, and unconsciously held stereotypes.”49
The implications of this change are extraordinary. As Blevins notes, “if government can ban individual judgment just because that judgment might be faulty, then we’ve abandoned the basic premise of limited government.”50 Kang’s rhetorical flourish of a conclusion is more telling: “We’ve met the enemy, and it is us.” His turn of phrase should be taken seriously: implicit bias justifies policies that regard the American people as enemies, in perpetuity, even absent any intent to discriminate, who should be treated in law as enemies rather than as citizens.51
And implicit bias theory already has proceeded from legal theory to actual law and regulation.
Equal Employment Opportunity Commission
No single agency authorized the recognition and the use of implicit bias in law and regulation. Yet a very important stage in the legal recognition of implicit bias was the Equal Employment Opportunity Commission’s (EEOC) 2007 initiative Eradicating Racism and Colorism from Employment, which gave legal recognition to implicit bias—and prescribed diversity training to employers as a means to address it. The EEOC filed discrimination lawsuits based on unconscious bias against Walmart and Walgreens.52 Although the suit against Walmart ultimately failed, the Walgreens suit was successful: the company settled for $24 million.53
The EEOC’s adoption of implicit bias gave lawyers a very important support in their legal use of implicit bias in the courts, if not a guaranteed victory. But few corporations have the resources or the persistence of Walmart. Corporations who wished to avoid the expenses and risks of a lawsuit were advised to adopt diversity trainings preemptively as a means to protect themselves against legal liability for “unconscious bias.”
How are prudent employers to prevent ‘unconscious bias’ or ‘subtle’ racism? How do you protect your company from a potentially devastating class action lawsuit like the one being faced by Walgreens? The best answers come from a non-binding ‘Guidance’ issued on the subject of race and color discrimination last year by the EEOC. This very broad Guidance, which suggests employers need to take serious action and affirmative steps to eradicate race discrimination, demonstrated how seriously the EEOC takes race and color discrimination. … employers must be attuned to the subtle and unconscious ways that race and color stereotypes and bias can negatively affect all aspects of an individual’s employment, such as networking, mentoring, etc.54
The power of federal antidiscrimination law has accelerated “voluntary” adoptions of implicit bias as a concept and implicit bias tests as a practice, even absent direct legislative and administrative requirements. The cost-benefit analysis of myriad private actors disseminated implicit bias training and ideology, as a low-cost way to avoid the possibility of an antidiscrimination lawsuit.
And, of course, the EEOC has continued to press its understanding of implicit bias on corporations. In 2021, the EEOC launched “a diversity, equity, and inclusion (DE&I) workshop series, starting with ‘Understanding Unconscious Bias in the Workplace.’”55 Corporations acquiesce to implicit bias theory and practice in the face of continuing pressure from the EEOC.
Implicit Bias Policies
Implicit bias has entered American policy diffusely, by means of a variety of federal administrative actions, state statutes, state administrative requirements, local ordinances, and private sector policies. Implicit bias policies advanced steadily until 2020, when their imposition accelerated significantly in the wake of the George Floyd riots. A widening number of states and localities have required “implicit bias” and “unconscious bias” trainings, in the legal profession, the police, the medical professions, and more. Academics, moreover, are preparing the way to use “implicit bias” in ever more extensive areas of American life, including the jury system, the operation of the courts, and all areas of government policy, from the health system to the schools to real estate licensure.
Government Legal Personnel
In 2016, the U.S. Department of Justice (DOJ) announced that it would “train all of its law enforcement agents and prosecutors to recognize and address implicit bias as part of its regular training curricula.” This requirement would immediately affect more than 28,000 department employees in the FBI, the Drug Enforcement Administration, the Bureau of Alcohol, Tobacco, Firearms and Explosives, the U.S. Marshals Service, and the ninety-four U.S. Attorney’s Offices. The DOJ planned to eventually train its other personnel, including prosecutors in the department’s litigating components and agents of the Office of the Inspector General. This program expanded on the DOJ’s existing work, since 2010, to provide implicit bias training to state and local law enforcement personnel, via the Office of Community Oriented Policing Services’ Fair and Impartial Policing program.56 At the state level, in 2020 the New Hampshire Office of the Attorney General required implicit bias training “for all attorneys, investigators, legal staff and victim/witness advocates in the Attorney General’s Office, all County Attorney Offices, all state agency attorneys, and all prosecutors, including police prosecutors.”57
Courts
The National Center for State Courts wrote that implicit bias justified new court policies, including policies to “provide routine diversity training that emphasizes multiculturalism and encourage court leaders to promote egalitarian behavior as part of a court’s culture,” “routinely check thought processes and decisions for possible bias,” and “assess visual and auditory communications for implicit bias.”58 California’s biennial training for judges and subordinate judicial officers now includes “a survey of the social science on implicit bias, unconscious bias, and systemic implicit bias, including the ways that bias affects institutional policies and practices,” “the administration of implicit association tests to increase awareness of one’s unconscious biases based on the characteristics listed in Section 11135,” and “inquiry into how judges and subordinate judicial officers can counteract the effects of juror implicit bias on the outcome of cases.”59 Bills to mandate judiciary implicit bias requirements also have been introduced in New Jersey and Texas.60
Jurors
Radical activists also have used implicit bias arguments to justify calls for systematic changes to jury selection and juror decision-making.61 Su argues for some version of implicit bias training for jurors.62 In 2022, Colorado’s General Assembly considered a bill that would allow “courts and opposing counsel to raise objections to the use of peremptory challenges with the potential to be based on racial or ethnic bias in criminal cases.” 63
Legal Profession
Bienias argues that implicit bias pervades the legal profession. His prescribed policies therefore include an administration of the Implicit Association Test and “behavioral changes” to “interrupt” one’s own implicit biases.64 Bienias further recommends “structural changes” in response to implicit bias, including a “commitment by management to diversity,” implicit bias training, a “commitment to women in counter-stereotypical roles,” and mentoring. Bienias argues that one’s active participation in opposing “unconscious bias” is necessary: “Good intentions are not enough; if you are not intentionally including everyone by interrupting bias, you are unintentionally excluding some.”65
Police
The U.S. Department of Justice’s Understanding Bias: A Resource Guide’s Community Relations Services Toolkit for Policing presumes the existence of implicit bias and recommends “positive contacts with members of that group [toward whom one displays implicit bias] … through ‘counter-stereotyping,’ in which individuals are exposed to information that is the opposite of the stereotypes they have about a group,” increasing “cultural competency,” and using the Implicit Association Test (IAT).66 California statute now requires that police training include a diversity education component: “The curriculum shall be evidence-based and shall include and examine evidence-based patterns, practices, and protocols that make up racial or identity profiling, including implicit bias.” A Racial and Identity Profiling Advisory Board, moreover, shall annually “conduct, and consult available, evidence-based research on intentional and implicit biases, and law enforcement stop, search, and seizure tactics.”67 Illinois’s curriculum for probationary law enforcement officers, as well as its triennial in-service training requirements, now must include “cultural competency, including implicit bias and racial and ethnic sensitivity.”68 In 2020, New Jersey Governor Phil Murphy signed legislation requiring law enforcement officers to take implicit bias training as part of their cultural diversity training curriculum.69 In 2022, the New Jersey General Assembly passed two further bills that would require police officers to undergo diversity and implicit bias training.70 Bills to mandate implicit bias requirements in policing have also been introduced in Georgia, Indiana, New Jersey, South Carolina, and South Dakota.71
Education
The U.S. Department of Education’s Guiding Principles: A Resource Guide for Improving School Climate and Discipline (2014) recommends that, “to help ensure fairness and equity, schools may choose to explore the use of cultural competence training to enhance staff awareness of their implicit or unconscious biases. … Where appropriate, schools may choose to explore using cultural competence training to enhance staff awareness of their implicit or unconscious biases and the harms associated with using or failing to counter racial and ethnic stereotypes.”72 Illinois statute now requires that school personnel take implicit bias training.73 In 2020, New Jersey required K–12 schools to include instruction on unconscious bias as part of its mandated instruction in “diversity and inclusion.”74 The New York City Department of Education holds Implicit Bias Awareness workshops: “For the past five years, over 80,000 NYC educators have attended the Implicit Bias Awareness foundational workshop, either in-person or virtually.”75 The New York City Department of Education’s Implicit Bias Team has twelve staff.76 Bills to mandate implicit bias requirements in education have also been introduced in New Jersey and Texas.77
General Government
In 2021, President Biden’s Executive Order on Diversity, Equity, Inclusion, and Accessibility in the Federal Workforce required the head of each federal agency to use training programs in order for “Federal employees, managers, and leaders to have knowledge of systemic and institutional racism and bias against underserved communities, be supported in building skillsets to promote respectful and inclusive workplaces and eliminate workplace harassment, have knowledge of agency accessibility practices, and have increased understanding of implicit and unconscious bias.”78 The U.S. State Department requires unconscious bias training for its Foreign Service selection panels, all supervisors and managers, and all Foreign Service Selection Boards and Bureau Awards coordinators.79 The Central Intelligence Agency also has imposed “unconscious bias training.”80 At the state level, the New Jersey General Assembly passed two bills in 2022 that would require state lawmakers to undergo diversity and implicit bias training.81 At the local level, Columbus, Ohio now offers a citywide training course in implicit bias,82 while San Francisco’s Ordinance 71-19 requires members of city boards and commissions and city department heads to complete the Department of Human Resources’s online implicit bias training.83
Medical
Implicit bias policies have been mandated particularly intensively in the medical fields. The Institute of Medicine, now known as the National Academy of Medicine, lent credibility to implicit bias policies in medicine in its Unequal Treatment: Confronting Racial and Ethnic Disparities in Health Care (2003), which stated that implicit bias contributed to minority populations’ poorer health outcomes.84 At the federal level, the Centers for Disease Control and Prevention prescribes implicit bias training as a way to achieve so-called “health equity” and recommends that employers “train employees at all levels of the organization to identify and interrupt all forms of discrimination.”85 Since 2019, often prompted by the supposedly disparate effects of COVID-19,86 California,87 Illinois,88 Maryland,89 Massachusetts,90 Michigan,91 Minnesota,92 New York,93 and Washington94 have all mandated implicit bias training for some or all health care workers as a prerequisite for professional licensure or renewal.95 Bills to mandate medical implicit bias requirements also have been introduced in Indiana, Nebraska, New Jersey, New York, North Carolina, Oklahoma, South Carolina, Tennessee, Texas, Vermont, Virginia, and West Virginia.96 Medicals schools such as Harvard Medical School, the Icahn School of Medicine at Mount Sinai in New York, and the Ohio State University College of Medicine have begun to offer or require implicit bias training, independent of state requirements.97
Science
At the federal level, in 2015 the Office of Science and Technology Policy and the Office of Personnel Management established the Interagency Policy Group on Increasing Diversity in the STEM Workforce by Reducing the Impact of Bias (IPG). The IPG catalogued and/or recommended unconscious bias training and implicit bias training throughout the government agencies that hire scientific workforces, including the National Institutes of Health, the Department of Energy, and NASA. The NIH was also going to apply implicit bias evaluations to its R01 grant awards. The IPG, moreover, recommended:
Proactive Use of Diversity, Equity, or Inclusion Grants: Institutions can pursue programmatic support to develop and employ policies, practices, training materials, and recruitment and retention strategies designed to mitigate any bias in higher education.
Recommended solutions to alleged sex and race bias in grant reviews included “educational intervention on implicit bias,” which, the IPG claims, “reduced faculty members’ implicit bias regarding women and leadership (as measured by the Implicit Association Test).”98 The IPG also stated that the Department of Energy
will develop and promote “bias interrupters” as a resource for managers and employees who complete training related to mitigating the impact of bias. The “bias interrupters” will be tips and practices that employees can use to improve the objectivity and quality of decisions related to hiring, promotions, career development opportunities, and performance appraisals.99
Real Estate
In 2021, California added implicit bias training to the licensure requirements for real estate brokers and real estate salesmen—both for initial applicants and for continuing education requirements—mandating that a “three-unit semester course, or the quarter equivalent thereof,” include instruction on “real estate practice, which shall include a component on implicit bias, including education regarding the impact of implicit bias, explicit bias, and systemic bias on consumers, the historical and social impacts of those biases, and actionable steps students can take to recognize and address their own implicit biases.”100 A similar bill to mandate implicit bias requirements in real estate also has been introduced in Oregon.101
Camouflaging Radical Policy
As the policies listed above suggest, implicit bias is also frequently used as a tool with which to wield antidiscrimination law in order to mandate equal outcomes for all identity groups. An increasing number of regulations and statutes loosely refer to “implicit bias” or “unconscious bias” to justify their imposition of radical egalitarian and illiberal ideology and policy on Americans. Such regulations and statutes have particularly targeted lawyers, the police, and health care personnel, but they already affect, or potentially affect, virtually every sort of state licensure. Such requirements directly divert increasingly large amounts of private funds and taxpayer dollars to pay for required “anti-bias” trainings. They also create an institutional beachhead for the radical and discriminatory identity politics ideology sometimes referred to as critical race theory (CRT) or diversity, equity, and inclusion (DEI).
Implicit bias is also an intellectual engine that has significant legal advantages. When the Trump administration issued an executive order banning CRT, along with all other discriminatory ideologies, the Labor Department issued a ruling stating that implicit bias trainings were not banned, since they were not discriminatory. Implicit bias, which justified many of these policies in the first place, has been set up as a means to preserve the heart of CRT and DEI in the face of legal bans.
Implicit bias is used to justify a wide range of intrusive, ideologically motivated DEI policies, as well as to dismiss criticism of these policies as yet more “implicit bias”:
Organizations should (a) use trainings to educate members of their organizations about bias and about organizational efforts to address diversity, equity, and inclusion; (b) prepare for, rather than accommodate, defensive responses from dominant group members; and (c) implement structures that foster organizational responsibility for diversity, equity, and inclusion goals; opportunities for high-quality intergroup contact; affinity groups for underrepresented people; welcoming and inclusive messaging; and processes that bypass interpersonal bias. …
Because of staunchly held narratives of meritocracy and fairness, the idea that organizations or American society might be unfair is challenging for many people to accept, especially members of dominant or well-represented groups. … majority group members often resist information about inequality by justifying or holding onto misperceptions of inequality. … These defensive responses also extend to support for policies. When exposed to information documenting stark racial disparities in the prison system, Whites report higher support for punitive crime policies, which produce these disparities. … As organizations launch their diversity initiatives, they should be prepared for potential reactance and expect some defensive responses. Organizations can plan in advance to document how defensiveness manifests and to respond to defensiveness by correcting misperceptions; linking diversity efforts to the organization’s mission, values, and goals; and providing incentives for reaching diversity targets. … Rather than just hosting trainings about implicit bias, organizations might consider offering activities that focus directly on helping majority group attendees recognize and address potential defensiveness.102
In 2020, supporters of mandatory diversity training noted with relief that unconscious and implicit bias trainings would not be prohibited by the Trump administration’s executive order banning government trainings that contained discriminatory concepts.
Covered contractors can continue to implement unconscious and implicit bias trainings so long as the trainings are not blame-focused or targeting specific groups, and instead broadly address the development of biases, how they manifest themselves in our daily lives and how we can combat biases. … There are plenty of other workplace diversity and inclusion trainings and dialogues that the EO does not appear to prohibit, such as those involving cultural competence, generational diversity, harassment, microaggressions, communications across differences, mindfulness and trainings unrelated to race or gender, to name a few.103
In general, implicit bias is the engine for laws that might technically skirt bans on overtly discriminatory policies.
Pushback
Since 2021, some state legislators have begun to push back against implicit bias theory. In New Hampshire, a 2021 bill sought to prohibit divisive concepts such as unconscious bias.104 In 2022, Florida enacted a law to prohibit trainings that teach that “an individual, by virtue of his or her race, color, sex, or national origin, is inherently racist, sexist, or oppressive, whether consciously or unconsciously.”105 In 2021, Tennessee enacted a law that restricted what public school teachers could discuss in Tennessee classrooms about racism, so-called “white privilege,” and unconscious bias.106 In 2023, another Tennessee bill was introduced that would prohibit implicit bias training in Tennessee public schools, Tennessee colleges and universities, the Tennessee Department of Education, and the State Board of Education.107 Bills to prohibit implicit bias requirements also have been introduced in Arizona, Arkansas, Missouri, Utah, and West Virginia.108 The resistance to implicit bias theory, however, is much newer and smaller than the campaign to impose it.
Prospects
Implicit bias theory is altering American government and society piecemeal, through a host of individual laws and regulations enacted by federal, state, and local governments, as well as by private institutions and private businesses. These initiatives now impose implicit bias trainings, implicit association tests, diversity trainings, and a wide variety of other requirements on millions of Americans. The gravest consequence is the spread of implicit bias theory to our legal system, which threatens to replace individual intent in antidiscrimination law with disparate results—statistical associations showing “inequitable outcomes.” Implicit bias theory already pervades America; its proponents are working with all-too-great success to make it omnipresent.
Implicit bias theory has gone from success to success in the political world. At the same time, a large number of researchers have subjected it to devastating intellectual critique. Virtually every aspect of implicit bias theory may lack empirical substantiation.
The Emperor Has No Clothes: Critiques of Implicit Bias Theory
Unsteady Foundations
The most profound critiques of implicit bias theory are broader critiques of psychology as a whole, and especially of the subdiscipline of social psychology.
Psychology as a discipline embraced statistics early. Psychologists in the nineteenth century, who aspired to make psychology a science, sought to use quantitative methods to make universal statements in the study of the mind. Psychologists therefore seized on statistics early, as a means of quantification that offered a way to make bold scientific arguments that acknowledged the inescapable fact that human minds varied.
Yet even beyond the fundamental critique that the ambition to make such universal statements may have no real-world foundation,109 psychology’s statistical revolution has been troubled. Psychologists played a prominent role in the unwieldy marriage of R. A. Fisher’s approach to statistics and the “frequentist” approach derived from the work of Jerzy Neyman and Egon Pearson. Theoretical inconsistency about how to treat p-values fueled a “practical” ability to design psychological-statistical experiments. Psychology suffers as a discipline from running experiments with small sample sizes, and hence low statistical power—a low probability of a significance test detecting a true effect. The irreducible difficulties in defining mental characteristics, much less in establishing their comparability from individual to individual, limit the discipline’s ability to conduct rigorous statistical experiments. It also embraces the loose definition of statistical significance at p ≤ 0.05, rather than the tighter definitions embraced by other disciplines—some branches of physics, for example, use the “five-sigma” standard of p ≤ 0.00006. The social psychology subdiscipline appears to be unusually subject to politicized groupthink. For all of these reasons, psychology, and especially social psychology, has been unusually afflicted by the irreproducibility crisis. Any psychological research conclusion based upon statistical techniques warrants especially close scrutiny of its methodological foundations.110
With particular reference to implicit bias theory, Chin further noted in 2023 that behavioral priming research, a subset of social psychology, has been largely discredited. The general discrediting of behavioral priming particularly discredits the basic framework that justifies the argument behind the use of implicit bias training to reduce prejudicial behavior:
If priming (e.g., activating concepts like race and hostility) does not affect judgments and behavior, it seems unlikely that changing people’s automatic associations will be useful in reducing either those judgments and behavior, or tendencies that should be even harder to change, such as discrimination in legal judgments. Stated differently, if automatic associations do not predict behavior, then attempting to change someone’s IAT score to change their behavior—if that is possible—seems futile.111
None of these broader critiques directly address implicit bias theory itself. Yet all of them should, at the very least, give policymakers pause before they make regulations or laws based on any theory from psychology, and in particular from social psychology.
But these broader critiques are accompanied by a great many further critiques that specifically address implicit bias theory.
Critiques of Implicit Bias Theory
Policy based on implicit bias theory has spread throughout America even as implicit bias theory’s intellectual underpinnings have come under sustained and devastating assault. Implicit bias never acquired consensus support from psychologists—some published articles argued for its validity, while others then critically examined the evidence and the theory. As it so happens, an increasing number of psychologists have provided evidence for devastating problems with implicit bias theory.
Collectively, these critiques call into doubt virtually every aspect of implicit bias theory. It is best to list them individually, to give a full sense of how comprehensively they demolish implicit bias theory. The different critiques of implicit bias theory include the following:
- Andreychik (2012) provides evidence that some “negative” implicit evaluative associations register empathy rather than prejudice;112
- Arkes (2004) offers three objections to the argument that the IAT measures implicit prejudice: “(a) The data may reflect shared cultural stereotypes rather than personal animus, (b) the affective negativity attributed to participants may be due to cognitions and emotions that are not necessarily prejudiced, and (c) the patterns of judgment deemed to be indicative of prejudice pass tests deemed to be diagnostic of rational behavior”;113
- Cone (2017) reviews evidence that “implicit evaluations can be updated in a durable and robust manner,” which undermines the presumption that implicit bias cannot be overcome and therefore has deep and enduring effects;114
- Corneille (2020a) reviews evidence that undermines the presumption behind implicit bias that “an associative/affective formation of attitudes and fears” mode of learning exists, “defined as automatic and impervious to verbal information”;115
- Corneille (2020b) concludes that scholars have used inconsistent definitions of “implicit” and recommends using a new terminology, to prevent confusion;116 following up, Corneille (2022) recommends that the terminology of “implicit bias” be replaced with “unconscious social categorization effects”;117
- Cyrus-Lai (2022) provides evidence that there is no significant employment bias against women and that, at present, “social cue-based explicit and implicit behavioral biases could be pro-male, pro-female, anti-Black, pro-Black, and so forth.” Cyrus-Lai (2022) also provides evidence that groupthink among academics has predisposed them to expect bias against women;118
- Jussim (2018) argues that stereotype accuracy, the “flip side” of implicit bias that studies the same subject matter but with reversed conclusions, has far greater support in psychological research than does implicit bias research;119 and Jussim (2020a) adds that “the associations tapped by the IAT may reflect not just cultural stereotypes, but implicit cognitive registration of regularities and realities of the social environment.”120
- Rubinstein (2018) presents data providing evidence that “individuating information can reduce or eliminate stereotype bias in implicit and explicit person perception” and that “patterns of reliance on stereotypes and individuating information in implicit and explicit person perception generally converged”;121 and
- Skov (2020) reviews evidence of unconscious/implicit sex bias in academia and concludes that “ascribing observed gender gaps to unconscious bias is unsupported by the scientific literature.”122
A further body of scholarly literature reviews the evidence critiquing implicit bias theory.123 In 2019, Gawronski observed that
(a) There is no evidence that people are unaware of the mental contents underlying their implicit biases; (b) conceptual correspondence is essential for interpretations of dissociations between implicit and explicit bias; (c) there is no basis to expect strong unconditional relations between implicit bias and behavior; (d) implicit bias is less (not more) stable over time than explicit bias; (e) context matters fundamentally for the outcomes obtained with implicit-bias measures; and (f) implicit measurement scores do not provide process-pure reflections of bias.124
In 2022, Gawronski also reviewed evidence that there is no evident equation between implicit bias and bias on implicit measures.125 In the same year, Cesario provided a forceful argument that there is no solid evidence that implicit bias, if it even exists, “plays a role in real-world disparities.”126
The collective intellectual demolition of implicit bias theory is astonishingly thorough—and matched by the parallel demolition of the Implicit Association Test.
Critiques of the Implicit Association Test
Researchers also have provided evidence for equally devastating problems with the effectiveness of the Implicit Association Test (IAT). These are as comprehensive as the foregoing critiques of implicit bias theory and are also best listed individually. A summary of the critiques of the IAT includes:
- Anselmi (2011) notes that the IAT’s reliability as a way to measure implicit prejudice is reduced because “positive words increase the IAT effect whereas negative words tend to decrease it”;127
- Blanton (2009) argues that the IAT provides little or no predictive validity for discriminatory behavior;128
- Blanton (2015a) further concludes that a significant component of what the IAT measures is random noise and trial error;129
- Blanton (2015b) provides evidence that “the IAT metric is ‘right biased,’ such that individuals who are behaviorally neutral tend to have positive IAT scores”;130
- Blanton (2017) argues that the IAT’s unreliability as a measure of individual implicit bias also will render it an unreliable measure of group-level implicit bias;131
- Bluemke (2009) provides evidence that different materials (“stimulus base rates”) alter IAT effects; the IAT, to a significant extent, measures the test questions and format, not the attitudes of test-takers;132
- Van Dessel (2020) reviews data suggesting that implicit measures research has been compromised by vague and varying definitions of key terms such as automatic and implicit measures and that implicit measures have thus far failed to measure effectively;133
- Fiedler (2006) provides evidence that, although the Implicit Association Test “appears to fulfil a basic need, namely, to reveal people’s ultimate internal motives, desires, and unconscious tendencies,” it has not properly established the evidence for implicitness or association, or for the effectiveness of the test;134
- Forscher (2019) argues that “changes in implicit measures are possible, but those changes do not necessarily translate into changes in explicit measures or behavior”;135
- Hahn (2014) concludes that the subjects of IAT tests have strong abilities to predict their IAT measures, a result that casts “doubt on the belief that attitudes or evaluations measured by the IAT necessarily reflect unconscious attitudes”;136
- LeBel (2011) reviews evidence that implicit measures possess low reliability (=consistency of a measure in multiple uses of a test), which “imply higher amounts of random measurement error contaminating the measure’s scores,” and hence low replicability;137
- Hughes (2023) provides evidence that the Affect Misattribution Procedure (AMP) does not measure implicit effects, since individuals possess a high degree of influences awareness;138
- Oswald (2015) notes that few IAT studies have been done in the real world and that the theory lacks external validity: “No amount of statistical modeling or simulation can reveal the real-world meaning of correlations between IAT measures and lab-based criteria that are in the range of 0.15 to 0.25”;139
- Van Ravenzwaaij (2011) presents evidence that “offers no support for the contention that the name-race IAT originates mainly from a prejudice based on race”;140 and
- Unkelbach (2020) reviews evidence about the IAT: “Building on a Bayesian analysis and on the non-evaluative influences in the EP paradigm, we concluded that implicit measures are more likely prone to false-positives compared to false-negatives.”141
A further body of scholarly literature reviews the evidence critiquing the IAT.142 In 2023, Blanton summarized eleven major critiques of the IAT:
- The IAT has poor test-retest reliability and is contaminated by large amounts of random error.
- The IAT is weakly correlated with other measures of implicit attitudes, indicating it has low convergent validity.
- The IAT is contaminated by method variance. The scoring algorithm for the IAT was designed to remove it, but it does not.
- The IAT was constructed so that it measures relative attitudes towards two objects, rather than an attitude towards a single object. In doing so, the IAT unnecessarily confounds attitudinal dimensions in ways that restrict modeling and inference.
- The metric of the IAT is arbitrary, with an empirically unverified zero point; it cannot support statements of bias prevalence.
- The criteria for classifying people into ordinal implicit bias categories on the IAT website are arbitrary.
- Researchers who employ IAT measures to predict discrimination often report their data in ways that suggest discriminatory bias, when it was not observed.
- The revised IAT scoring algorithm artifactually equates unreliable responding with reduced implicit bias.
- The IAT predicts behavior, on average, no better than attitude measures from the 1960s and early 1970s that caused a crisis in social psychology and forced attitude theorists to re-examine the utility of the attitude construct.
- When statistically controlling for explicit attitudes in tests of IAT predictive utility, researchers routinely employ suboptimal and outdated measures of explicit attitudes, a practice that can inflate estimates of the impact of implicit attitudes on behavior.
- The IAT is confounded by many influences other than implicit attitudes. As such, it can support false and counterproductive narratives about its effects.143
In 2021, Henry noted that “implicit associations” is too frequently taken to mean “implicit attitudes,” when that is not what the IAT measures.144 In 2017, Mitchell noted that, “absent evidence linking difference scores on the IAT to observable behaviors, and absent evidence showing that persons in the same bias categories reliably show the same behavioral patterns, it is impossible to give meaning and practical significance to IAT scores.”145 In 2020, Jussim similarly noted that too many researchers, tautologically, “appear to presume that ‘implicit bias’ means ‘whatever is measured by the IAT.’”146 In 2023, Blanton concluded that “the IAT is not a viable measure of individual differences in biases or attitudes.”147 Machery, perhaps most scathingly, concluded in 2022 that
we do not know what indirect measures measure; indirect measures are unreliable at the individual level, and people’s scores vary from occasion to occasion; indirect measures predict behavior poorly, and we do not know in which contexts they could be more predictive; in any case, the hope of measuring broad traits is not fulfilled by the development of indirect measures; and there is still no reason to believe that they measure anything that makes a causal difference. These issues would not be too concerning for a budding science; they are anomalies for a 30-year-old research tradition that has been extremely successful at selling itself to policy makers and the public at large.148
Collectively, these researchers have left the scientific credibility of the IAT in tatters.
Critiques of Policies Based on Implicit Bias Theory
Researchers have found increasing evidence that policies based on implicit bias theory don’t work. The effectiveness of a policy does not, strictly speaking, prove or disprove the truth value of a theory used to justify that policy. Yet, if a policy proves ineffective, policymakers ought to consider whether they should continue to make policies based on that theory. It should also prompt scholars to consider seriously whether the theory is itself flawed. The growing number of critiques of policies based on implicit bias theory ought to prompt such a reassessment.
So it matters that, in 2021, Paluck presented evidence that methods intended to reduce prejudice—such as diversity trainings and interventions based on implicit bias theory—are empirically dubious and minimally effective and that publication bias (journals’ preference for publishing positive results) and untransparent data may be exaggerating the effects of researched methods.149 It likewise matters that, in 2023, Lai concluded that a “day-long implicit bias-oriented diversity training that sought to increase U.S. police officers’ knowledge of biases, concerns about bias, and use of evidence-based strategies to mitigate bias … was ineffective at durably increasing concerns or strategy use.”150 Perhaps of greatest weight, in 2020 the Government Equalities Office of the United Kingdom concluded that unconscious bias and diversity training would be phased out:
To be successful in tackling discrimination, unconscious bias training should change behaviour. However, evidence suggests that attitudes and behaviours are each driven by different psychological systems, so a single intervention is unlikely to impact effectively on both. A systematic review of unconscious bias training examining 492 studies (involving more than 87,000 participants), found changes to unconscious bias measures were not associated with changes in behaviour (1). Formal assessments of bias (eg the Implicit Association Test) have also been criticised for failing to generate replicable results even when the same individuals have been re-tested (2).
Further evidence also suggests that unconscious bias training may even have detrimental effects. The Equality and Human Rights Commission found that evidence for its ability effectively to change behaviour is limited and “there is potential for back-firing effects when UBT participants are exposed to information that suggests stereotypes and biases are unchangeable.” Instructions to suppress stereotypes may not only activate and reinforce unhelpful stereotypes, they may provoke negative reactions and actually make people exacerbate their biases.
Finally, there is no recognised way of assuring the quality of unconscious bias training and multiple interventions of variable content may be given that label. This has serious implications for organisations, who risk putting funding into poor quality and ineffective training.151
It seems increasingly likely that policies based on implicit bias theory have been at best useless and at worst actively harmful.
Dead Man Walking
On the whole, the defenders of implicit bias theory and the IAT simply have not responded to the full implications of these critiques. Such defenses as they have made are not very persuasive: one defense of the IAT and implicit bias theory, for example, is that “it doesn’t follow from a particular measure being flawed that the phenomenon we are attempting to measure is not real.”152 In 2022, Greenwald et al. summarized their sense of implicit bias theory and included responses to some, although not all, of the critiques presented above. While they indeed have provided counter-arguments for some of these critiques, their response is far from adequate or persuasive. The reader must judge for himself.153
But the insufficiency of the defenders’ responses to sharp, comprehensive, and effective critique has not notably reduced their influence. Bartels noted in 2021 that most introductory psychology textbooks provided biased or partially biased coverage of the IAT: “Of the 17 texts that discussed the IAT, a minority presented any of the concerns including the lack of measurement clarity (29%), an automatic preference for White people among African Americans (12%), lack of predictive validity (12%), and lack of caution about the meaning of a score (0%); most provided students with a link to the Project Implicit website (65%).”154 In 2023, Chin surveyed “a sample of 100 law journal articles mentioning ‘implicit bias training’ published from 2017-2021. Of those 100 articles, 58 recommend implicit bias training and only 8 of those 58 express any skepticism about its effectiveness. Overall, only 19 articles express skepticism about implicit bias training.”155 The ever-growing number of regulations and laws based on implicit bias theory speaks for itself. If ever there were an undead theory, a zombie that keeps on shambling no matter how often it is struck dead, it is implicit bias theory.
Conclusion
It is dubious that such a thing as implicit bias even exists, and if there is such a thing, it is unlikely to be so hard-edged and pervasive as its proponents claim. Nor does the IAT, the tool that is supposed best to measure implicit bias, appear to measure it accurately or reliably. Policies devised to reduce implicit bias, moreover, seem to be either ineffective or counterproductive.
Our technical studies are meant to contribute to an intellectual discussion about implicit bias theory and the IAT in which the proponents of implicit bias theory seem more adept at arguing than at listening.
Technical Studies: Contribution to the Scholarly Conversation
Our two technical studies join a great many articles that critique different aspects of implicit bias theory and the Implicit Association Test (IAT). In short, these two articles utilize p-value plots constructed using datasets from the meta-analysis to assess the validity of the IAT. The first technical study focuses on assessing claims for IAT−real-world behavior correlations relating to race, and the second technical study focuses on assessing claims for IAT−real-world behavior correlations relating to sex. (Gender in the technical studies, to defer to journal requirements; usually corrected here.) These two studies together provide further evidence that the IAT is insufficient for its two main uses of measuring implicit bias in race and sex. The second study also highlights confounders—unexamined variables that affect the analyzed variables and that, when accounted for, alter their putative relationship—that implicit bias theory should have considered and that further weaken this theory’s evidentiary basis.
We will not repeat here the theoretical and technical background of our p-value plot methodology—although we do strongly urge readers to follow the links in the footnote below, to understand the nature of that background.156 What we will emphasize is that our method provides an extremely quantitative approach to critiquing implicit bias theory and the IAT. We simply assemble the evidence, from multiple studies, that the evidence that supports the IAT is simply a few false positives amid an overwhelming number of negative results. Our p-value plotting provides a striking visual representation of the weakness of the literature in favor of the IAT—the 45-degree line indicates random results in the literature’s data as a whole and, hence, a theory based on cherry-picking false positives.
We do not seek to explain why these results might be flawed—and here we refer readers to the scholarly literature we have cited above, which provides many plausible reasons. Rather, we provide a quantitative basis for skepticism that results from studying the data from the scholarly literature as a whole.
We believe this method is particularly useful for the discipline of psychology, and especially social psychology. As noted above, the irreproducibility crisis has particularly afflicted psychology as a discipline because of its penchant for running experiments with small sample sizes and, hence, low statistical power. Our approach, based on assessing meta-analyses, is therefore particularly useful for correcting for small sample sizes and low statistical power. Of course, we do not claim that this is an original approach in psychology! But we do believe that our technical studies further demonstrate the particular value of this approach, especially for assessments of implicit bias theory and the IAT. Quantitative studies and critiques of the scholarly literature as a whole, and not just of individual studies, facilitate especially well-substantiated scholarly judgments.
Our technical studies also highlight what we believe may be a useful approach for future scholars. In our second technical study, we provide p-value plots both for IAT measures of implicit bias and for sex (female−male) differences in vocational interests reported in the 2009 Su et al. meta-analysis.157 The p-value plot for the data from Su et al. produced a horizontal line, with all results meeting the psychological discipline’s definition of statistical significance; the p-value plot for the IAT measures produced a 45-degree line, with no results meeting the psychological discipline’s definition of statistical significance. The simple comparison would lead one to conclude, by quantitative judgment, that theories based on the data from Su et al. are more plausible than theories based on IAT measures.
Put more broadly, p-value plotting provides a usefully quantitative approach for comparing the plausibility of alternate theories or models to explain a given subject. To say that implicit bias theory and the IAT (or any theory or model) is unsupported by evidence naturally leads to follow-up queries, including what theories or models does the evidence best support? P-value plotting can contribute to that constructive task, in addition to the necessary clearance-work of establishing the insufficiency of implicit bias theory and the IAT.
Readers should keep these broader ambitions in mind as they read the details of the two technical studies below.
Technical Study #1: The Reproducibility of the Implicit Association Test (IAT): Meta-Analysis of Racial Bias Research Claims
Introduction
Implicit Association Test
Psychologists developed the Implicit Association Test (IAT) as a tool to measure “implict bias.”158 The IAT is a visual and speed-reaction test taken on a computer in which a subject associates words and pictures. The test speed of association is taken to measure the “strength of association” between pictures and words>. The IAT’s proponents claim that this “strength of association” measures> implicit bias (also known as unconscious bias), such as that of whites toward blacks (racial discrimination), males toward females (sex discrimination), or wealthy people toward poor people (wealth discrimination). For example, researchers have classified the majority of white Americans who have taken the IAT as anti-black—that is, they register as possessing “implicit bias” toward blacks.159
The IAT’s proponents do not claim that this test is the only measure of conscious or unconscious bias. Other established psychological tools exist that measure such biases, including explicit questionnaires160 and observations of real-world behaviors.161
A great many academic researchers in the fields of psychology, sociology, neuroscience, and social sciences use the IAT. A Google Scholar search of the phrase “Implicit Association Test” on 31 May 2024 returned about 49,500 articles and/or citations.162 These scholars frequently use the IAT and the concept of implicit or unconscious bias to explain disparities (differences) in behaviors in fields such as health care,163 education,164 employment and hiring,165 and criminal justice.166 These scholars present implicit bias as just one of many other factors that may explain disparities in these fields, including age or experience.
Many businesses use the IAT as a tool for dealing with DEI (“diversity, equity, and inclusion”) issues in the workplace.167 So, too, do many universities.168 The federal government, via presidential executive order, has imposed DEI training on federal agencies such as the Centers for Disease Control and Prevention, the Department of Education, the Department of Justice, the Office of Science and Technology Policy, and the Office of Personnel Management.169
Validity and Reliability
The IAT, as we have seen, possesses numerous problems. Many scholars over the last twenty years have criticized the validity (accuracy) and reliability (reproducibility) of the IAT.170 Psychometricians, scientists who study measurements of people’s knowledge, skills, and abilities, question whether an IAT measurement actually measures what it purports to measure. >These scholars look at relationships between an IAT measurement and measurements of actual biased behavior to establish the IAT measurement’s validity. If the IAT measure is valid, IAT measures of unconscious bias (implicit bias) should correlate with explicit measures and observations of real-world behaviors or actions that display bias.
Our case study aims to independently test the reliability (ability to reproduce) of racial bias research claims of black−white relations based on IAT and explicit measurements. In particular, it tests whether negative IAT measures of whites toward blacks correlate with real-world negative behaviors by whites toward blacks. Our case study uses statistical p-value plots171 and publicly available datasets to examine the strength of meta-analyses of research claims claiming that such correlations exist.
Methods
As a way to ensure that our own work meets reproducibility standards by preregistering our methodology, we first developed and posted a research plan for our study.172 To assess the validity of IAT measurements of racial bias, we have examined (1) the IAT itself; (2) explicit measures of bias—such as indications of attitude, belief, or preference of bias—captured in a questionnaire; and (3) observations/measurements of real-world biased behaviors or actions. ‘1’ and ‘2’ should be positively correlated with ‘3’ in order for ‘1’ and ‘2’ to be taken as valid measures—a putative measurement of bias should correlate with actual biased behavior to substantiate a claim to validity.
In 2013, Oswald et al. performed a meta-analysis of studies examining the predictive validity of (1) IAT measures and (2) explicit measures against (3) measures of real-world behaviors for a broad range of racial bias categories.173 Categories used as proxies for racial and ethnic discrimination included: brain activity, response time, micro-behavior, interpersonal behavior, person perception, and policy/political preferences. We focused on data about racial discrimination between white and black groups—specifically, on data about negative behaviors by whites toward blacks. Oswald et al.’s research claims were:
- The IAT provides little insight into who will discriminate against whom and provides no more insight than explicit measures of bias.
- Explicit measures of bias yielded predictions no worse than the IATs.
We intentionally used Oswald et al. as our case study because its publicly available datasets allowed us to employ p-value plots to confirm or refute their meta-analytic research claims. Our study only looked at two of their six categories of racial bias measures specific to black versus white groups>—micro-behavior and person perception. Oswald et al. describe real-world micro-behavior measures as: “measures of nonverbal and subtle verbal behavior, such as displays of emotion and body posture during intergroup interactions and assessments of interaction quality based on reports of those interacting with the participant or coding of interactions by observers.” They describe real-world person perception measures as: “explicit judgments about others, such as ratings of emotions displayed in the faces of minority or majority targets or ratings of academic ability.”
We extracted IAT and explicit bias meta-analytic datasets specific to black versus white groups for these two categories from the Oswald et al. supplemental files.174 Meta-analysis, which is used in many scientific and social scientific disciplines, including psychology, is a procedure that combines test statistics from individual studies that examine a particular research question.175 A meta-analysis can evaluate a research question by taking a test statistic (e.g., a correlation coefficient between two variables of interest), along with a measure of its reliability (e.g., a confidence interval), from multiple individual studies from the literature. The meta-analysis combines the test statistics to give a more reliable estimate of correlation between the two variables.
But meta-analyses are more reliable than individual studies if and only if the base studies themselves are valid. Meta-analysis is only reliable if the test statistics in the individual studies analyzed are unbiased estimates.176 If they are not unbiased, a meta-analysis just repeats the original studies’ biases. We therefore conducted an independent evaluation of the Oswald et al. published meta-analysis; in so doing, we follow the professional practice used elsewhere of conducting an independent evaluation of a meta-analysis of a particular research question to assess the statistical reproducibility of a claim coming from that field of research. 177
To conduct our independent evaluation, we first converted correlation coefficient (r) values to p-values.178 We present the resulting p-values in p-value plots, which can be used to visually check the characteristics of a set of test statistics that address the same research question. The plot, originally presented by Schweder and Spjøtvoll, is professionally well-regarded and has been cited more than five hundred times in scientific literature.179
We construct and interpret p-value plots as follows:
- We compute and order p-values from smallest to largest and plot them against the integers 1, 2, 3, …
- If the points on the plot follow an approximate 45-degree line, we conclude that the p-values resulted from a random (chance) process and that the data therefore supported the null hypothesis of no significant association.
- If the points on the plot approximately follow a line with a flat/shallow slope, where most of the p-values were small (less than 0.05), then the p-values provide evidence for a real (statistically significant) association.
- If the points on the plot exhibit a bilinear shape (divided into two lines), then the p-values used for meta-analysis are consistent with a two-component mixture, and a general (overall) claim is not supported; in addition, the p-value reported for the overall claim in the meta-analysis paper cannot be taken as valid.
In short, every 45-degree line or bilinear shape in the results below provides evidence that there are no significant associations between the tested variables.
Results
Implicit Bias (IAT)−Real-World Behavior Correlations
Here we examine correlations between IAT and negative micro-behavior measures of whites toward blacks. A positive effect is an increase; where negative effects occur, they can be taken as chance or no-effect results. Table 1 of Oswald et al.180 gives 87 correlations between IAT and micro-behavior measures of black versus white groups. Rank-ordered, the p-values computed for these 87 correlations are presented in Figure 1. Most of the p-values follow a 45-degree line in the plot, indicating randomness.
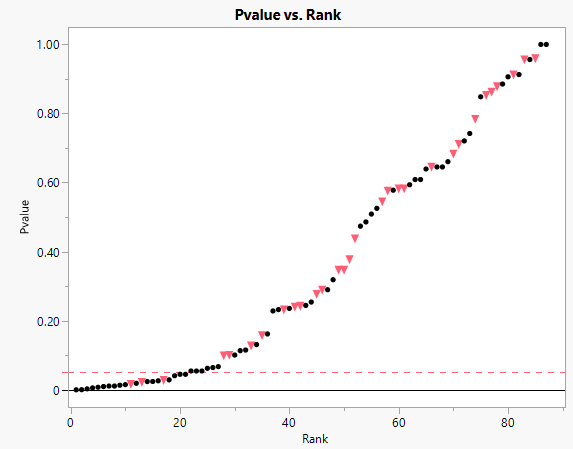
There are multiple (30) negative correlations—i.e., an IAT result is negatively correlated with micro-behavior—which are shown as downward pointing triangles (▼). Note that, according to psychology theory, a downward pointing triangle should not be possible. These data points should be considered random (chance) results.
There are multiple (21) p-values less than 0.05 in Figure 1, including three decreases. A global test of the p-value distribution for the 87 p-values using Fisher’s Combining of P-values181 gives a Chi-square of 322.51, with a p-value < 0.0001. The method assumes that p-values are independent of one another. However, this is not the case here, as multiple p-values came from the same study.182
To further evaluate the importance of small p-values in Figure 1, we computed multiplicity-adjusted p-values for the 10 smallest values using the method of Benjamini and Hochberg.183 Unadjusted p-values and false discovery rate (FDR)–adjusted p-values184 for the 10 smallest p-values are listed in Table 1. Although some of the unadjusted p-values are small, the adjusted p-values are not impressive, with only two smaller than 0.05 (see Table 1); and for these micro-behaviors, most of the variability is due to other factors.
Oswald et al. criterion description |
Unadjusted p-value |
FDR–adjusted p-value |
---|---|---|
Cold |
0.000301 |
0.022733 |
Speaking time |
0.000523 |
0.022733 |
Hand/arm movement (load) |
0.002949 |
0.085534 |
Speech errors |
0.005784 |
0.121068 |
Expressive |
0.00766 |
0.121068 |
Interactionally rigid |
0.009695 |
0.121068 |
Smiling |
0.011133 |
0.121068 |
Experiment’s rating of interaction |
0.011133 |
0.121068 |
Interactionally rigid |
0.013455 |
0.130064 |
Seating selection |
0.015185 |
0.130617 |
Regarding Oswald et al.’s 2013 findings, Oswald et al. later stated:
we found in our meta-analysis that across 87 effect sizes, the mean correlation between the race IAT and negative micro-behaviors toward African Americans (sometimes called “microaggressions”) was only .07, with a 95% confidence interval encompassing zero. This finding of a small and unreliable effect of IAT in the prediction of micro-behaviors runs counter to common assertions that implicit bias primarily expresses itself through subtle negative behaviors during interracial interactions.185
Our independent p-value plot (Figure 1) agrees with Oswald et al.’s (2015) statements above. Our plot displays considerable randomness. Even if a few of the correlations might replicate, most of them are not expected to replicate. Our finding is that IAT evidence suggesting micro-behaviors as a possible cause of racial disparities of whites toward blacks is unproven by the data and analysis.
Explicit Bias−Real-World Behavior Correlations
Table 5 of Oswald et al. (2013a) gives 83 correlations between explicit bias and micro-behavior measures. Rank-ordered p-values computed for these 83 correlations are presented in Figure 2. As with Figure 1, most of the p-values follow a 45-degree line in the Figure 2 plot, indicating randomness. There are multiple (33) negative correlations—i.e., an explicit bias result is negatively correlated with micro-behavior—which are shown as downward pointing triangles. Again, any decrease is assumed to be a random result.
We computed multiplicity-adjusted p-values for the 5 smallest values in Figure 2 using the method of Benjamini and Hochberg.186 The unadjusted p-values and false discovery rate (FDR)–adjusted p-values for the 5 smallest p-values are listed in Table 2. None of the 5 adjusted p-values are impressive (see Table 2).
A global test of the p-value distribution for the 83 p-values using Fisher’s Combining of P-values187 gives a Chi-square of 160.63, with a p-value = 0.603, indicating that nothing is going on. Keep in mind, the method assumes that the p-values are independent, and that cannot be accepted here.
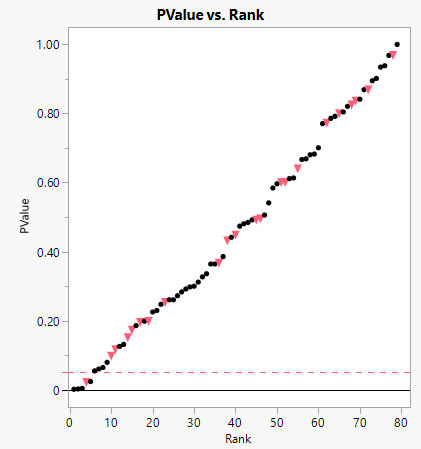
Oswald et al. criterion description |
Unadjusted p-value |
FDR–adjusted p-value |
---|---|---|
Attitudes towards blacks, Carney |
0.0190 |
0.5762 |
Attitudes towards blacks, Carney |
0.0284 |
0.5762 |
Pro-black attitudes, Heider &… |
0.0301 |
0.5762 |
Sem diff & F therm, McMonnell &… |
0.0346 |
0.5762 |
Attitudes towards blacks, Carney |
0.0604 |
0.8631 |
Oswald et al. are silent about the (lack of) correlation between explicit bias and micro-behaviors observed in their meta-analysis.188 Our p-value plot displays considerable randomness, and, although the 5 smallest unadjusted p-values are < 0.05, the adjusted p-values are > 0.05. Our p-value plot and the negative correlations indicate that none of the explicit bias measure−real-world micro-behavior correlations are real. Our finding is that even explicit bias measures are not associated with negative micro-behaviors of whites toward blacks.
Person Perception Measures
Implicit Bias (IAT)−Real-World Behavior Correlations
Here we examine correlations between IAT and negative person perception measures toward blacks. As before, a positive effect is an increase; where negative effects occur, they can be taken as chance or no-effect results. Table 1 of Oswald et al. (2013a) gives 75 correlations between IAT and person perception measures.189
Rank-ordered p-values computed for these 75 correlations are presented in Figure 3. Most of the p-values follow a 45-degree line in the plot, indicating randomness. There are multiple (26) negative correlations—i.e., an IAT result is negatively correlated with person perception measures—which are shown as downward pointing triangles. Any decrease is assumed to be a random (chance) result.
A Fisher combined p-value was computed.190 The Chi-square value was 249.82, with a p-value < 0.0001. Multiplicity-adjusted p-values were computed for the 10 smallest values in Figure 3 using the method of Benjamini and Hochberg. 191 The unadjusted p-values and false discovery rate (FDR)–adjusted p-values for the 10 smallest p-values are listed in Table 3.
Although the 10 smallest unadjusted p-values are < 0.05, the adjusted p-values are not impressive, with only one smaller than 0.05 (see Table 3). Again, the method assumes that p-values are independent of one another. This, again, is not the case here, as multiple p-values came from the same study.192
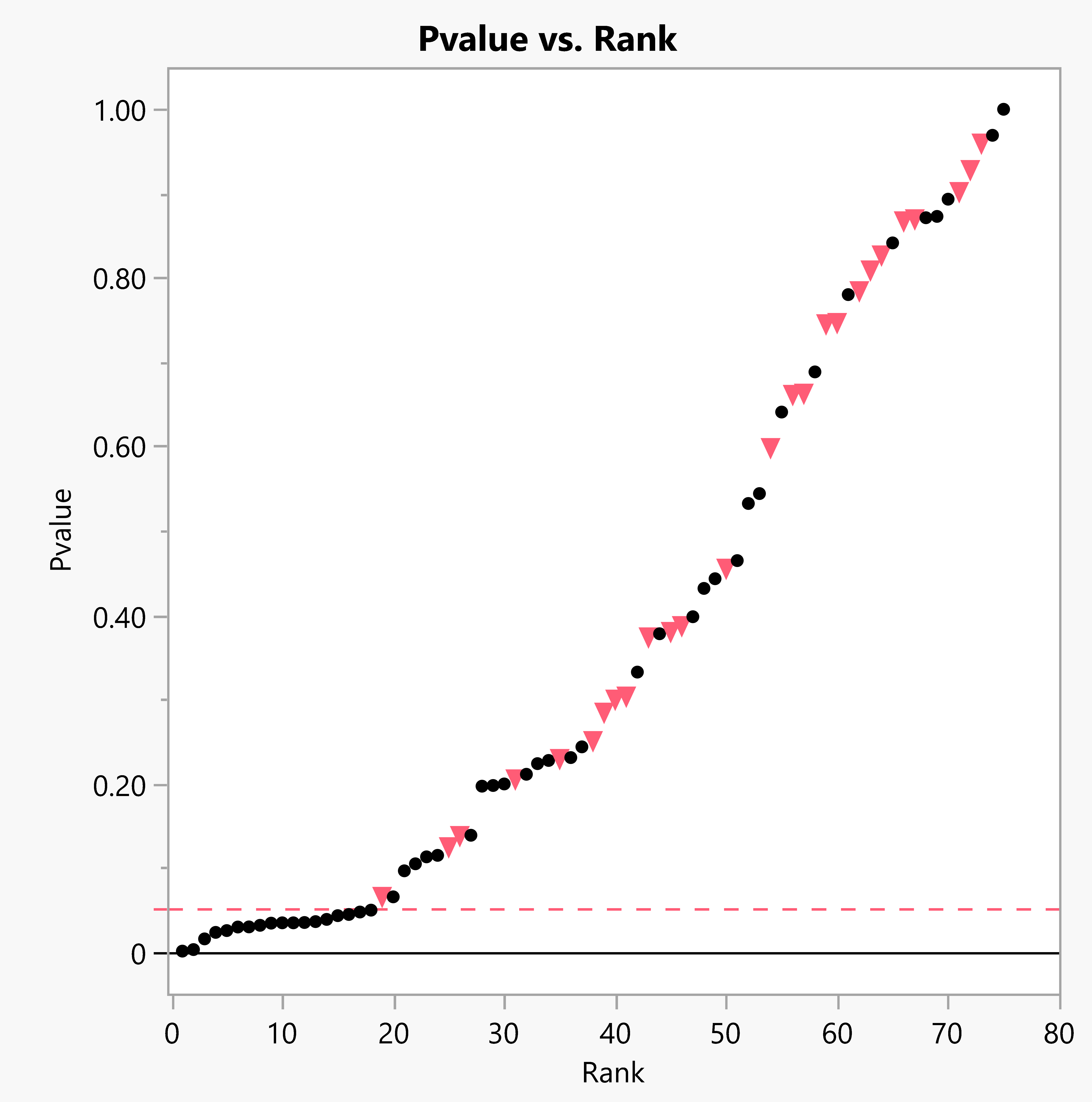
Rank |
R |
N |
Unadjusted p-value |
FDR–adjusted p-value |
---|---|---|---|---|
1 |
0.582 |
31 |
0.000429 |
0.032196 |
2 |
0.4182 |
50 |
0.002256 |
0.084608 |
3 |
0.43 |
31 |
0.014952 |
0.204054 |
4 |
0.46 |
24 |
0.022669 |
0.204054 |
5 |
0.326 |
47 |
0.024811 |
0.204054 |
6 |
0.217 |
101 |
0.029044 |
0.204054 |
7 |
-0.28 |
60 |
0.029859 |
0.204054 |
8 |
0.48 |
20 |
0.031059 |
0.204054 |
9 |
0.34 |
39 |
0.033624 |
0.204054 |
10 |
0.24 |
78 |
0.034022 |
0.204054 |
Explicit Bias−Real-World Behavior Correlations
Table 5 of Oswald et al. (2013a) gives 79 correlations between explicit bias and person perception measures. Rank-ordered p-values computed for these 79 correlations are presented in Figure 4. As with previous p-value plots, the p-values follow a 45-degree line in the Figure 4 plot, indicating randomness. There are multiple (22) negative correlations—i.e., an explicit bias result is negatively correlated with person perception—which are shown as downward pointing triangles. Again, any decrease is assumed to be a random (chance) result.
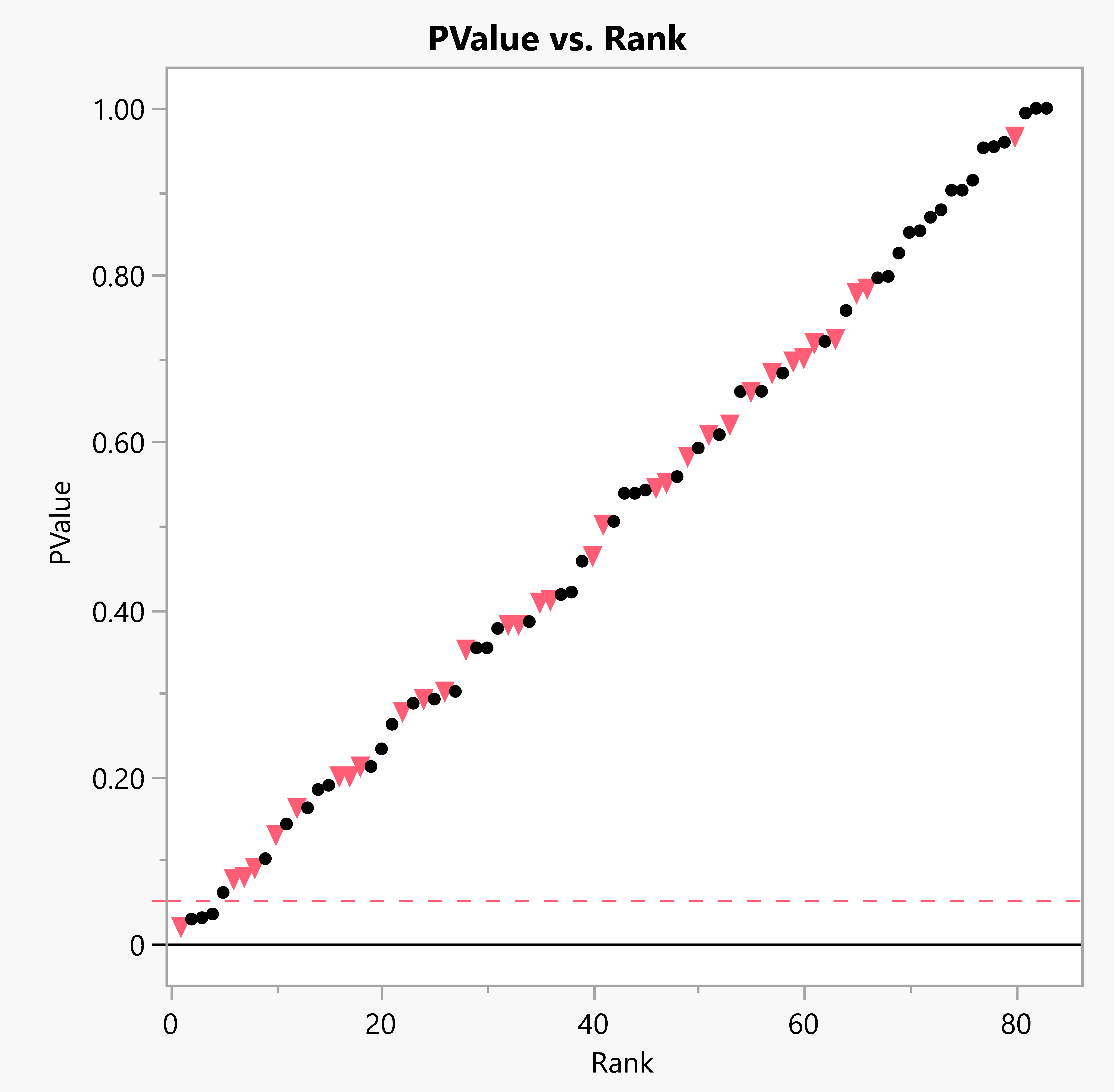
A Fisher combined p-value was computed.193 The Chi-square value was 186.54, with a p-value of 0.0600. We computed multiplicity-adjusted p-values for the 5 smallest values in Figure 4 using the method of Benjamini and Hochberg.194 The unadjusted p-values and false discovery rate (FDR)–adjusted p-values for the 5 smallest p-values are listed in Table 4. None of the 5 adjusted p-values are impressive (see Table 4).
Rank |
R |
N |
Unadjusted p-value |
FDR–adjusted p-value |
---|---|---|---|---|
1 |
0.700 |
16 |
0.001765 |
0.106167 |
2 |
0.506 |
32 |
0.002688 |
0.106167 |
3 |
0.320 |
77 |
0.004332 |
0.114069 |
4 |
-0.383 |
35 |
0.022434 |
0.380790 |
5 |
0.390 |
33 |
0.024101 |
0.380790 |
Discussion
We analyzed three aspects of the validity of an IAT measure of racial bias of whites toward blacks: (1) the IAT itself; (2) explicit measures of bias—for example, indications of attitude, belief, or preference of bias in a questionnaire; and (3) observations/measurements of real-world biased behaviors or actions. The main issue is the validity of the IAT (measure of implicit bias), which is frequently employed in academic research and used pervasively by businesses as a tool for addressing DEI issues in the workplace.
Oswald et al. point out that the IAT must be confirmed by other measurements and be able to predict real-world results if it is to claim validity as a measurement of bias.195 Our p-value plots show that the IAT does not predict real-world micro-behaviors (Figure 1) or person perception judgments (Figure 3). The IAT is a repeatable measure—that is, it generates similar results for repeated analyses of the same data. Yet we have found, just as did Oswald et al., that it does not predict real-world behaviors. We have also found that the average correlation (r) of IAT measurements reported by Oswald et al. in their meta-analysis of IAT−micro-behavior correlations specific to negative behaviors of whites toward blacks is small (0.07). The variance explained by a correlation is r2; in other words, the IAT explains less than one percent of the race variability, which is about the same explanatory effect as explicit bias. Ninety-nine percent of differences between the races is due to factors other than implicit bias.
The random behavior of the p-values depicted in our plots—the approximately 45-degree lines—also shows that, in the base studies used in the Oswald et al. meta-analysis, explicit measures of bias poorly predict real-world micro-behaviors (Figure 2) and person perception judgments (Figure 4). Here, Oswald et al. explain that the poor performance of both the IAT and measures of explicit bias are mostly consistent with “flawed instruments explanation”—that is, fundamental problems in the theories that motivated the development and use of these measurements are responsible for these measurements’ poor performance. We agree with this explanation.
Returning to the IAT, Schimmack argues that it is invalid because psychometric (statistical) analysis measures essentially the same mental phenomenon as do explicit measurements of bias.196 Dang et al. note that the person-to-person variability of the IAT is small, which means that its ability to correlate with explicit or real-world measures of bias is very limited.197 In other words, it is likely that the IAT is mostly measuring inherent human “reaction time” to a stimulus—the time it takes to detect, process, and respond to a stimulus—and that this reaction time bears little or no relationship to subconscious thinking.
Further examination of the research literature reveals that measurements of racial bias resulting from the IAT provide little or no explanatory power for actual racially biased behavior (i.e., negative behaviors of whites toward blacks). Dehon et al.’s systematic review of evidence of the correlation between physician implicit racial bias based on the IAT and real-world clinical decision-making observed no significant correlation between the race IAT and physician decision-making in eight of nine studies—i.e., results from the race IAT were not correlated with real-world physician decision-making.198 Nor, for that matter, does implicit bias as measured on the IAT appear to affect real-world behavior in several categories other than race. (See Appendix 1: IAT and Real-World Behavior.) These studies more generally support Schimmack’s position that implicit bias and explicit bias measures are essentially the same; once explicit measures are considered, nothing is gained by using implicit measures.
Our p-value plots show that the IAT does not predict real-world negative micro-behaviors (Figure 1) and person perception judgments (Figure 3) of whites toward blacks for the base studies used in Oswald et al.’s 2013 meta-analysis. Our plots also show that explicit bias measures do not predict real-world negative micro-behaviors (Figure 2) and person perception judgments (Figure 3) of whites toward blacks. Our results are consistent with Oswald et al.’s 2013 findings.
Schimmack notes that, over the past decade, it has become apparent that the empirical foundations of the implicit social-cognition paradigm are problematic.199 He states that a key imposition that inhibits researchers within a paradigm from noticing these problems is publication bias. This bias ensures that studies that are consistent with a flawed paradigm (e.g., the IAT is a valid measure of an equally valid concept of implicit bias) are published and highlighted in review articles to offer false evidence supporting the paradigm. Indeed, once positive results are obtained, even if flawed, a research claim can become canonized.200
The studies presented here support the claim that the IAT is an invalid metric when compared to real-world observations—that is, it does not measure what it claims to measure. Does an IAT add any information over and above explicit measures of bias in explaining negative white against black behaviors? The straightforward answer is that it does not add information over and above explicit measures of bias. Indeed, explicit measures of bias also offer weak explanations of white against black behaviors.
Conclusions
We independently examined three instruments of interest in a case study using statistical p-value plots regarding IAT measurements of black–white racial bias—the IAT itself; explicit measure(s) of bias; and observations/measurements of real-world biased behaviors or actions. The p-value plots exhibited considerable randomness for all IAT−real-world behavior and explicit bias−real-world behavior correlations examined. This randomness supports a lack of correlation between the IAT (implicit bias) and explicit bias measurements and real-world behaviors of whites toward blacks. These findings pertained to micro-behaviors (measures of nonverbal and subtle verbal behavior) and person perception judgments (explicit judgments about others). The findings of the p-value plots were consistent with the case study research claim that the IAT provides little insight into who will discriminate against whom. It was also observed that the amount of real-world micro-behavior variance explained by the IAT and explicit bias measurements was small, less than 1%.
Technical Study #2: The Reliability of the Sex Implicit Association Test (sIAT) for High-Ability Careers
Introduction
Background
Males outnumber females in many high-status, high-tech fields, such as science, technology, engineering, and mathematics (STEM)201 and medical academia.202 Researchers frequently assume that females and males are nearly equal or equal in all relevant aspects of ability and interest.203 They therefore often attribute sex differences in STEM and medical professorship careers to implicit (unconscious) bias.204
Psychologists developed the Implicit Association Test (IAT), a visual and speed-reaction test taken on a computer in which a person associates words with pictures, to measure implicit bias.205 The IAT claims to measure implicit bias toward a topic of interest, such as sex bias—for example, a group tendency to prefer males over females.
Researchers measure sex implicit bias with the sex Implicit Association Test (sIAT).206 Since sIAT scores indicating a sex-difference gap are reported to be large, it has been assumed that implicit bias is an important factor contributing to this gap.207
We must examine three questions to evaluate the accuracy of this belief. First, how repeatable is the sIAT? Second, does the sIAT correlate well with explicit measures of sex difference and measurements/observations of real-world sex actions? Third, how much sex-difference variance is accounted for by the sIAT? As to why these issues merit further attention, there is considerable evidence that IAT measures in general, and sIAT measures in particular, correlate poorly with explicit measures and explain very little of the variance of sex differences.208
Study Objectives
Kurdi et al. and Kurdi and Banaji recently undertook a meta-analysis of studies examining correlations between implicit social cognition (i.e., implicit bias measures based on the IAT, including implicit sex bias) and real-world measures of female and male behavior.209 These real-world measures included expressions of policy preferences, resource allocation, academic performance, subtle nonverbal behaviors, performance on interference tasks like the Stroop task, and criminal sentencing decisions. Kurdi et al. claimed that they
found significant implicit–criterion correlations (ICCs) and explicit–criterion correlations (ECCs), with unique contributions of implicit and explicit measures revealed by structural equation modeling.210
One objective of our study was to independently test the reliability (ability to reproduce) of the claim of implicit sex bias differences in high-ability careers. We used the publicly available Kurdi et al. and Kurdi and Banaji sex dataset and statistical p-value plots211 to visually inspect the reproducibility of the claim. This objective builds upon Kurdi et al.’s own skepticism about the reliability of the IAT, including the sIAT:
Statistically, the high degree of heterogeneity suggests that any single point estimate of the implicit–criterion relationship [ICC] would be misleading. Conceptually, it suggests that debates about whether implicit cognition and behavior are related to each other are unlikely to offer any meaningful conclusions.212
If the sIAT poorly predicts sex differences, and if high IAT heterogeneity renders individual predictions questionable, this raises the question of what alternate factors or variables may actually be important explainers of sex differences, as well as whether IAT users have accounted for these alternate factors in their analysis and modeling.
One such set of factors may be vocational interests. Vocational interests have been defined as: trait-like preferences to engage in activities, contexts in which activities occur, or outcomes associated with preferred activities that motivate goal-oriented behaviors and orient individuals toward certain environments.213 Su et al. noted real sex differences in STEM interests in a meta-analysis of vocational interests.214 They observed that these differences paralleled the female–male composition in STEM educational programs and occupations and may play a role in sex occupational choices and sex disparity in the STEM fields. This may also be the case for other high-ability careers like academic medicine.
A second objective of our study was to independently test the ability to reproduce the sex (female−male) differences in vocational interests reported in the Su et al. meta-analysis. Specifically, we used the Su et al. dataset and a statistical p-value plot to visually inspect the reproducibility of their claim.
Methods
As a way to ensure that our own work meets reproducibility standards by preregistering our methdology, we first developed and posted a research plan for our study at Researchers.One.215 To assess the validity of IAT measurements of sex bias, we have examined: (1) the sIAT; (2) explicit measures of bias—such as indications of attitude, belief, or preference of sex bias—captured in a questionnaire or a similar research instrument; and (3) observations/measurements of real-world sex biased behaviors or actions. Measures of ‘1’ and ‘2’ should be positively correlated with ‘3’ for ‘1’ and ‘2’ to be considered valid.
Meta-analysis, which is used in many scientific and social scientific disciplines, including psychology, is a procedure that combines test statistics from individual studies that examine a particular research question.216 A meta-analysis can evaluate a research question by taking a test statistic (e.g., a correlation coefficient between two variables of interest), along with a measure of its reliability (e.g., a confidence interval), from multiple individual studies from the literature. The meta-analysis combines the test statistics to give a more reliable estimate of the correlation between the two variables.
But meta-analyses are more reliable than individual studies if and only if the base studies themselves are valid. Meta-analysis is only reliable if the test statistics in the individual studies analyzed are unbiased estimates.217 If they are not unbiased, a meta-analysis just repeats the original studies’ biases. We therefore conducted an independent evaluation of published meta-analyses on sex implicit bias; in so doing, we follow the professional practice used elsewhere of conducting an independent evaluation of a meta-analysis of a particular research question to assess the statistical reproducibility of a claim coming from that field of research.218
We examined and extracted meta-analysis datasets from the Kurdi et al. and Kurdi and Banaji meta-analyses.219 We initially selected studies addressing sex. These selected studies had a combined sample size of 1,155.
The sex data of interest to us consisted of individual study author, year, title, journal, and correlation coefficient (r) values for three two-variable comparisons:
- implicit-criterion correlations (ICCs): an ICC is the correlation between an sIAT measurement/score and observations/measurements of real-world sex-related measures, such as sex attitude, stereotype, and identity.
- explicit-criterion correlations (ECCs): an ECC is the correlation between an explicit measure(s) of bias—such as an indication(s) of attitude, belief, or preference of sex bias—captured in a questionnaire or a similar research instrument and real-world sex-related measures.
- implicit-explicit correlations (IECs): an IEC is the correlation between implicit and explicit variable measurements.
We only used studies with data for all ICC, ECC, and IEC comparisons in our evaluation. This smaller dataset comprised a sample size of 535 from 27 individual studies. We computed mean ICC, ECC, and IEC correlation coefficient (r) values for each of the 27 studies. We then converted r values to p-values using Fisher’s Z-transformation.220
Su et al. undertook a meta-analysis examining the magnitude and variability of sex differences in vocational interests.221 Su et al. initially screened and evaluated technical manuals of vocational interest inventories from educational settings, public institutions, and private organizations (n=108), of which 47 interest inventories were selected for meta-analysis. The inventories were intended to measure vocational interests (documented assessments of people’s interests in potential careers, educational paths, and the world of work). The inventories were published in English with collections of scores from female and male populations (norm samples) from the US or combined norm samples from both the US and Canada.
The 47 inventories were published over four decades (between 1964 and 2007) and comprised a total of 81 samples consisting of 259,518 women and 243,670 men (ntotal = 503,188). Mean ages of the samples ranged from 12.5 to 42.6 years. The oldest cohort of the samples was born in 1939, and the youngest in 1987.
Su et al. calculated weighted mean effect sizes (Cohen’s d), standard deviation (SD), and lower– and upper–95 percent confidence intervals (CIs) for eleven different dimensions of vocational interests. These included: (1) interest in working with things versus people (Things-People); (2) interest in working with data versus ideas (Data-Ideas); RIASEC interests, including (3) Realistic interest in working with things and gadgets or working outdoors; (4) Investigative interest in science, including mathematics, physical and social sciences, and biological and medical sciences; (5) Artistic interest in creative expression, including writing and the visual and performing arts; (6) Social interest in helping people; (7) Enterprising interest in working in leadership or persuasive roles directed toward achieving economic objectives; (8) Conventional interest in working in well-structured environments, especially business settings, and STEM interests; (9) Science; (10) Mathematics; and (11) Engineering.
We used d values and lower– and upper–95 percent CIs to estimate standard error (SE) and Z-scores for each dimension assuming normal distributions: SE = (95% CI – 5% CI)/3.92, and Z = d/SE.
Z-score values were then converted to p-values using the standard normal distribution (Fisher et al. 1990).
The p-values for a set of test statistics were displayed in a p-value plot. The plot is used to visually check the characteristics of test statistics that address the same research question. The plot, originally presented by Schweder and Spjøtvoll, is professionally well-regarded and has been cited more than five hundred times in scientific literature.222
We construct and interpret p-value plots as follows:
- We compute and order p-values from smallest to largest and plot them against the integers 1, 2, 3, …
- If the points on the plot follow an approximate 45-degree line, we conclude that the p-values resulted from a random (chance) process and that the data therefore supported the null hypothesis of no significant association.
- If the points on the plot approximately follow a line with a flat/shallow slope, where most of the p-values were small (less than 0.05), then the p-values provide evidence for a real (statistically significant) association.
- If the points on the plot exhibit a bilinear shape (divided into two lines), then the p-values used for meta-analysis are consistent with a two-component mixture, and a general (overall) claim is not supported; in addition, the p-value reported for the overall claim in the meta-analysis paper cannot be taken as valid.
In short, every 45-degree line or bilinear shape in the results below provides evidence that there are no significant associations between the tested variables.
Regarding sIAT p-value plots, another possibility is that, in the absence of methodological, reporting, and publication biases,223 deviations from a near−45⁰ line in the plot may indicate departures from the uniform distribution and that there could be a real, non-random association between tested variables. We consider this possibility remote, as the methodological limitations (and biases) of the IAT are well-published.224
We also used a volcano plot to visually examine p-values from the Su et al. meta-analysis dataset. This is a type of scatterplot used to check for patterns in data, particularly for identifying statistically significant differences between two populations.225
Results
sIAT
We examined three correlations in the Kurdi et al. and Kurdi and Banaji226 meta-analysis sex dataset: (1) implicit-criterion correlations (ICCs), (2) explicit-criterion correlations (ECCs), and> (3) implicit-explicit correlations (IECs). Figure 5 displays Z-statistic frequency histograms (left side), box-and-whisker plots (right side), and quantiles (bottom) for each of the three correlations. Medians for each of the three comparisons are remarkably close to zero, which would make sense if the IAT is invalid measure.
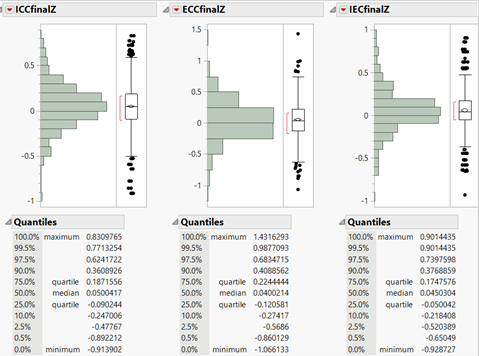
We present rank-ordered p-values computed for 27 ICC, ECC, and IEC correlations in Figures 6–8, respectively, for 27 studies from Kurdi et al. and Kurdi and Banaji meta-analysis dealing with sex. The p-value trends displayed irregular (unexpected) shapes in each of the plots. Averaging r values in each individual study reduces the measurement error, which may explain the resulting irregular trend of p-values in these plots. In any case, p-values in the plots are all greater than 0.05 and do not support real associations between the tested variables.
We would like to emphasize how unusual it is for a meta-analysis of an established body of research to discover that none of the p-values in the individual base studies are less than 0.05—that none of the individual studies meet even the weak p<0.05 standard of statistical significance.
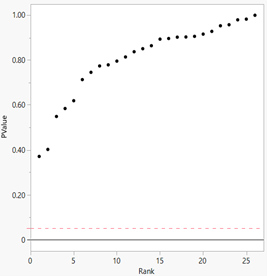
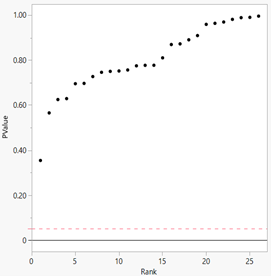
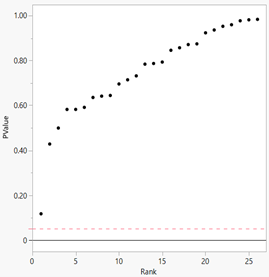
Vocational Interests
We then computed rank-ordered p-values for the 11 vocational interest dimensions reported by Su et al.; these are shown in Figure 9a.227 The plot shows that most of the observed data points are less than 0.05. The plot, in effect, supports real, non-random sex (female−male) differences in vocational interests. Our volcano plot (Figure 9b) also shows Social, Artistic, Conventional, and Data-Ideas vocational interest dimensions favoring women, especially the Social and Artistic dimensions. The strongest vocational interest dimensions favoring men are Realistic, Things-People, and Engineering.
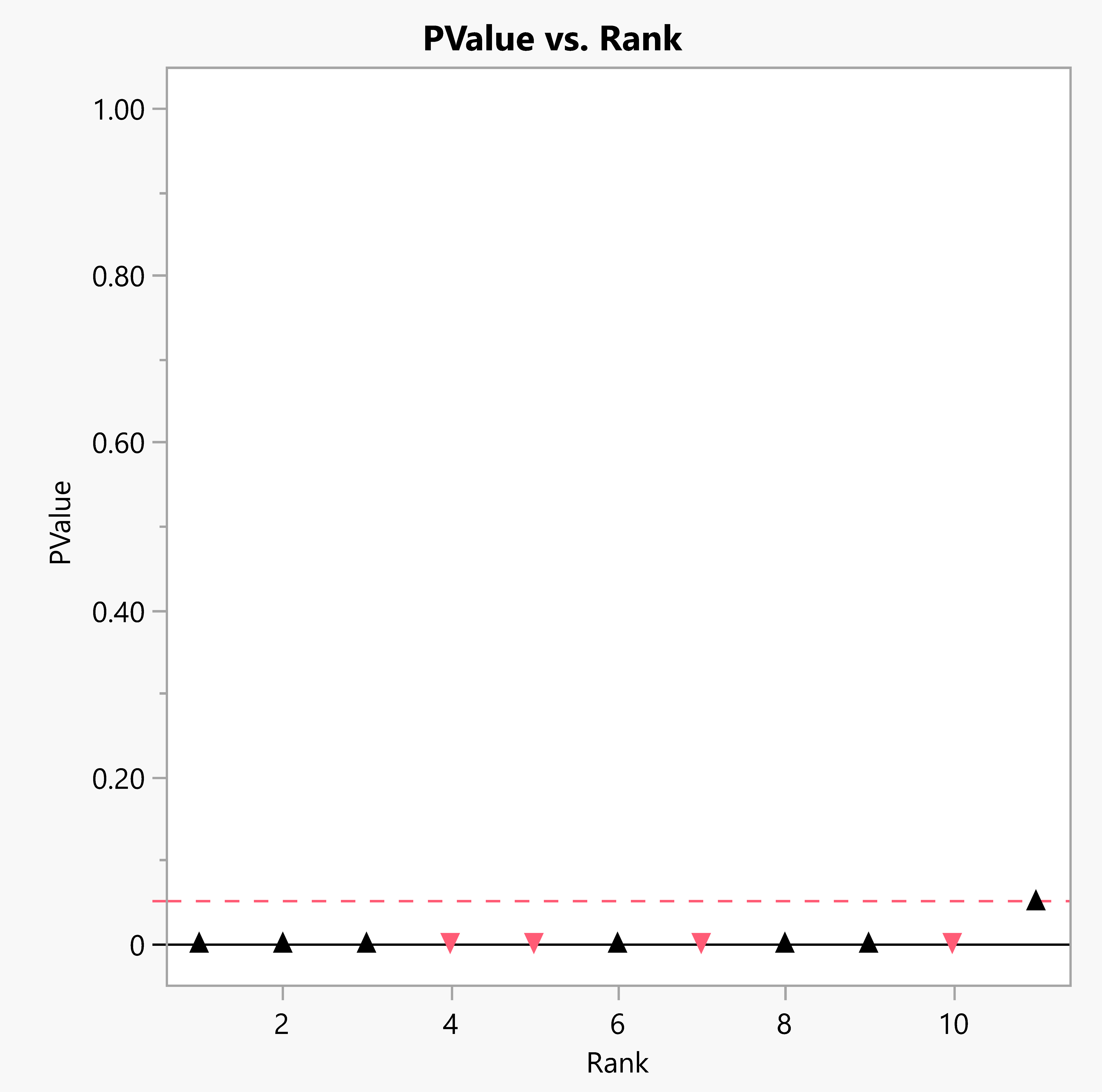
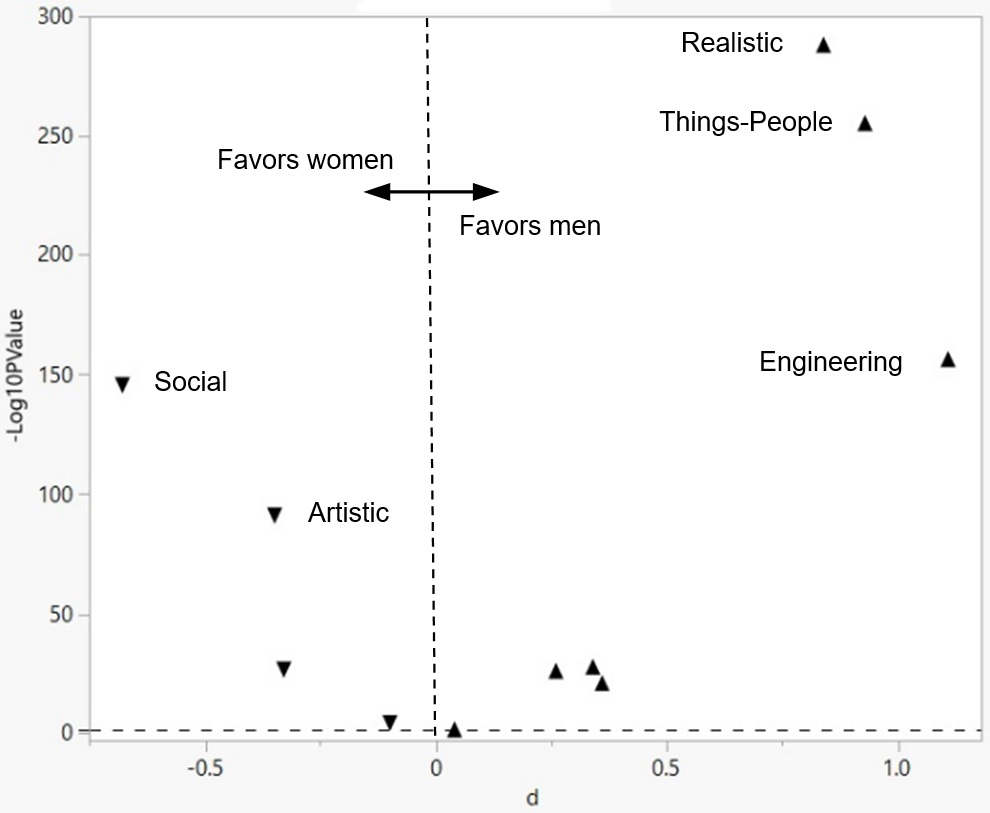
We would like to emphasize that our methodology registered a true result in the horizontal line in Figure 9a, which consists almost entirely of p-values less than 0.05. This is in strong contrast to the 45-degree lines in Figures 6–8, where none of the p-values in the individual base studies were less than 0.05.
Discussion
We considered three relevant measures to assess the validity of an sIAT measure: (1) the sIAT (implicit measures); (2) explicit measures of bias—such as indications of attitude, belief, or preference of sex bias—captured in a questionnaire or a similar research instrument; and (3) observations/measurements of real-world sex biased behaviors or actions (criterion measures). We considered these instruments in relation to their ability to explain sex differences in high-ability careers.
The p-value plots (Figures 6 –8) explored implicit-criterion correlations (ICCs), explicit-criterion correlations (ECCs), and implicit-explicit correlations (IECs). The data points (p-values) in these plots were all greater than 0.05 and do not support real associations between the tested variables.
Implicit bias, as measured by the Implicit Association Test (IAT), is one of psychology’s biggest ideas in the last thirty years. A Google Scholar search of the phrase “Implicit Association Test” on 2 May 2024 returned 49,500 articles and/or citations. Even so, there is now strong criticism of the IAT. Two key criticisms predominate—validity and reliability. Regarding validity, research has shown that the IAT has low correlation with explicit measures and real-world actions.228 This research implies that the IAT does not measure what it is said to measure.
Reliability is also in question. Repeated measures of the IAT show that much of the “measurement” is measurement error; the value for an individual fluctuates considerably around a mean value, so much so that, in the case of race, it is not useful for predicting discrimination.229 The p-value plots (Figures 6–8) support these IAT criticisms. The applicability of the sIAT for describing sex differences in high-ability careers is questionable.
We previously stated that there is evidence that the IAT, in general, and the sIAT, in particular, poorly correlate with explicit measures and explain very little of the variance of sex differences.230 This raises the question of what is missing in studies of sex differences in high-ability careers. As noted previously, Su et al. observed real sex differences in STEM interests in a meta-analysis of vocational interests.231 The p-value and volcano plots (Figures 9a and 9b) show strong female−male differences for several vocational interest dimensions (Social and Artistic dimensions favoring women; and Realistic, Things-People, and Engineering dimensions favoring men).
Given these strong female−male differences, there is merit in examining aspects of bias in the sIAT—specifically, that of residual bias from possible missing confounding factors. Good examples are female−male differences in vocational interests. This bias is referred to as omitted variable bias in the professional literature.232 A complete discussion of all possible factors in relation to STEM and medical academic careers is not something that can be addressed here. However, we use examples for several factors to show the importance of bias from omitted variables.
Confounders
Initially, we use simple linear regression models between two variables to examine theoretical aspects of confounding from omitted variables. We then follow up with several sex studies of professors in academic medicine that show the importance of confounding from omitted variables. (See theoretical discussion of linear regression models in the footnote.)233 If there are important unknown confounders omitted in modeling, then a modeling exercise exploring sex differences can be biased (unreliable). We consider below two sex studies of professors in medical schools—Jena et al. and Carr et al.234
Jena (2015): Jena et al. examined the proportion of females at the rank of full professor in US medical schools over the period 1980−2014. Their sample size was very large—91,073 US academic physicians. They considered physician sex (independent or predictor variable) and several confounding factors in their model—age, years since residency, specialty, authored publications (a measure of research productivity), National Institutes of Health (NIH) funding, and clinical trial participation. They noted that the percentage of full professors was 28.6 (males) and 11.9 (females) before adjusting for these variables—resulting in a gap of 16.6 percentage points. After adjusting for the multiple omitted variables noted above, the gap shrank to only 3.8 percentage points.
Carr (2018): Carr et al. tracked 1,273 faculty at 24 medical schools in the US for 17 years to identify predictors of advancement, retention, and leadership for female faculty as part of the National Faculty Survey. This was a national cohort of faculty followed from 1995 to 2012–2013 to examine differences in career outcomes by sex. Carr et al. looked at physician sex (independent variable) and numerous confounders in their model. These included: race; medical specialization; seniority level; percent effort distribution for administrative, research, clinical, and teaching activities; marital status; parental status; and academic productivity as measured by the total number of refereed publications.
After adjusting for all confounders except refereed career publications, females were less likely than males to achieve the rank of professor (odds ratio, OR = 0.57; 95% confidence interval, CI, 0.43–0.78) or to remain in academic careers (OR = 0.68; 95% CI, 0.49–0.94). However, when total number of refereed publications was added to their model, Carr et al. observed that differences by sex in retention and attainment of senior rank were no longer significant.
The findings of Jena et al. and Carr et al. show the importance of confounders in studies of sex differences in academic medicine, including the sIAT. Any study could quite easily show large sex differences in US academic physician positions by omitting many of the confounders used by Jena et al. and Carr et al.
Broad Confounders
When we consider the professional career choices of females and males, it is relatively easy to tabulate the numbers of females and males in a given profession. Consider females in STEM academic faculty positions. As of 2019, females/males made up 34.5/65.5% of STEM faculty and 28.2/71.8% of tenured STEM faculty at academic institutions in the US.235 Some researchers have attributed sex differences in STEM and medical professorship careers to implicit bias.236 However, we and other researchers have provided substantial evidence that the insufficient applicability and reproducibility of the sIAT (Figures 6–8) argues that implicit bias provides a poor explanation for sex differences in these careers.
Haier noted that there are no simple answers to sex differences in professional careers and that there are persuasive data that suggest multiple interacting cultural, social, and biological factors are at play.237 Researchers argue that several of these factors contribute meaningfully to sex differences in professional careers, including female−male differences in:
- math, verbal, and social skills;
- interests, career, and lifestyle preferences; and
- cognitive (thinking) ability.238
Researchers have presented data that some of these factors weigh in favor of females, while others weigh in favor of males. These factors are widely known among psychologists, which renders it puzzling that proponents of implicit bias theory have neither acknowledged their existence nor used them as confounders to see if implicit bias (i.e., sIAT) measures add any predictive power to these alternate factors in explaining sex differences in the STEM field and academic medicine.
For illustrative purposes, we further explore two possible vocational interest dimension confounders that weigh in favor of males—Things-People and Engineering interests (Figure 9b). We ignored the Realistic dimension, as Su et al. noted that it accounted for most of the Things-People sex difference.239 Our interest here is to try to understand how these interests may confound an implicit bias claim based on the sIAT.
Things-People vocational interests: Females and males are free to choose their professional careers as well as how they want to make their way in the world based on their interests. People’s interests are predictive of their behaviors in particular environments, such as their choice of college major and career occupation.240 Figure 9b shows a very strong effect for sex differences in working with things or people; there is a stronger effect of males favoring working with things over people.
We illustrate a hypothetical example with Things-People data from Su et al. (Figure 10). Su et al. reported a mean effect size (d) for the Things-People dimension of 0.93 favoring men in their study.241 This is comparable to a Things-People d = 1.01 favoring men reported by Morris in a more recent study of vocational interests of US residents.242 The distribution for females is shifted slightly to the left in the figure. A shift to the right of zero indicates greater interest for things over people, whereas a shift to the left of zero indicates greater interest for people over things.
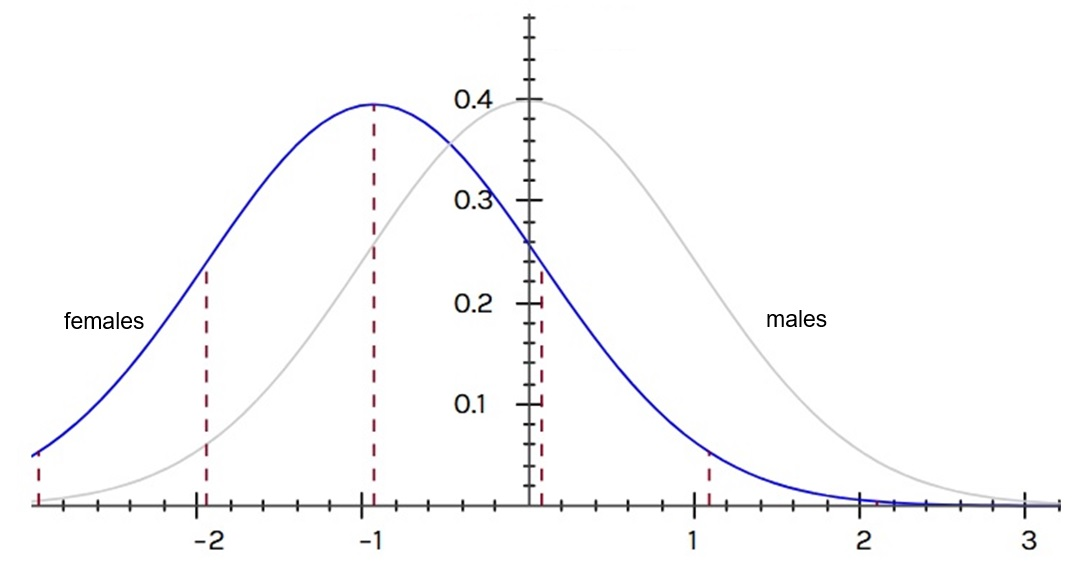
Now, 50 percent of males are to the right of zero, whereas only 17.6 percent of females are to the right, with a preference for working with things (refer to Table 5). Moving further to the right of zero on the horizontal axis in Figure 10 makes a huge difference in the numbers of females with a preference for working with things. The ratio of males to females at one (two) standard deviation to the right is 5.9 (13) (Table 5).
These differences are noteworthy given that STEM fields are typically things-oriented.244 As an example, if a person needs to be one to two standard deviations to the right of zero in the Things-People distribution to select a STEM vocation, then there will be 5.9 to 13 times as many males as females in this pool.
Standard deviation (SD) relative to male |
Area under male curve above SD (AUCm) |
Area under female curve above SD (AUCf) |
AUCm/AUCf ratio |
---|---|---|---|
0 |
0.5000 |
0.1762 |
2.8 |
1 |
0.1587 |
0.0268 |
5.9 |
2 |
0.0228 |
0.0017 |
13 |
3 |
0.0014 |
0.0004 |
32 |
Engineering Vocational Interests: Students graduating high school are more likely to enroll in engineering programs if their standardized Scholastic Assessment Test (SAT) math scores are favorable.245 Further, math proficiency skills (SAT math scores) have been shown to be a strong positive predictor of attending an engineering graduate program;246 a graduate PhD degree is a necessary requirement for any type of tenured postsecondary academic position in this field.
Math proficiency skills and cognitive ability overlap.247 These skills are also known to be highly general capabilities for processing complex information of any type and for successful performance in high-complexity careers.248 These attributes can influence job performance, particularly higher up occupational hierarchies in various fields, including STEM and academic medicine.
Math proficiency skills based on scores in numeracy tests (e.g., SAT math scores) have more consistent positive effects on job content skills and wages than scores on non-math literacy tests.249 Female−male performance in the standardized SAT for math over a 44-year period in United States is shown in Figure 11.
Females consistently underperformed males by 30 or more points over the period represented in Figure 11. Further, 2016 SAT math score data (mean (µ) = 494, σ = 116, n = 875,342 (females); µ = 524, σ = 126, n = 762,247 (males)) translates into an effect size (d) disadvantage of −0.248 (σ = 0.92) for females.250
How might a general female disadvantage in math proficiency skills play out in high-ability careers? A hypothetical example is illustrated with 2016 SAT math score data (Figure 12). We again use males as the reference point, i.e., the distribution for males has µ = 0 and σ = 1, while the distribution for females has µ = −0.248 (shown as −0.25) and σ = 0.92 in Figure 12. The distribution for females is shifted slightly to the left in the figure. A shift to the right of zero indicates higher SAT math scores, whereas a shift to the left of zero indicates lower scores.
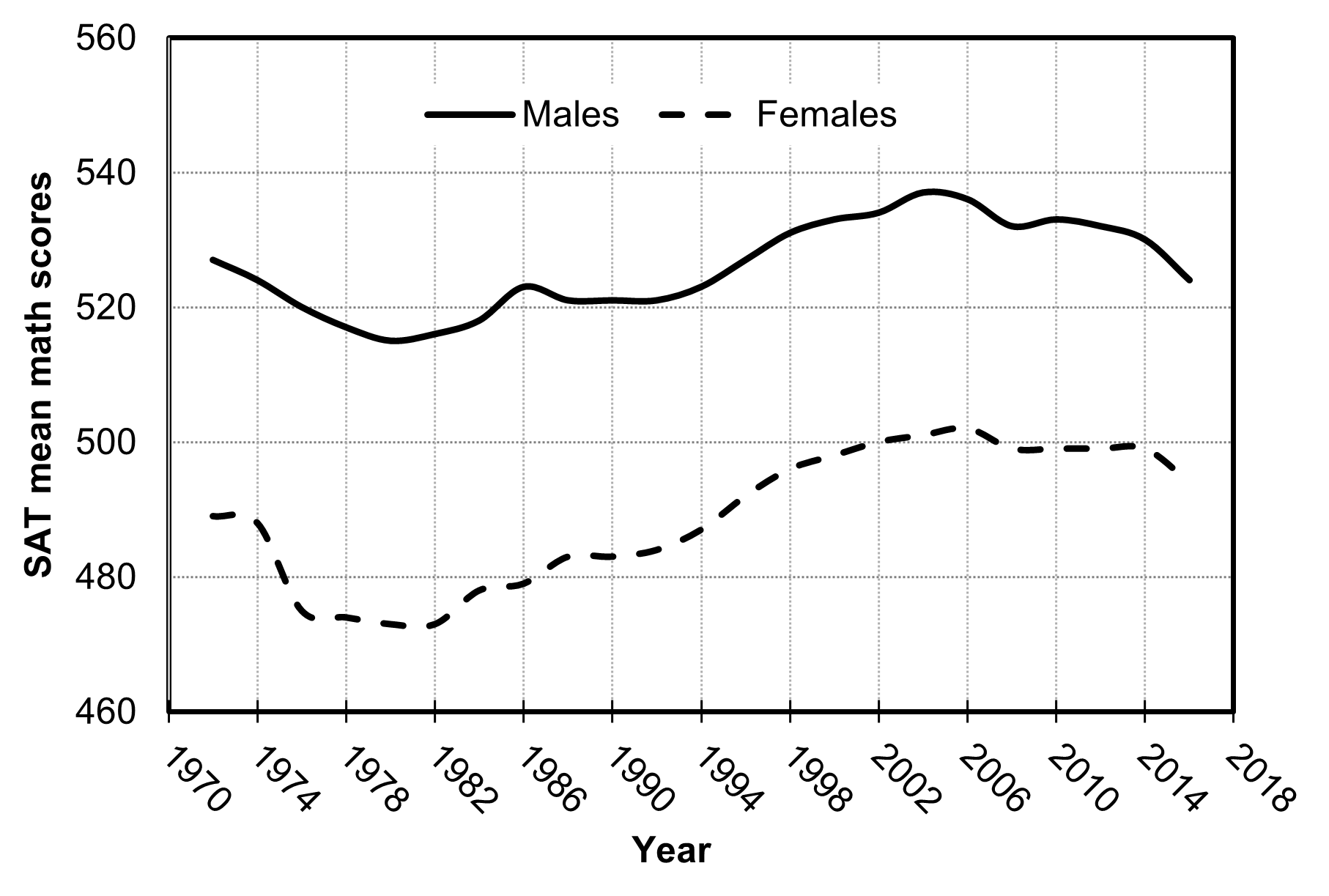
Differences further above the average (i.e., above µ = 0) may be most relevant for assessing how females and males compete for and occupy high-ability careers. The net effect is that the ratio (and numbers) of males to females increases with increasing SAT math scores—there are fewer numbers of females than males at higher levels of SAT math scores.
For the area under the curves in Figure 12—which represent SAT math scores greater than one, two, and three standard deviations to the right of zero on the horizontal axis—there are>, respectively, 1.8, 3, and 7 times as many males as females (Table 6). At the right side of Figure 12, there are fewer females than there are males.
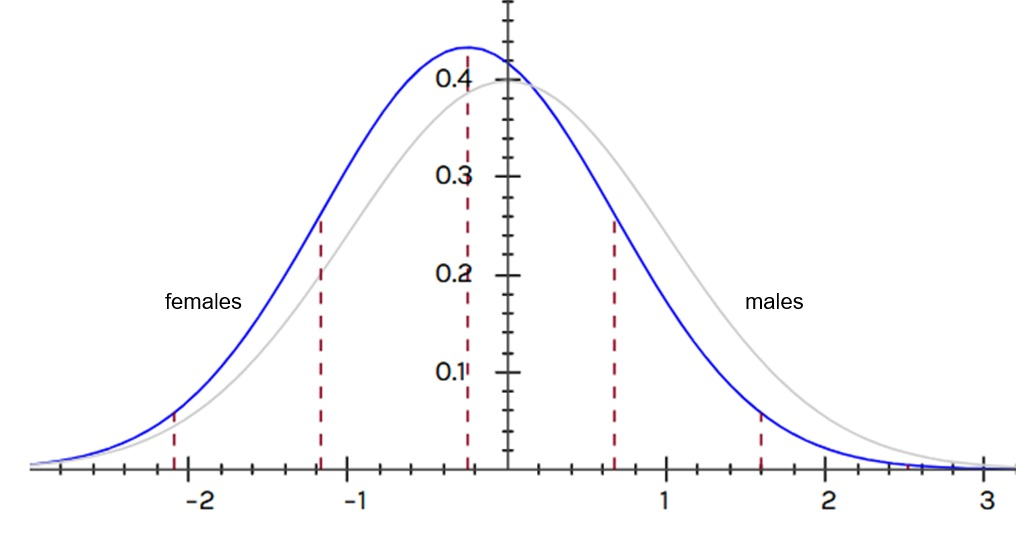
Standard deviation (SD) relative to male |
Area under male curve above SD (AUCm) |
Area under female curve above SD (AUCf) |
AUCm/AUCf ratio |
---|---|---|---|
0 |
0.5000 |
0.3930 |
1.3 |
1 |
0.1587 |
0.0875 |
1.8 |
2 |
0.0228 |
0.0075 |
3.0 |
3 |
0.0014 |
0.0002 |
7.0 |
These two hypothetical examples illustrate that there are fewer numbers of females with Things-People interests and that math proficiency skills may offer better explanations than implicit bias for sex differences in the makeup of academic STEM or medicine faculties. Factors like this and others that weigh in favor of males argue that it is predictable that there will be more males than females in high-ability careers.
There may be good counterarguments regarding the weight to be ascribed to these alternate explanatory factors. Yet the proponents of implicit bias theory do not make these counterarguments, because virtually no sIAT studies discuss or consider other factors that could explain fewer females in these fields. We illustrated two possible factors that favor males, interests in working with things and math proficiency skills. There could be other important factors. What matters is that the proponents of implicit bias theory and measurements scarcely consider confounding factors at all. Since they do not, we should not be surprised that implicit bias (sIAT) measures have little or no explanatory power for describing sex differences in high-ability careers.
Conclusions
Males outnumber females in many high-ability careers, including the fields of science, technology, engineering, and mathematics (STEM) and medical academia. Researchers often attribute these differences to implicit (subconscious) bias.
We used statistical p-value plots to independently test the ability to reproduce a claim of implicit bias made in the Kurdi et al. and Kurdi and Banaji meta-analyses of sex bias studies.253 The meta-analysis examined correlations between implicit bias measures based on the sex Implicit Association Test (sIAT) and measures of intergroup (female and male) behavior.
The p-value plots constructed using datasets from the meta-analysis (Figures 6–8) did not support real associations between the tested variables. These plots did not reproduce the research claim of implicit bias made in the Kurdi et al. and Kurdi and Banaji meta-analyses. These findings reinforce the lack of correlation between sIAT (implicit bias) measures and real-world sex behaviors in high-ability careers.
We used a p-value plot to independently test the ability to reproduce sex (female−male) differences in vocational interests reported in a meta-analysis of vocational interests by Su et al.254 The p-value plot for the meta-analysis (Figure 9a), in effect, supported real, non-random sex (female−male) differences in vocational interests.
Implicit bias measures have little or no explanatory power for sex differences in high-ability careers. There is little need to appeal to implicit bias (sIAT) measures to explain fewer females in these positions.
Recommendations
What Is at Stake
The stakes of the implicit bias revolution are extraordinary—and stretch far beyond the realm of academic psychology. In a host of professional venues, and throughout American society, Americans have assented to a new demonology—a Malleus Maleficarum pretending to find evidence of the witchcraft of “implicit bias” and, further, discerning from the discovery of the telltale marks of witchery a host of incidents of the evil eye, prejudicial behavior that the coven of witches surely exhibited, because it had been proved that they were witches. Implicit bias theory deserves no more credit than the Malleus, but credulous Americans by the millions have treated our woke witch doctors with PhDs as guardians of the truth, because such shamans have claimed the authority of science.
Radical activists, through some mixture of Machiavellianism and self-deception, have seized upon implicit bias theory to push through a host of requirements—in fields including medicine, policing, and law—for implicit bias training, diversity training, and a host of other forms of ideological indoctrination in the authoritarian precepts of “diversity, equity, and inclusion.” These activists would doubtless push for “diversity trainings” even without the formal support of implicit bias theory. But implicit bias theory and the IAT have helped justify these illiberal policies and may claim decisive credit—and deserve decisive blame—for giving them the authority of science with policymakers and the public.
The implicit bias revolution is most dangerous in its infiltration of the legal arena. Implicit bias removes individual intent and action from the law and replaces it with a statistical study of putative discrimination. The law is no longer a means to ascertain individual innocence and guilt but a means for the administrative state to impose “equity,” regardless of individual merit or justice. Its means, moreover, are arbitrarily assigned statements of what “equity” should consist of, while the evidence for implicit bias—for discrimination of any sort—is statistical. Every aspect of the irreproducibility crisis—groupthink, false positives, small samples—thereby becomes exported from the world of scientific and social scientific research to the world of law and justice. Radical activists now seek to use false positives to establish policy goals by means of judicial fiat, jury tampering, and subordinating the very ideal of individual justice to the ideology of identity-group equity.
Irreproducible false positives have become not only a research flaw but also the justification for and essential means by which radical activists can suborn the state to act at their behest, without restraint, in every sphere of society. Implicit bias is the means by which our courts replace law and justice with the false positives and groupthink that distort the radical bureaucrats’ understanding of the scientific method.
Policy Recommendations
Policymakers at every level have introduced laws and regulations based on implicit bias theory—federal bureaucrats, governors, state lawmakers, city officials, executives of professional associations, and more. These political initiatives ultimately need not seek a scientific justification for their reliance on implicit bias theory. But once they lose the patina of science, they simply become the assertion that bigotry is so ingrained in mankind that the people cannot be entrusted with the freedom to make any decisions in life and that the law cannot and should not account for individual intent and volition. Stated baldly, this ideology reveals itself as tyrannous contempt for mankind.
The citizens of a free republic should not allow such policies to rule them. Since implicit bias theory and its works have been revealed to be hollow pseudoscience, policymakers should work at once to remove the infringements of liberty undertaken in its name.
We cannot direct our recommendations to one federal agency, as we did in previous reports with our recommendations to the Environmental Protection Agency, the Food and Drug Administration, and the Centers for Disease Control and Prevention. Our recommendations here, necessarily, are abstract principles meant to guide policymakers and the public in every venue. These recommendations focus on the realm of the law and the judiciary, but they do not do so exclusively.
We make four general recommendations:
1. Rescind all laws, regulations, and programs based on implicit bias theory. Implicit bias theory and the IAT have been debunked as thoroughly as any scientific theory can be. No Americans should be subject to policy based on nonsense—much less policy intended to promote the radical ideology of identity politics. Policymakers should give priority to rescinding regulations that affect the personnel involved in executing law and order, such as judges, lawyers, and policemen, as well as medical personnel. Private institutions and enterprises should be encouraged by public opinion to rescind all activities, such as diversity trainings, based on implicit bias theory.
2. Establish a federal commission to determine what grounds should be used to cite social scientific research in federal regulation. Federal agencies such as the NIH or the EPA have procedures for requiring that regulations be founded on substantial scientific research—the procedures may not yet properly account for the irreproducibility crisis, but such procedures do exist. Generally, no equivalent procedures exist to guide, for example, the invocation of implicit bias by the U.S. Department of Education’s Guiding Principles: A Resource Guide for Improving School Climate and Discipline (2014).255 Social sciences such as psychology are not understood to be as rigorous as physics or chemistry, and a resource guide does not have the immediate effect of an EPA regulation. Nevertheless, some procedures need to be applied to all publications by the federal government, including both regulations and resource guides, to determine whether research that invokes concepts such as implicit bias has sufficient scientific justification. A commission should determine general guidelines for the use of social scientific research in such publications, with due weight given to transparent data, preregistration, proper statistical controls, publication bias, politicized groupthink, and all the aspects of the irreproducibility crisis. Each individual department and agency should then be mandated to apply the commission’s guidelines to their own procedures and publications.
3. Establish federal and state legislative committees to oversee social scientific support for proposed laws and regulations. Federal and state legislatures should have dedicated committees to investigate and provide judgment on all bills and new laws that justify their policies with social scientific research. Permanent committees, with permanent staff, will be able to provide informed judgment on all such bills and new laws. These committees should have the power to inform their fellow policymakers and the public about the social scientific support, or lack thereof, for new bills and new laws. Other committees should have the option to send a new bill to these social science committees for their judgment but should not be required to do so.
4. Legal and judicial education. Law schools, continuing legal education, and continuing judicial education should provide courses for lawyers and judges on the irreproducibility crisis, social scientific research, and best legal and judicial practices for assessing social scientific research and the testimony of expert witnesses. Federal and state policymakers should consider whether such courses ought to be mandatory.
We also suggest a principle that should govern policy concerning the judicial system, legal education, and the theoretical presumption of the operation of the law.
Individual behavior and events, and the first principles of due process, the presumption of innocence, and individual responsibility, should govern the operations of the law and determine the course of justice; no argument or policy based on statistical disparities should have any role in the operations of the law.
This principle should apply at least to:
- The education of judges, jurors, policemen, court personnel, and any other state employee involved in executing law and order;
- The training, work requirements, and promotion requirements for judges, jurors, policemen, court personnel, and any other state employee involved in executing law and order;
- Police enforcement of the law;
- Jury selection;
- Jury verdicts; and
- Judicial decisions.
We have not yet formulated these principles at the level of model legislation—nor, given the variety of assaults on the legal system, do we think that recommendations at that level of detail are appropriate. At present, we believe that policymakers and the public can enact substantial reform if they follow the principle that puts individual responsibility and the rule of the law above the activist false positives of statistical analysis. Even more importantly, following these principles will preserve America’s palladium of liberty, the rule of the law.
Conclusion
This is the fourth and last of our Shifting Sands reports. Each of them has provided a case study on how flawed science has underwritten costly policies that undermine liberty. This affliction has affected the Environmental Protection Agency, the Food and Drug Administration, the Centers for Disease Control and Prevention, and, through implicit bias theory and the IAT, a host of federal, state, and local governments, as well as private institutions and enterprises.
Our Shifting Sands reports do not merely show that a few federal agencies have come to misguided decisions based upon flawed science. Our reports reveal a deeper corruption of our republic, where the irreproducibility crisis of modern science, based above all upon the flawed use of statistics and groupthink, has facilitated the rise of an ideological, irresponsible, and incompetent elite, which endangers not only the practice of science but also all of our liberty and our law.
We have argued in each of our Shifting Sands reports for a range of reforms to correct how government uses science and statistics. Yet the stakes are much greater than a detailed reform of EPA or FDA regulations. Indeed, what is at issue is not just the spurious methods of irreproducible science but the use of these spurious methods at the heart of the broader attempt to dismantle American liberty. We do not pretend that our suggested reforms will work by themselves to protect America’s law and liberty. But we believe that these reforms are a necessary part of that broader struggle.
We will provide a larger view of the problem, and a larger series of suggested solutions, in our forthcoming capstone report to our Shifting Sands project. In brief, we must eliminate arbitrary science to eliminate arbitrary government.
Our capstone report will provide concrete suggestions on how to achieve that goal.
Appendix 1: The IAT and Real-World Behavior
Robstad et al. recently examined weight bias among 159 intensive care unit (ICU) nurses treating obese patients using the three instruments: the IAT (A), an explicit bias questionnaire (B), and an anti-fat questionnaire of behavior intentions (C).256 The latter—an anti-fat questionnaire of behavior intentions—captured whether the ICU nurses followed acceptable treatment rules/algorithms using treatment vignettes.
Like previous IAT studies discussed here, Robstad et al. reported that, as a group, ICU nurses had a positive weight IAT score, suggesting a group bias against obese patients. However, the treatment vignettes showed that the nurses followed acceptable treatment guidance irrespective of the suggested weight bias IAT score. In their analysis Robstad et al. noted that, after explicit bias (conscious attitudes or beliefs) was considered, there was little or no predictive power of IAT scores in explaining the behavior intentions of the nurses in their treatment of patients.
Robstad et al. computed correlation coefficient (r) values between implicit and explicit measures, age, work experience as an ICU nurse, and an anti-fat questionnaire of behavioral intentions. ICU nurses completed two IATs (Attitude and Stereotype), and their r correlations with the anti-fat questionnaire of behavior intentions were 0.11 and 0.03, respectively. Based on r2, these would account for about 1% of the variance of their behavior intentions.
We extracted the 78 correlations reported by Robstad et al., converted them to p-values using Fisher’s Z-transformation,257 and constructed a p-value plot. This is shown in Figure 1. Although not shown here, there were many highly significant correlations (i.e., p-values << 0.05), yet the crucial correlations of the IATs (Attitude and Stereotype) with anti-fat behavior intentions were not among these. Specifically, the Attitude IAT−behavior intention and Stereotype IAT−behavior intention p-values were 0.172 and 0.739, respectively.
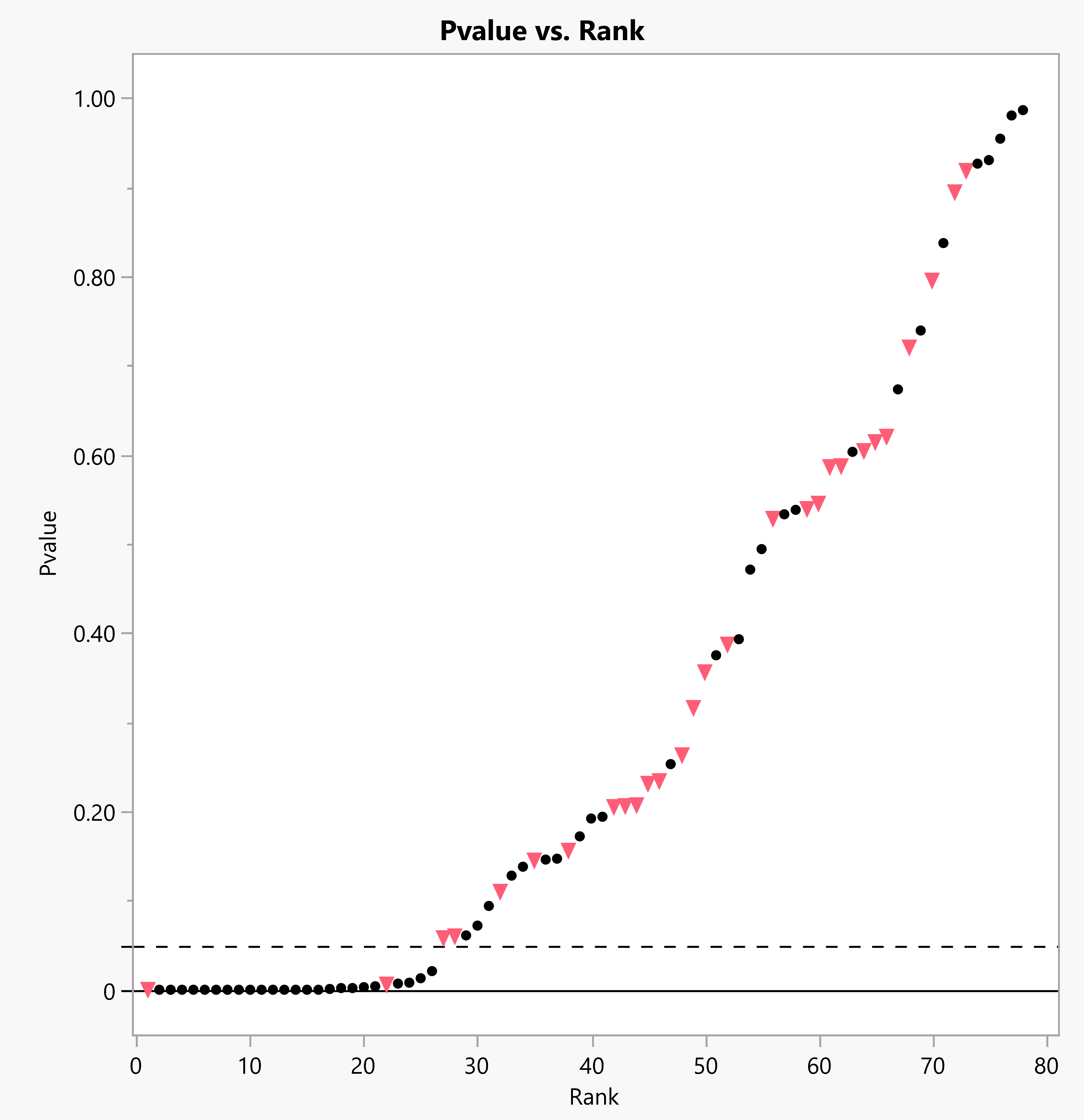
Finally, Freichel et al.258 employed several machine learning methods to assess the usefulness of implicit suicide cognitions, self-harm IATs, to predict a concurrent desire to self-harm or die with an online community sample of 6,855 participants. Specifically, they assessed whether self-harm IATs add to the prediction capability of (explicit) concurrent self-reported suicidality (thoughts or ideas about the possibility of ending one’s life) and desire to self-harm, over and above more easily collected measures—such as sociodemographic factors, self-reported history of self-harm and suicide, and explicit momentary self-harm and suicide cognitions.
Freichel et al. observed that, in their best-performing model, self-harm, suicide, and death IATs offered very little (<2%) to no predictive value on top of explicit measures that are much easier to collect to explain concurrent self-reported suicidality. Mood, explicit associations, and past suicidal thoughts and behaviors were the most important predictors of concurrent self-reported suicidality.
Implicit measures (self-harm, suicide, and death IATs) provided little to no gain in predictive accuracy. This study directly supports Schimmack’s position that implicit bias and explicit bias measures are essentially the same; once explicit measures are considered, nothing is gained by using implicit measures.
References
68 IAC (Illinois Administrative Code) 1130.500. N.d. Implicit Bias Awareness Training. https://www.ilga.gov/commission/jcar/admincode/068/068011300E05000R.html.
Aidman, E. V., & Carroll, S. M. 2003. Implicit individual differences: Relationships between implicit self-esteem, gender identity, and gender attitudes. European Journal of Personality 17, 1: 19–36. https://doi.org/10.1002/per.465.
Akbarzai, S. 2021. New Jersey is the latest state to require schools to offer courses on diversity and unconscious bias. CNN, April 11, 2021. https://www.cnn.com/2021/04/11/us/schools-new-jersey-new-law-unconscious-bias/index.html.
Aldrich, M. W. 2021. Tennessee governor signs bill restricting how race and bias can be taught in schools. The Tennessean, May 25, 2021. https://www.tennessean.com/story/news/education/2021/05/25/tennessee-critical-race-theory-governor-signs-bill-restricting-how-race-and-bias-can-taught-schools/7427131002/.
Aldrich, M. W. 2023. Bill would curb ‘implicit bias’ training in Tennessee schools, universities. Chalkbeat Tennessee, January 12, 2023. https://tn.chalkbeat.org/2023/1/12/23552718/implicit-bias-tennessee-school-employee-training-legislature.
Al-Marzouki, S., Evans, S., Marshall, T., & Roberts, I. 2005. Are these data real? Statistical methods for the detection of data fabrication in clinical trials. British Medical Journal 331: 267–70. https://doi.org/10.1136/bmj.331.7511.267.
Andreychik, M. R., & Gill, M. J. 2012. Do negative implicit associations indicate negative attitudes? Social explanations moderate whether ostensible “negative” associations are prejudice-based or empathy-based. Journal of Experimental Social Psychology 48, 5: 1082−93. https://doi.org/10.1016/j.jesp.2012.05.006.
Anselmi, P., Vianello, M., & Robusto, E. 2011. Positive associations primacy in the IAT: A many-facet Rasch measurement analysis. Experimental Psychology 58, 5: 376–84. https://doi.org/10.1027/1618-3169/a000106.
Arkes, H. R., & Tetlock, P. E. 2004. Attributions of implicit prejudice, or “Would Jesse Jackson ‘fail’ the Implicit Association Test?” Psychological Inquiry 15, 4: 257–78. https://doi.org/10.1207/s15327965pli1504_01.
Baker, M. 2016. 1,500 scientists lift the lid on reproducibility. Nature 533, 7604: 452–54. http://www.nature.com/news/1-500-scientists-lift-the-lid-on-reproducibility-1.19970.
Banaji, M. R., & Greenwald, A. G. 2013. Blindspot: Hidden Biases of Good People. New York, NY: Delacorte Press.
Bartels, J. M., & Schoenrade, P. 2021. The Implicit Association Test in introductory psychology textbooks: Blind spot for controversy. Psychology Learning & Teaching 21, 2: 113–25. https://doi.org/10.1177/14757257211055200.
Bass, A. C. 2021. The effects of explicit and implicit racial bias on evaluations of individuals involved with the criminal justice system. Dissertations, Theses, and Masters Projects. College of William & Mary. Paper 1627047840. http://dx.doi.org/10.21220/s2-werm-3q41.
Basu, D. 2024. How likely is it that omitted variable bias will overturn your results? Working Paper No. 2024-1. University of Massachusetts Amherst, Department of Economics. https://papers.ssrn.com/sol3/papers.cfm?abstract_id=4704246.
Begley, C. G., & Ellis, L. M. 2012. Raise standards for preclinical cancer research. Nature 483: 531−33. https://doi.org/10.1038/483531a.
Benjamin, D. J., Berger, J. O., Johannesson, M., Nosek, B. A., Wagenmakers, E.-J., Berk, R. ... Johnson, V. E. 2018. Redefine statistical significance. Nature Human Behaviour 2, 1: 6−10. https://doi.org/10.1038/s41562-017-0189-z.
Benjamini, Y., & Hochberg, Y. 1995. Controlling the false discovery rate: A practical and powerful approach to multiple testing. Journal of the Royal Statistical Society, Series B 57, 1: 289–300. https://doi.org/10.1111/j.2517-6161.1995.tb02031.x.
Bennett, M. W. 2010. Unraveling the Gordian knot of implicit bias in jury selection: The problems of judge-dominated voir dire, the failed promise of Batson, and proposed solutions. Harvard Law & Policy Review 4, 1: 149–71. https://harvardlpr.com/wp-content/uploads/sites/20/2013/05/4.1_8_Bennett.pdf.
Biden, J. R., Jr. 2021. Executive Order on Diversity, Equity, Inclusion, and Accessibility in the Federal Workforce. The White House. June 25, 2021. https://www.whitehouse.gov/briefing-room/presidential-actions/2021/06/25/executive-order-on-diversity-equity-inclusion-and-accessibility-in-the-federal-workforce/.
Bienias, E., Costales, S., Lynch, C., Pham, T. T., Rodman, R., & Rosenwald, L. 2017. Implicit Bias in the Legal Profession. Intellectual Property Owners Association. https://ipo.org/wp-content/uploads/2017/11/Implicit-Bias-White-Paper-2.pdf.
Blanton, H., & Jaccard, J. 2006. Arbitrary metrics in psychology. American Psychologist 61, 1: 27–41. https://doi.org/10.1037/0003-066X.61.1.27.
Blanton, H., & Jaccard, J. 2008. Unconscious racism: A concept in pursuit of a measure. Annual Review of Sociology 34: 277–97. https://doi.org/10.1146/annurev.soc.33.040406.131632.
Blanton, H., Jaccard, J., Klick, J., Mellers, B., Mitchell, G., & Tetlock, P. E. 2009. Strong claims and weak evidence: Reassessing the predictive validity of the IAT. Journal of Applied Psychology 94, 3: 567–82. https://doi.org/10.1037/a0014665.
Blanton, H., Jaccard, J., & Burrows, C. N. 2015a. Implications of the Implicit Association Test D-transformation for psychological assessment. Assessment 22, 4: 429–40. https://doi.org/10.1177/1073191114551382.
Blanton, H., Jaccard, J., Strauts, E., Mitchell, G., & Tetlock, P. E. 2015b. Toward a meaningful metric of implicit prejudice. Journal of Applied Psychology 100, 5: 1468–81. https://doi.org/10.1037/a0038379.
Blanton, H., & Jaccard, J. 2017. You can’t assess the forest if you can’t assess the trees: Psychometric challenges to measuring implicit bias in crowds. Psychological Inquiry 28, 4: 249–57. https://doi.org/10.1080/1047840X.2017.1373550.
Blanton, H., & Jaccard, J. 2023. Listening to measurement error: Lessons from the IAT. In J. A. Krosnick, T. H. Stark, & A. L. Scott, eds., The Cambridge Handbook of Implicit Bias and Racism. Cambridge: Cambridge University Press. https://osf.io/ar4u6.
Blevins, E. 2017. Can government regulate your subconscious? Pacific Legal Foundation, December 20, 2017. https://pacificlegal.org/can-government-regulate-your-subconscious/.
Bluemke, M., & Fiedler, K. 2009. Base rate effects on the IAT. Consciousness and Cognition 18, 4: 1029–38. https://doi.org/10.1016/j.concog.2009.07.010.
Bonessi, D. M. 2021. Here are all the new laws taking effect in Maryland on Friday. DCist, September 29, 2021. https://dcist.com/story/21/09/29/here-are-all-the-new-laws-taking-effect-in-maryland-on-friday/.
Boos, D. D., & Stefanski, L. A. 2013. Essential Statistical Inference: Theory and Methods. Springer Texts in Statistics 120. New York, NY: Springer. https://doi.org/10.1007/978-1-4614-4818-1.
Borchelt, J. W., & Smith, A. M. 2019. Employers need to be conscious of unconscious bias. Reminger, Employment Newsletter, August 2019. https://www.reminger.com/publication-829.
Bosak, J., & Kulich, C. 2023. Gender similarities hypothesis. In T. K. Shackelford, ed., Encyclopedia of Sexual Psychology and Behavior. Cham, Switzerland: Springer. https://doi.org/10.1007/978-3-031-08956-5_163-1.
Briggs, W. M. 2017. The substitute for p-values. Journal of the American Statistical Association 112: 897–98. https://doi.org/10.1080/01621459.2017.1311264.
Briggs, W. M. 2019. Everything wrong with p-values under one roof. In V. Kreinovich, N. N. Thach, N. D. Trung, & D. Van Thanh, eds., Beyond Traditional Probabilistic Methods in Economics. Studies in Computational Intelligence 809. Cham, Switzerland: Springer. https://doi.org/10.1007/978-3-030-04200-4_2.
Van den Brink, M. 2011. Scouting for talent: Appointment practices of women professors in academic medicine. Social Science & Medicine 72, 12: 2033–40. https://doi.org/10.1016/j.socscimed.2011.04.016.
BRM (Board of Registration in Medicine). 2021. The Massachusetts Board of Registration Adopts Policy Requiring CME on the Topic of Implicit Bias in Healthcare. Commonwealth of Massachusetts, November 18, 2021. https://www.mass.gov/news/the-massachusetts-board-of-registration-adopts-policy-requiring-cme-on-the-topic-of-implicit-bias-in-healthcare.
Buchanan, J. M., & Tullock, G. 2004. The Calculus of Consent: Logical Foundations of Constitutional Democracy. Indianapolis: Liberty Fund, Inc. http://files.libertyfund.org/files/1063/Buchanan_0102-03_EBk_v6.0.pdf.
Cabinet Office. 2020. Written Ministerial Statement on Unconscious Bias Training. Gov.uk, December 17, 2020. https://www.gov.uk/government/news/written-ministerial-statement-on-unconscious-bias-training.
Carlsson, R., & Agerström, J. 2016. A closer look at the discrimination outcomes in the IAT literature. Scandinavian Journal of Psychology 57, 4: 278–87. https://doi.org/10.1111/sjop.12288.
Carr, P. L., Raj, A., Kaplan, S. E., Terrin, N., Breeze, J. L., & Freund, K. M. 2018. Gender differences in academic medicine: Retention, rank, and leadership comparisons from the National Faculty Survey. Academic Medicine 93, 11: 1694–99. https://doi.org/10.1097/ACM.0000000000002146.
CDCP (Centers for Disease Control and Prevention). N.d. What Is Health Equity? https://web.archive.org/web/20240710083747/https://www.cdc.gov/healthequity/whatis/index.html.
Cesario, J. 2022. So close, yet so far: Stopping short of killing implicit bias. Psychological Inquiry 33, 3: 162−66. https://doi.org/10.1080/1047840X.2022.2106753.
CGC (California Government Code) § 68088. N.d. Section 68088 - Racial, ethnic and gender bias and sexual harassment training; training on implicit bias. https://casetext.com/statute/california-codes/california-government-code/title-8-the-organization-and-government-of-courts/chapter-1-general-provisions/section-68088-racial-ethnic-and-gender-bias-and-sexual-harassment-training-training-on-implicit-bias.
CGA (Colorado General Assembly) SB22-128. 2022. Implicit Bias in Jury Selection. https://leg.colorado.gov/bills/sb22-128.
Chambers, C. 2017. The Seven Deadly Sins of Psychology: A Manifesto for Reforming the Culture of Scientific Practice. Princeton, NJ: Princeton University Press.
Chapter 2022-72 (2022). State of Florida. http://laws.flrules.org/2022/72.
Chequer, S., & Quinn, M. G. 2021. More error than attitude in Implicit Association Tests (IATs), a CFA-MTMM analysis of measurement error. PsyArXiv Preprints. https://psyarxiv.com/afyz2.
Chin, J., Holcombe, A., Zeiler, K., Forscher, P., & Guo, A. 2023. Metaresearch, psychology, and law: A case study on implicit bias. Boston University School of Law, Scholarly Commons at Boston University School of Law. https://scholarship.law.bu.edu/faculty_scholarship/3422/.
Chivers, C. 2022. Combatting implicit bias in the legal profession. The Justice and Diversity Center, The Bar Association of San Francisco. September 7, 2022. https://www.sfbar.org/blog/combatting-implicit-bias-in-the-legal-profession/.
CIA (Central Intelligence Agency). 2016. CIA Diversity and Inclusion Strategy (2016-2019). https://www.cia.gov/static/afbc609d09f79da0ba7a0d4eed27956d/Diversity-Inclusion-Strategy-2016-to-2019.pdf.
Clyde, M. 2000. Model uncertainty and health effect studies for particulate matter. Environmetrics 11, 6: 745–63. https://doi.org/10.1002/1099-095X(200011/12)11:6<745::AID-ENV431>3.0.CO;2-N.
College Board. 2016. 2016 College-Bound Seniors, Total Group Profile Report. https://reports.collegeboard.org/media/pdf/2016-total-group-sat-suite-assessments-annual-report.pdf.
College Board. 2024. SAT Suite Data and Reports Archive. New York, NY: College Board. https://reports.collegeboard.org/sat-suite-program-results/data-archive.
Columbus. N.d. Implicit Bias. Citywide Training Course Offerings. City of Columbus. https://web.archive.org/web/20230223201431/https://www.columbus.gov/hr/citywide-training/course-offerings/Implicit-Bias/.
Cone, J., Mann, T. C., & Ferguson, M. J. 2017. Chapter Three - Changing our implicit minds: How, when, and why implicit evaluations can be rapidly revised. Advances in Experimental Social Psychology 56: 131−99. https://doi.org/10.1016/bs.aesp.2017.03.001.
Corneille, O., & Mertens, G. 2020a. Behavioral and physiological evidence challenges the automatic acquisition of evaluations. Current Directions in Psychological Science 29, 6. https://doi.org/10.1177/0963721420964111.
Corneille, O., & Hütter, M. 2020b. Implicit? What do you mean? A comprehensive review of the delusive implicitness construct in attitude research. Personality and Social Psychology Review 24, 3: 212–32. https://doi.org/10.1177/1088868320911325.
Corneille, O., & Béna, J. 2022. The “implicit bias” wording is a relic. Let’s move on and study unconscious social categorization effects. Psychological Inquiry 33, 3: 167–72. https://doi.org/10.1080/1047840X.2022.2106754.
Couzin, J., & Unger, K. 2006. Cleaning up the paper trail. Science 312, 5770: 38–43. https://doi.org/10.1126/science.312.5770.38.
Coyle, T. R. 2018. Non-g factors predict educational and occupational criteria: More than g. Journal of Intelligence 6, 3: 43. https://doi.org/10.3390/jintelligence6030043.
CPC (California Penal Code) § 13519.4. N.d. https://leginfo.legislature.ca.gov/faces/codes_displaySection.xhtml?lawCode=PEN§ionNum=13519.4.
CSB (California Senate Bill) 263. 2021. State of California. https://leginfo.legislature.ca.gov/faces/billTextClient.xhtml?bill_id=202120220SB263.
Cummins, H. J. 2007. Two lawsuits promote growing awareness of ‘unconscious bias.’ South Coast Today, April 10, 2007. https://www.southcoasttoday.com/story/business/employment/2007/04/10/two-lawsuits-promote-growing-awareness/52927987007/.
Cyrus-Lai, W., Tierney, W., du Plessis, C., Nguyen, M., Schaerer, M., Clemente, E. G., & Uhlmann, E. L. 2022. Avoiding bias in the search for implicit bias. Psychological Inquiry 33, 3: 203–12. https://doi.org/10.1080/1047840X.2022.2106762.
Dang, J., King, K. M., & Inzlicht, M. 2020. Why are self-report and behavioral measures weakly correlated? Trends in Cognitive Sciences 24, 4: 267–69. https://doi.org/10.1016/j.tics.2020.01.007.
Danziger, K. 1990. Constructing the Subject: Historical Origins of Psychological Research. Cambridge Studies in the History of Psychology. Cambridge, UK: Cambridge University Press.
Dehon, E., Weiss, N., Jones, J., Faulconer, W., Hinton, E., & Sterling, S. 2017. A systematic review of the impact of physician implicit racial bias on clinical decision making. Academic Emergency Medicine 24, 8: 895–904. https://doi.org/10.1111/acem.13214.
van Dessel, P., Cummins, J., Hughes, S., Kasran, S., Cathelyn, F., & Moran, T. 2020. Reflecting on 25 years of research using implicit measures: Recommendations for their future use. Social Cognition 38, Suppl.: S223–S242. https://doi.org/10.1521/soco.2020.38.supp.s223.
Diener, E., & Biswas-Diener, R. 2018. The replication crisis in psychology. In R. Biswas-Diener & E. Diener, eds. Introduction to Psychology. Champaign, IL: DEF Publishers. https://nobaproject.com/modules/the-replication-crisis-in-psychology. In https://nobaproject.com/textbooks/introduction-to-psychology-the-full-noba-collection.
Donyéa, T. 2022. N.J. lawmakers approve bias training for police, assemblyman’s comments decried as ‘rooted in racist ideology.’ WHYY, February 28, 2022. https://whyy.org/articles/n-j-lawmakers-approve-bias-training-for-police-assemblymans-comments-decried-as-rooted-in-racist-ideology/.
EEOC (U.S. Equal Employment Opportunity Commission). 2008. Final Decree Entered with Walgreens for $24 Million in Landmark Race Discrimination Suit by EEOC. March 25, 2008. https://www.eeoc.gov/newsroom/final-decree-entered-walgreens-24-million-landmark-race-discrimination-suit-eeoc-0.
EEOC (U.S. Equal Employment Opportunity Commission). 2021. EEOC Launches Diversity, Equity, & Inclusion (DE&I) Workshop Series. August 4, 2021. https://www.eeoc.gov/newsroom/eeoc-launches-diversity-equity-inclusion-dei-workshop-series.
Egan, P. 2020. Whitmer to require implicit bias training for Michigan’s medical professionals. Detroit Free Press, July 9, 2020. https://www.freep.com/story/news/local/michigan/detroit/2020/07/09/whitmer-implicit-bias-training-healthcare-professionals/3287034001/.
Egger, M., Davey Smith, G., & Altman, D. G. 2001. Problems and limitations in conducting systematic reviews. In M. Egger, G. Davey Smith., & D. G. Altman, eds., Systematic Reviews in Health Care: Meta-Analysis in Context. 2nd ed. London: BMJ Books. https://doi.org/10.1002/9780470693926.
Elek, J. K., & Hannaford-Agor, P. 2013. First, do no harm: On addressing the problem of implicit bias in juror decision making. Court Review: The Journal of the American Judges Association 404: 190–98. https://core.ac.uk/download/pdf/215161343.pdf.
Elosiebo, Y. 2018. Implicit bias and equal protection: A paradigm shift. N.Y.U. Review of Law & Social Change 42: 451–94. https://socialchangenyu.com/wp-content/uploads/2018/07/Elosiebo_Digital-Proof_7.23.18.pdf.
Ethics Commission. N.d. Implicit Bias Training Requirement for City Commissioners and Department Heads. City and County of San Francisco. Updated, February 5, 2024. https://sfethics.org/commission/implicit-bias-training-requirement-for-city-commissioners-and-department-heads.
Farrell, L., & McHugh, L. 2017. Examining gender-STEM bias among STEM and non-STEM students using the Implicit Relational Assessment Procedure (IRAP). Journal of Contextual Behavioral Science 6, 1: 80–90. https://doi.org/10.1016/j.jcbs.2017.02.001.
FHP (FordHarrison Publications). 2020. Unconscious bias training still permissible under President Trump’s diversity training executive order. FordHarrison, October 13, 2020. https://www.fordharrison.com/unconscious-bias-training-still-permissible-under-president-trumps-diversity-training-executive-order.
Fiedler, K., Messner, C., & Bluemke, M. 2006. Unresolved problems with the “I”, the “A”, and the “T”: A logical and psychometric critique of the Implicit Association Test (IAT). European Review of Social Psychology 17, 1: 74–147. https://doi.org/10.1080/10463280600681248.
Fisher, R. A. 1921. On the ‘probable error’ of a coefficient of correlation deduced from a small sample. Metron 1: 3–32. https://digital.library.adelaide.edu.au/dspace/bitstream/2440/15169/1/14.pdf.
Fisher, R. A. 1925. Statistical Methods for Research Workers. 13th ed. London: Oliver and Boyd. 99–101.
Fisher, R. A. 1990. Statistical Methods, Experimental Design, and Scientific Inference. New York, NY: Oxford University Press.
Forscher, P. S., Lai, C. K., Axt, J. R., Ebersole, C. R., Herman, M., Devine, P. G., & Nosek, B. A. 2019. A meta-analysis of procedures to change implicit measures. Journal of Personality and Social Psychology 117, 3: 522–59. https://doi.org/10.1037/pspa0000160.
Franco, A., Malhotra, N., & Simonovits, G. 2014. Publication bias in the social sciences: Unlocking the file drawer. Science 345, 6203: 1502–5. https://doi.org/10.1126/science.1255484.
Freichel, R., Kahveci, S., & O’Shea, B. 2024. How do explicit, implicit, and sociodemographic measures relate to concurrent suicidal ideation? A comparative machine learning approach. Suicide and Life-Threatening Behavior 54, 1: 49–60. https://doi.org/10.1111/sltb.13017.
Gaines, K. 2021. CA requires implicit bias training for all new grad nurses. Nurse.org, September 28, 2021. https://nurse.org/articles/california-nursing-implicit-bias-training/.
Gawronski, B. 2019. Six lessons for a cogent science of implicit bias and its criticism. Perspectives on Psychological Science 14, 4. https://doi.org/10.1177/1745691619826015.
Gawronski, B., Ledgerwood, A., & Eastwick, P. W. 2022. Implicit bias ≠ bias on implicit measures. Pyschological Inquiry 33, 3: 139–55. https://doi.org/10.1080/1047840X.2022.2106750.
Gelman, A., & Loken, E. 2014. The statistical crisis in science. American Scientist 102, 6: 460–465. https://www.americanscientist.org/article/the-statistical-crisis-in-science.
Gerber, A. S., & Malhotra, N. 2008. Publication bias in empirical sociological research: Do arbitrary significance levels distort published results? Sociological Methods and Research 37, 1: 3–30. http://journals.sagepub.com/doi/abs/10.1177/0049124108318973.
Girod, S., Fassiotto, M., Grewal, D., Ku, M. C., Sriram, N., Nosek, B. A., & Valantine, H. 2016. Reducing implicit gender leadership bias in academic medicine with an educational intervention. Academic Medicine 91, 8: 1143–50. https://doi.org/10.1097/ACM.0000000000001099.
Google Scholar. (2024a). Google search of Schweder and Spjøtvoll (1982) paper “Plots of p-values to evaluate many tests simultaneously.” https://scholar.google.com/scholar?hl=en&as_sdt=0%2C5&q=%22Plots+of+p-values+to+evaluate+many+tests+simultaneously%22&btnG=.
Google Scholar (2024b). https://scholar.google.com/scholar?hl=en&as_sdt=0%2C33&q=“Implicit+Association+Test”&btnG=.
Gottfredson, L. S. 2002. Where and why g matters: Not a mystery. Human Performance 15, 1–2: 25–46. https://doi.org/10.1080/08959285.2002.9668082.
Gottfredson, L. S. 2003. g, jobs and life. In H. Nyborg, ed., The Scientific Study of General Intelligence: Tribute to Arthur R. Jensen. New York, NY: Elsevier Sciences. 293–342. https://gwern.net/doc/iq/ses/2003-gottfredson.pdf.
Greenwald, A. G., & Banaji, M. R. 1995. Implicit social cognition: Attitudes, self-esteem, and stereotypes. Psychological Review 102, 1: 4–27. https://doi.org/10.1037/0033-295x.102.1.4.
Greenwald, A. G., McGhee, D. E., & Schwartz, J. L. K. 1998. Measuring individual differences in implicit cognition: The Implicit Association Test. Journal of Personality and Social Psychology 74, 6: 1464–80. https://doi.org/10.1037//0022-3514.74.6.1464.
Greenwald, A. G., & Farnham, S. D. 2000. Using the Implicit Association Test to measure self- esteem and self-concept. Journal of Personality and Social Psychology 79, 6: 1022–38. https://doi.org/10.1037//0022-3514.79.6.1022.
Greenwald, A. G., & Krieger, L. H. 2006. Implicit bias: Scientific foundations. California Law Review 94, 4: 945–67. https://scholarspace.manoa.hawaii.edu/server/api/core/bitstreams/cccf922b-2a03-441a-940a-90960f3b442c/content.
Greenwald, A. G., Dasgupta, N., Dovidio, J. F., Kang, J., Moss-Racusin, C. A., & Teachman, B. A. 2022. Implicit-bias remedies: Treating discriminatory bias as a public-health problem. Psychological Science in the Public Interest 23, 1: 7–40. https://doi.org/10.1177/15291006211070781.
Grine, A., & Coward, E. 2017. Recognizing implicit bias within the Equal Protection framework. Trial Briefs, April 2017. 26–30. https://www.sog.unc.edu/sites/www.sog.unc.edu/files/course_materials/Ag%20and%20Coward%20Article.pdf.
GSP (Granite State Progress). N.d. HB 544: Banning Implicit Bias Training (Oppose). https://granitestateprogress.org/our-work/state-house-hearings-votes/hb-544-banning-implicit-bias-training-oppose/.
Hahn, A., Judd, C. M., Hirsh, H. K., & Blair, I. V. 2014. Awareness of implicit attitudes. Journal of Experimental Psychology: General 143, 3: 1369–92. https://doi.org/10.1037/a0035028.
Hahn, A., & Goedderz, A. 2020. Trait-unconsciousness, state-unconsciousness, preconsciousness, and social miscalibration in the context of implicit evaluation. Social Cognition 38, Supp.: S114–S134. https://guilfordjournals.com/doi/pdf/10.1521/soco.2020.38.supp.s115.
Haier, R. J. 2009. Cognition and the brain: Sex matters. In C. H. Sommers, ed., The Science on Women and Science. Washington, DC: The AEI Press. 190–201. https://www.aei.org/wp-content/uploads/2014/07/-the-science-on-women-and-science_160107817595.pdf.
Hanushek, E. A., Schwerdt, G., Wiederhold, S., & Woessmann, L. 2015. Returns to skills around the world: Evidence from PIAAC. European Economic Review 73: 103−130. https://doi.org/10.1016/j.euroecorev.2014.10.006.
Harris, R. 2017. Rigor Mortis: How Sloppy Science Creates Worthless Cures, Crushes Hope, and Wastes Billions. New York, NY: Basic Books.
Harrison, J., & Lakin, J. 2018. Mainstream teachers’ implicit beliefs about English language learners: An Implicit Association Test study of teacher beliefs. Journal of Language, Identity & Education 17, 2: 85–102. https://doi.org/10.1080/15348458.2017.1397520.
Hart, S. A., Petrill, S. A., Thompson, L. A., & Plomin, R. 2009. The ABCs of math: A genetic analysis of mathematics and its links with reading ability and general cognitive ability. Journal of Educational Psychology 101, 2: 388–402. https://doi.org/10.1037/a0015115.
Henry, P. J. 2021. A survey researcher’s response to the Implicit revolution: Listen to what people say. In J. A. Krosnick, T. H. Stark, & A. L. Scott, eds., The Cambridge Handbook of Implicit Bias and Racism. Cambridge, UK: Cambridge University Press. https://osf.io/y62ct.
Hirukawa, M., Murtazashvili, I., & Prokhorov, A. 2023. Yet another look at the omitted variable bias. Econometric Reviews 42, 1: 1−27. https://doi.org/10.1080/07474938.2022.2157965.
HPIO (Health Policy Institute of Ohio). 2022. States adopt policies to require implicit bias training for health workers. April 29, 2022. https://www.healthpolicynews.org/daily_review/2022/04/states-adopt-policies-to-require-implicit-bias-training-for-health-workers.html.
Hubbard, R. 2015. Corrupt Research: The Case for Reconceptualizing Empirical Management and Social Science. London, UK: Sage Publications.
Hughes, S., Cummins, J., & Hussey, I. 2023. Effects on the Affect Misattribution Procedure are strongly moderated by influence awareness. Behavior Research Methods 55: 1558–86. https://doi.org/10.3758/s13428-022-01879-4.
Hui, K., Sukhera, J., Vigod, S., Taylor, V. H., & Zaheer, J. 2020. Recognizing and addressing implicit gender bias in medicine. Canadian Medical Association Journal 192, 42: E1269–E1270. https://doi.org/10.1503/cmaj.200286.
Hur, M., Ware, R. L., Park, J., McKenna, A. M., Rodgers, R. P., Nikolau, B. J., ... Marshall, A. G. 2018. Statistically significant differences in composition of petroleum crude oils revealed by volcano plots generated from ultrahigh resolution Fourier transform ion cyclotron resonance mass spectra. Energy & Fuels 32, 2: 1206−12. https://doi.org/10.1021/acs.energyfuels.7b03061.
Hyde, J. S. 2005. The gender similarities hypothesis. American Psychologist 60, 6: 581–92. https://doi.org/10.1037/0003-066X.60.6.581.
Hyde, J. S., & Mertz, J. E. 2009. Gender, culture, and mathematics performance. Proceedings of the National Academy of Sciences 106, 22: 8801–7. https://doi.org/10.1073/pnas.0901265106.
Hyde, J. S., Bigler, R. S., Joel, D., Tate, C. C., & van Anders, S. M. 2019. The future of sex and gender in psychology: Five challenges to the gender binary. American Psychologist 74, 2: 171–93. https://doi.org/10.1037/amp0000307.
IBAW (Implicit Bias Awareness Workshop). N.d. New York City Department of Education. https://infohub.nyced.org/in-our-schools/programs/race-and-equity/equity-literacy/implicit-bias-awareness-workshops.
IBT (Implicit Bias Team). N. d. New York City Department of Education. https://web.archive.org/web/20220702133148/https://nycimplicitbias-workshop.com/meet-our-team/.
IHHA (Illinois Health and Hospital Association). 2022. Upcoming Mandatory Implicit Bias Training for Healthcare Providers, March 29, 2022. https://web.archive.org/web/20240221220951/https://www.team-iha.org/quality-and-safety/physicians-and-advanced-practice-providers/mandatory-implicit-bias-training.
ILCS (Illinois Compiled Statutes) 20 ILCS 2105/2105-15.7. N.d. Implicit bias awareness training. https://www.ilga.gov/legislation/ilcs/fulltext.asp?DocName=002021050K2105-15.7.
ILCS (Illinois Compiled Statutes) 50 ILCS 705/7. N.d. Illinois Police Training Act. https://www.ilga.gov/legislation/ilcs/fulltext.asp?DocName=005007050K7.
IM (Institute of Medicine (US) Committee on Understanding and Eliminating Racial and Ethnic Disparities in Health Care, Smedley, B. D., Stith, A. Y., & Nelson, A. R., eds.). 2003. Unequal Treatment: Confronting Racial and Ethnic Disparities in Health Care. National Academies Press (US).
Ioannidis, J. P. A. 2005. Why most published research findings are false. PLoS Medicine 2, 8: e124. https://doi.org/10.1371/journal.pmed.0020124.
Ioannidis, J P. A. 2022. Correction: Why most published research findings are false. PLoS Medicine 19, 8: e1004085. https://doi.org/10.1371/journal.pmed.1004085. Erratum for: PLoS Medicine 2, 8: e124.
IPA (Illinois Public Act) 100-0014. N.d. An Act Concerning Education. https://www.ilga.gov/legislation/publicacts/fulltext.asp?Name=100-0014&GA=100.
IPA (Illinois Public Act) 102-0004. N.d. Illinois Health Care and Human Service Reform Act. https://www.ilga.gov/legislation/publicacts/102/PDF/102-0004.pdf.
IPA (Illinois Public Act) 102-0604. N.d. An Act Concerning Children. https://ilga.gov/legislation/publicacts/fulltext.asp?Name=102-0604.
IPG (Interagency Policy Group on Increasing Diversity in the STEM Workforce by Reducing the Impact of Bias). 2016. Reducing the Impact of Bias in the STEM Workforce: Strengthening Excellence and Innovation. Office of Science and Technology Policy (OSTP) and Office of Personnel Management (OPM). November 2016. https://www.si.edu/content/OEEMA/OSTP-OPM_ReportDigest.pdf.
IQA (Information Quality Act). 2001. Public Law 106—554, Sec. 515.
Jena, A. B., Khullar, D., Ho, O., Olenski, A. R., & Blumenthal, D. M. 2015. Sex differences in academic rank in US medical schools in 2014. Journal of the American Medical Association 314, 11: 1149–58. https://doi.org/10.1001/jama.2015.10680.
Joe, I. O. 2020. Regulating implicit bias in the federal criminal process. California Law Review 108, 3. https://doi.org/10.15779/Z38RN3080X.
Johnson, V. E. 2013. Revised standards for statistical evidence. Proceedings of the National Academy of Sciences 110, 48: 19313−17. https://doi.org/10.1073/pnas.1313476110.
Jolls, C., & Sunstein, C. R. 2006. The law of implicit bias. California Law Review 94, 4: 969–96. https://law.yale.edu/sites/default/files/documents/pdf/The_Law_of_Implicit_Bias.pdf.
Jussim, L., Stevens, S. T., & Honeycutt, N. 2018. Unasked questions about stereotype accuracy. Archives of Scientific Psychology 6, 1: 214–29. https://doi.org/10.1037/arc0000055.
Jussim, L., Careem, A., Goldberg, Z. Honeycutt, N., & Stevens, S. T. 2020a. IAT Scores, Racial Gaps, and Scientific Gaps. In J. A. Krosnick, T. H. Stark, & A. L. Scott, eds., The Future of Research on Implicit Bias (Cambridge, UK: Cambridge University Press, forthcoming). https://osf.io/4nhdm.
Jussim, L. 2020b. Implicit Bias: Racial Gaps and Scientific Gaps. OSFHome. https://osf.io/vmd38.
Jussim, L., Thulin, E., Fish, J., & Wright, J. D. 2023. Articles Critical of the IAT and Implicit Bias. OFSHome. https://osf.io/74whk/.
Kahn, L. M. 2018. Permanent jobs, employment protection, and job content. Industrial Relations: A Journal of Economy and Society 57, 3: 469−538. https://doi.org/10.1111/irel.12209.
Kang, J., Bennett, M., Carbado, D., Casey, P., Dasgupta, N., Faigman, D., ... Mnookin, J. 2012. Implicit bias in the courtroom. UCLA Law Review 59: 1124−86. https://www.uclalawreview.org/pdf/59-5-1.pdf.
Kang, J. 2014. Implicit bias and segregation: Facing the enemy. NYU Furman Center: The Dream Revisited. August 2014. https://furmancenter.org/research/iri/essay/implicit-bias-and-segregation-facing-the-enemy.
Kindzierski, W., Young, S., Meyer, T., & Dunn, J. 2021. Evaluation of a meta-analysis of ambient air quality as a risk factor for asthma exacerbation. Journal of Respiration 1, 3: 173−96. https://doi.org/10.3390/jor1030017.
Kuhn, E. 2016. Science and deference: The “best available science” mandate is a fiction in the ninth circuit. Harvard Environmental Law Review, November 7, 2016. https://harvardelr.com/2016/11/07/elrs-science-and-deference-the-best-available-science-mandate-is-a-fiction-in-the-ninth-circuit/.
Kuncel, N. R., & Hezlett, S. A. 2010. Fact and fiction in cognitive ability testing for admissions and hiring decisions. Current Directions in Psychological Science 19, 6: 339−45. https://doi.org/10.1177/0963721410389459.
Kurdi, B., Seitchik, A. E., Axt, J. R., Carroll, T. J., Karapetyan, A., Kaushik, N., ... Banaji, M. R. 2019a. Relationship between the Implicit Association Test and intergroup behavior: A meta-analysis. American Psychologist 74, 5: 569–86. Advance online publication. http://doi.org/10.1037/amp0000364. Data and other materials are at Open Science Framework (OSF) at https://osf.io/47xw8/.
Kurdi, B., & Banaji, M. R. 2019b. Relationship between the Implicit Association Test and explicit measures of intergroup cognition: Data from the meta-analysis by Kurdi et al. (2018). https://banaji.sites.fas.harvard.edu/research/publications/articles/2019_Kurdi_ICE.pdf.
Kyoung Ro, H., Lattuca, L. R., & Alcott, B. 2017. Who goes to graduate school? Engineers’ math proficiency, college experience, and self-assessment of skills. Journal of Engineering Education 106, 1: 98–122. https://doi.org/10.1002/jee.20154.
Lai, C. K., & Wilson, M. E. 2021. Measuring implicit intergroup biases. Social and Personality Psychology Compass 15, 1, e12573. https://doi.org/10.1111/spc3.12573.
Lai, C. K., & Lisnek, J. A. 2023. The impact of implicit bias-oriented diversity training on police officers’ beliefs, motivations, and actions. Psychological Science. https://osf.io/58zsn.
Lamiell, J. T. 2019. Psychology’s Misuse of Statistics and Persistent Dismissal of Its Critics. Palgrave Studies in the Theory and History of Psychology. Palgrave Macmillan. https://doi.org/10.1007/978-3-030-12131-0.
LeBel, E. P., & Paunonen, S. V. 2011. Sexy but often unreliable: The impact of unreliability on the replicability of experimental findings with implicit measures. Personality & Social Psychology Bulletin 37, 4: 570–83. https://doi.org/10.1177/0146167211400619.
LegiScan. (N.d.) “Implicit Bias.” https://legiscan.com/gaits/search?state=ALL&keyword=%22implicit+bias%22.
Levy, J., & Kimura, D. 2009. Women, men, and the sciences. In C. H. Sommers, ed., The Science on Women and Science. Washington, DC: The AEI Press. 202–84. https://www.aei.org/wp-content/uploads/2014/07/-the-science-on-women-and-science_160107817595.pdf.
Li, W., Freudenberg, J., Suh, Y. J., & Yang, Y. 2014. Using volcano plots and regularized-chi statistics in genetic association studies. Computational Biology and Chemistry 48: 77−83. https://doi.org/10.1016/j.compbiolchem.2013.02.003.
Lieber, L. D. 2007. EEOC targets ‘unconscious bias.’ Law.com, July 30, 2007. https://www.lawjournalnewsletters.com/2007/07/30/eeoc-targets-unconscious-bias/.
Machery, E. 2022a. Anomalies in implicit attitudes research. Wiley Interdisciplinary Reviews. Cognitive Science 13, 1, e1569. https://doi.org/10.1002/wcs.1569.
Machery, E. 2022b. Anomalies in implicit attitudes research: Not so easily dismissed. Wiley Interdisciplinary Reviews. Cognitive Science 13, 3, e1591. https://doi.org/10.1002/wcs.1591.
Maina, I. W., Belton, T. D., Ginzberg, S., Singh, A., & Johnson, T. J. 2018. A decade of studying implicit racial/ethnic bias in healthcare providers using the implicit association test. Social Science & Medicine 199: 219–29. https://doi.org/10.1016/j.socscimed.2017.05.009.
Malecki Brooks Ford. N.d. New Illinois Law Requires Implicit Bias Training for Health Professionals. https://www.mbhealthlaw.com/new-illinois-law-requires-implicit-bias-training-for-health-professionalsea96a010.
Meissner, F., Grigutsch, L. A., Koranyi, N., Müller, F., & Rothermund, K. 2019. Predicting behavior with implicit measures: Disillusioning findings, reasonable explanations, and sophisticated solutions. Frontiers in Psychology 10, 2483. https://doi.org/10.3389/fpsyg.2019.02483.
Michaels, P. J. 2008. Evidence for “publication bias” concerning global warming in Science and Nature. Energy & Environment 19, 2: 287−301. http://journals.sagepub.com/doi/abs/10.1260/095830508783900735?journalCode=eaea.
Minnesota Statutes 144.1461. 2022. Dignity in Pregnancy and Childbirth. https://www.revisor.mn.gov/statutes/cite/144.1461.
Mitchell, G., & Tetlock, P. E. 2017. Popularity as a poor proxy for utility: The case of implicit prejudice. In S. O. Lilienfeld & I. D. Waldman, eds., Psychological Science under Scrutiny: Recent Challenges and Proposed Solutions. 164–95. Wiley Blackwell. https://doi.org/10.1002/9781119095910.ch10.
Mitchell, P. G., & Tetlock, P. E. 2024. Stretching the limits of science: Was the implicit-bias debate social psychology’s bridge too far? In J. A. Krosnick, T. H. Stark, & A. L. Scott, eds., The Cambridge Handbook of Implicit Bias and Racism. Cambridge, UK: Cambridge University Press.
Morris, M. L. 2016. Vocational interests in the United States: Sex, age, ethnicity, and year effects. Journal of Counseling Psychology 63, 5: 604–15. https://doi.org/10.1037/cou0000164.
MSMS (Michigan State Medical Society). 2021. State requires anti-bias training for health professionals. https://www.msms.org/About-MSMS/News-Media/state-requires-anti-bias-training-for-health-professionals.
Nalty, K. 2016. Strategies for confronting unconscious bias. The Colorado Lawyer 45, 5: 45–52. https://kathleennaltyconsulting.com/wp-content/uploads/2016/05/Strategies-for-Confronting-Unconscious-Bias-The-Colorado-Lawyer-May-2016.pdf.
NASEM (National Academies of Sciences, Engineering, and Medicine). 2016. Statistical Challenges in Assessing and Fostering the Reproducibility of Scientific Results: Summary of a Workshop. Washington, DC: The National Academies Press. https://nap.nationalacademies.org/read/21915/chapter/1.
NASEM (National Academies of Sciences, Engineering, and Medicine). 2019. Reproducibility and Replicability in Science. Washington, DC: The National Academies Press. https://nap.nationalacademies.org/read/25303/chapter/1.
NCSC (National Center for State Courts). N.d. Addressing Implicit Bias in the Courts. https://www.nccourts.gov/assets/inline-files/public-trust-12-15-15-IB_Summary_033012.pdf?VersionId=q_DMMIVv0v_eDJUa1ADxtw59Zt_svPgl?q_DMMIVv0v_eDJUa1ADxtw59Zt_svPgl.
New Jersey. 2020. Governor Murphy Signs Legislation Requiring Implicit Bias and Cultural Diversity Training for Law Enforcement Officers. August 27, 2020. https://nj.gov/governor/news/news/562020/approved/20200827d.shtml.
Nissen, S. B., Magidson, T., Gross, K., & Bergstrom C. T. 2016. Research: Publication bias and the canonization of false facts. eLife 5: e21451. https://doi.org/10.7554/eLife.21451.
N.J. Stat. § 18A:35-4.36a. N.d. CaseText. https://casetext.com/statute/new-jersey-statutes/title-18a-education/chapter-18a35-2-year-course-of-study-in-history/section-18a35-436a-curriculum-to-contain-instruction-on-diversity-and-inclusion.
NNU (National Nurses United). 2021. New state law tackles implicit bias in nursing education. National Nurse Magazine, December 2021. https://www.nationalnursesunited.org/article/new-state-law-tackles-implicit-bias-in-nursing-education.
NSF (News Service of Florida). 2022. A new law could transform anti-bias training on Florida campuses. WUSF, June 25, 2022. https://wusfnews.wusf.usf.edu/politics-issues/2022-06-25/a-new-law-could-transform-anti-bias-training-on-florida-campuses.
NSF NCSES (National Science Foundation, National Center for Science and Engineering Statistics). 2019. Women, Minorities, and Persons with Disabilities in Science and Engineering: 2019. Special report NSF 19-304. https://ncses.nsf.gov/pubs/nsf19304/data.
Nuttgens, S. 2023. Making psychology “count”: On the mathematization of psychology. Europe’s Journal of Psychology 19, 1: 100–12. https://doi.org/10.5964/ejop.4065.
OAG (Office of the Attorney General). 2020. Implicit Bias Training Hosted by the New Hampshire Attorney General’s Office. New Hampshire Department of Justice. https://web.archive.org/web/20220813002746/https://www.doj.nh.gov/implicit-bias-training/index.htm.
Ollove, M. 2022. With implicit bias hurting patients, some states including Maryland, train doctors. Maryland Matters, April 25, 2022. https://www.marylandmatters.org/2022/04/25/with-implicit-bias-hurting-patients-some-states-including-maryland-train-doctors/.
Onyeador, I. N., Hudson, S. T. J., & Lewis, N. A., Jr. 2021. Moving beyond implicit bias training: Policy insights for increasing organizational diversity. Policy Insights from the Behavioral and Brain Sciences 8, 1. https://journals.sagepub.com/doi/full/10.1177/2372732220983840.
Ordinance 71-19. 2019. City and County of San Francisco, April 19, 2019. https://sfgov.legistar.com/View.ashx?M=F&ID=7179259&GUID=90611A31-A8DC-4B32-A314-16F2E846BC81.
Oswald, F. L., Mitchell, G., Blanton, H., Jaccard, J., & Tetlock, P. E. 2013a. Predicting ethnic and racial discrimination: A meta-analysis of IAT criterion studies. Journal of Personality and Social Psychology 105, 2: 171–92. https://doi.org/10.1037/a0032734.
Oswald, F. L., Mitchell, G., Blanton, H., Jaccard, J., & Tetlock, P. E. 2013b. Supplemental material for Predicting ethnic and racial discrimination: A meta-analysis of IAT criterion studies. https://supp.apa.org/psycarticles/supplemental/a0032734/a0032734_supp.html.
Oswald, F. L., Mitchell, G., Blanton, H., Jaccard, J., & Tetlock, P. E. 2015. Using the IAT to predict ethnic and racial discrimination: Small effect sizes of unknown societal significance. Journal of Personality and Social Psychology 108, 4: 562–71. https://doi.org/10.1037/pspa0000023.
Paluck, E. L., Porat, R., Clark, C. S., & Green, D. P. 2021. Prejudice reduction: Progress and challenges. Annual Review of Psychology 72: 533–60. https://doi.org/10.1146/annurev-psych-071620-030619.
Payne, K., Niemi, L., & Doris, J. M. 2018. How to think about ‘implicit bias.’ Scientific American, March 27, 2018. https://www.scientificamerican.com/article/how-to-think-about-implicit-bias/.
Potier, B. 2004. Making case for concept of ‘implicit prejudice.’ Harvard Gazette, December 16, 2004. https://news.harvard.edu/gazette/story/2004/12/making-case-for-concept-of-implicit-prejudice/.
RAND Corporation. 2023. Diversity, Equity, and Inclusion at RAND. RAND Corporation, Santa Monica, CA. https://www.rand.org/about/diversity-equity-inclusion.html.
Randall, D., & Welser, C. 2018. The Irreproducibility Crisis of Modern Science: Causes, Consequences, and the Road to Reform. New York, NY: National Association of Scholars. https://www.nas.org/reports/the-irreproducibility-crisis-of-modern-science/full-report.
Randall, D. 2023. The implicit-bias house of cards. City Journal, October 3, 2023. https://www.city-journal.org/article/the-implicit-bias-house-of-cards.
van Ravenzwaaij, D., van der Maas, H. L. J., & Wagenmakers, E.-J. 2011. Does the name-race Implicit Association Test measure racial prejudice? Experimental Psychology 58, 4: 271–77. https://doi.org/10.1027/1618-3169/a000093.
Reber, A. S. 1989. Implicit learning of tacit knowledge. Journal of Experimental Psychology: General 118, 3: 219–35. https://doi.org/10.1037/0096-3445.118.3.219.
Redman, B. K. 2013. Research Misconduct Policy in Biomedicine: Beyond the Bad-Apple Approach. Cambridge, MA: The MIT Press.
Rezaei, A. R. 2011. Validity and reliability of the IAT: Measuring gender and ethnic stereotypes. Computers in Human Behavior 27, 5: 1937–41. https://doi.org/10.1016/j.chb.2011.04.018.
Ritchie, S. 2020. Science Fictions: How Fraud, Bias, Negligence, and Hype Undermine the Search for Truth. New York, NY: Henry Holt and Company.
Robstad, N., Westergren, T., Siebler, F., Söderhamn, U., & Fegran, L. 2019. Intensive care nurses’ implicit and explicit attitudes and their behavioural intentions towards obese intensive care patients. Journal of Advanced Nursing 75, 12: 3631–42. https://doi.org/10.1111/jan.14205.
Rothman, K. J. 1990. No adjustments are needed for multiple comparisons. Epidemiology 1, 1: 43–46. https://www.jstor.org/stable/pdf/20065622.pdf?seq=1.
Rounds, J., & Su, R. 2014. The nature and power of interests. Current Directions in Psychological Science 23, 2: 98−103. https://doi.org/10.1177/0963721414522812.
Rubinstein, R. S., Jussim, L., & Stevens, S. T. 2018. Reliance on individuating information and stereotypes in implicit and explicit person perception. Journal of Experimental Social Psychology 75: 54−70. https://doi.org/10.1016/j.jesp.2017.11.009.
Ryan, P. 2011. Impact of observational analysis design: Lessons from the Observational Medical Outcomes Partnership. http://www.niss.org/sites/default/files/OMOP_Ryan_NISS_16Jun2011.pdf.
Sailer, J. 2023. Inside Ohio State’s DEI factory. Wall Street Journal, November 20, 2023. https://www.wsj.com/articles/inside-ohio-states-dei-factory-faculty-report-diversity-hiring-cefd804d.
Sarewitz, D. 2012. Beware the creeping cracks of bias. Nature 485: 149. https://doi.org/10.1038/485149a.
Schimmack, U. 2019. The Implicit Association Test: A method in search of a construct. Perspectives on Psychological Science 16, 2: 396–414. https://doi.org/10.1177/1745691619863798.
Schimmack, U. 2021. Invalid claims about the validity of Implicit Association Tests by prisoners of the implicit social-cognition paradigm. Perspectives on Psychological Science 16, 2: 435–42. https://doi.org/10.1177/1745691621991860.
Schwarz, J. 1998. Roots of unconscious prejudice affect 90 to 95 percent of people, psychologists demonstrate at press conference. UW News, September 29, 1998. http://www.washington.edu/news/1998/09/29/roots-of-unconscious-prejudice-affect-90-to-95-percent-of-people-psychologists-demonstrate-at-press-conference.
Schweder, T., & Spjøtvoll, E. 1982. Plots of p-values to evaluate many tests simultaneously. Biometrika 69, 3: 493−502. https://doi.org/10.1093/biomet/69.3.493.
Skov, T. 2020. Unconscious gender bias in academia: Scarcity of empirical evidence. Societies 10, 2: 31. https://doi.org/10.3390/soc10020031.
Smedslund, J. 2021. From statistics to trust: Psychology in transition. New Ideas in Psychology 61, 100848. https://doi.org/10.1016/j.newideapsych.2020.100848.
Staddon, J. 2019. Object of inquiry: Psychology’s other (non-replication) problem. Academic Questions 32, 2: 246−56. https://dukespace.lib.duke.edu/server/api/core/bitstreams/6892c41a-655f-472e-96ea-75b9e40a5663/content.
Stark, J. 2021. Addressing implicit bias in policing. Police Chief Online, July 28, 2021. https://www.policechiefmagazine.org/addressing-implicit-bias-in-policing/.
State of Vermont. N.d. Overview of Employment Discrimination under Vermont Law. https://workplacesforall.vermont.gov/employers/responsibilities/overview-employment-discrimination-under-vermont-law.
Stewart-Williams, S., & Halsey, L. G. 2018. Men, women, and STEM: Why the differences and what should be done? (preprint). PsyArXiv. https://doi.org/10.31234/osf.io/ms524.
Stewart-Williams, S., & Halsey, L. G. 2021. Men, women and STEM: Why the differences and what should be done? European Journal of Personality 35, 1: 3–39. https://doi.org/10.1177/0890207020962326.
Stigler, S. M. 1992. A historical view of statistical concepts in psychology and educational research. American Journal of Education 101, 1: 60–70. http://www.medicine.mcgill.ca/epidemiology/hanley/bios601/Surveys/StiglerPsychStats.pdf.
Stoet, G., & Geary, D. C. 2022. Sex differences in adolescents’ occupational aspirations: Variations across time and place. PLoS One 17, 1: e0261438. https://doi.org/10.1371/journal.pone.0261438.
Su, R., Rounds, J., & Armstrong, P. I. 2009. Men and things, women and people: A meta-analysis of sex differences in interests. Psychological Bulletin 135, 6: 859–84. https://doi.org/10.1037/a0017364.
Su, R., & Rounds, J. 2015. All STEM fields are not created equal: People and things interests explain gender disparities across STEM fields. Frontiers in Psychology 6: 189. https://doi.org/10.3389/fpsyg.2015.00189.
Su, A. 2020. A proposal to properly address implicit bias in the jury. Hasting’s Women’s Law Journal 31, 1: 79–100. https://repository.uchastings.edu/cgi/viewcontent.cgi?article=1436&context=hwlj&%3A%7E%3Atext=Several%20courts%20have%20addressed%20the%2Cin%20the%20case%20at%20hand.
Sullivan, T. 2022. Illinois to require implicit bias awareness CME training. Policy & Medicine, July 17, 2022. https://www.policymed.com/2022/07/illinois-to-require-implicit-bias-awareness-cme-training.html.
Sulzer, S. H. 2022. Implicit bias and public health law. The Network for Public Health Law, January 11, 2022. https://www.networkforphl.org/news-insights/implicit-bias-and-public-health-law/.
Tan, L., Bradburn, I. S., Knight, D. B., Kinoshita, T., & Grohs, J. 2022. SAT patterns and engineering and computer science college majors: An intersectional, state-level study. International Journal of STEM Education 9, 1: 68. https://doi.org/10.1186/s40594-022-00384-6.
Tetlock, P. E., & Mitchell, G. 2009. Implicit bias and accountability systems: What must organizations do to prevent discrimination? Research in Organizational Behavior 29: 3–38. https://doi.org/10.1016/j.riob.2009.10.002.
TJMLLRG (Thomas J. Meskill Law Library Research Guides). N.d. Implicit Bias in the Law. UConn School of Law. https://libguides.law.uconn.edu/implicit.
TN HB0158. 2023. LegiScan. https://legiscan.com/TN/bill/HB0158/2023.
Unkelbach, C., & Fiedler, K. 2020. The challenge of diagnostic inferences from implicit measures: The case of non-evaluative influences in the evaluative priming paradigm. Social Cognition 38, Suppl.: S208–S222. https://doi.org/10.1521/soco.2020.38.supp.s208.
USDE (U.S. Department of Education). 2014. Guiding Principles: A Resource Guide for Improving School Climate and Discipline. https://files.eric.ed.gov/fulltext/ED544743.pdf.
USDJ (U.S. Department of Justice). 2016. Department of Justice Announces New Department-Wide Implicit Bias Training for Personnel. Justice News, June 27, 2016. https://www.justice.gov/opa/pr/department-justice-announces-new-department-wide-implicit-bias-training-personnel.
USDJ (U.S. Department of Justice). N.d. Understanding Bias: A Resource Guide. Community Relations Services Toolkit for Policing. https://www.justice.gov/file/1437326/download.
USM (Under Secretary for Management). 2019. Our Commitment to Diversity and Inclusion. November 22, 2019. https://2017-2021.state.gov/remarks-and-releases-under-secretary-for-management/our-commitment-to-diversity-and-inclusion/index.html.
Vankov, I., Bowers, J., & Munafò, M. R. 2014. On the persistence of low power in psychological science. Quarterly Journal of Experimental Psychology 67, 5: 1037–40. https://doi.org/10.1080/17470218.2014.885986.
Washington RCW 43.70.613. N.d. Health care professionals—Health equity continuing education. https://app.leg.wa.gov/rcw/default.aspx?cite=43.70.613#:~:text=This%20requires%20individual%20health%20care,the%20quality%20of%20care%20provided.
WBK (Weiner Brodsky Kider PC). 2022. Reminder: California DRE to require implicit bias training beginning jan. 2023. June 23, 2022. https://www.thewbkfirm.com/industry/reminder-california-dre-to-require-implicit-bias-training-beginning-jan-2023.
Weis, J. 2020. The rise of implicit bias training. Salud America! September 22, 2020. https://salud-america.org/the-rise-of-implicit-bias-training/.
Westfall, P. H., & Young, S. S. 1993. Resampling-Based Multiple Testing: Examples and Methods for p-Value Adjustment. New York, NY: John Wiley & Sons.
Whitmer, G. 2020. Executive Directive No. 2020-9, August 5, 2020. https://www.michigan.gov/whitmer/news/state-orders-and-directives/2020/08/05/executive-directive-2020-9.
Wicklin, R. 2017. Fisher’s transformation of the correlation coefficient. SAS Blogs, 20 September 2017. https://blogs.sas.com/content/iml/2017/09/20/fishers-transformation-correlation.html.
Williams, Z. 2022. New law aims to help New York’s first responders fight their ‘implicit bias.’ New York Post, May 6, 2022. https://nypost.com/2022/05/06/law-aims-to-help-ny-first-responders-fight-implicit-bias/.
Wilms, R., Mäthner, E., Winnen, L., & Lanwehr, R. 2021. Omitted variable bias: A threat to estimating causal relationships. Methods in Psychology 5: 100075. https://doi.org/10.1016/j.metip.2021.100075.
WIPR (World Intellectual Property Review). 2020. Hidden depths: The problem of unconscious bias. Sterne Kessler. November 4, 2020. https://www.sternekessler.com/news-insights/news/hidden-depths-problem-unconscious-bias.
Wittenbrink, B., Judd, C. M., & Park, B. 1997. Evidence for racial prejudice at the implicit level and its relationship with questionnaire measures. Journal of Personality and Social Psychology 72, 2: 262−74. https://doi.org/10.1037/0022-3514.72.2.262.
WMSID (Wal-Mart Stores, Inc. v. Dukes). 2011. Oyez. https://www.oyez.org/cases/2010/10-277.
Yong, E. 2018. Psychology’s replication crisis is running out of excuses. The Atlantic, November 19, 2018. https://www.theatlantic.com/science/archive/2018/11/psychologys-replication-crisis-real/576223/.
Young, S. S. 2008. Statistical analyses and interpretation of complex studies. Medscape. https://www.medscape.org/viewarticle/571523.
Young, S. S., & Kindzierski, W. B. 2019. Evaluation of a meta-analysis of air quality and heart attacks, a case study. Critical Reviews in Toxicology 49, 1: 85–94. https://doi.org/10.1080/10408444.2019.1576587.
Young, S. S., & Kindzierski, W. B. 2021a. Standard meta-analysis methods are not robust. arXiv:2110.14511 [stat.ME]. https://doi.org/10.48550/arXiv.2110.14511.
Young, S. S., Kindzierski, W., & Randall, D. 2021b. Shifting Sands: Unsound Science and Unsafe Regulation, Report 1. Keeping Count of Government Science: P-Value Plotting, P- Hacking, and PM2.5 Regulation. New York, NY: National Association of Scholars. https://www.nas.org/reports/shifting-sands-report-i/full-report.
Young, S. S., & Kindzierski, W. B. 2022a. Statistical reliability of a diet-disease association meta-analysis. International Journal of Statistics and Probability 11, 3: 40−50. https://doi.org/10.5539/ijsp.v11n3p40.
Young, S. S., Kindzierski, W. B., & Randall, D. 2022b. Shifting Sands: Unsound Science and Unsafe Regulation, Report 2. Flimsy Food Findings: Food Frequency Questionnaires, False Positives, and Fallacious Procedures in Nutritional Epidemiology. New York, NY: National Association of Scholars. https://www.nas.org/reports/shifting-sands-report-ii.
Young, S. S., & Kindzierski, W. B. 2023a. Research plan to examine the reliability of implicit bias. Submitted 2023-06-19. https://researchers.one/articles/23.06.00005.
Young, S. S., & Kindzierski, W. B. 2023b. Reproducibility of health claims in meta-analysis studies of COVID quarantine (stay-at-home) orders. International Journal of Statistics and Probability 12, 1: 54–65. https://doi.org/10.5539/ijsp.v12n1p54.
Young, S. S., Kindzierski, W., & Randall, D. 2023c. Shifting Sands: Unsound Science and Unsafe Regulation, Report 3. The Confounded Errors of Public Health Policy Response to the COVID-19 Pandemic. New York, NY: National Association of Scholars. https://www.nas.org/reports/shifting-sands-report-iii/full-report.
Young, S. S., & Kindzierski, W. B. 2024a. Reproducibility of Implicit Association Test (IAT) – Case study of meta-analysis of racial bias research claims. International Journal of Statistics and Probability 13, 2: 33–45. https://doi.org/10.5539/ijsp.v13n2p33.
Young, S. S., & Kindzierski, W. B. 2024b. Protocol: Evaluation of gender IAT reliability. Submitted 2024-02-20. https://researchers.one/articles/24.02.00004.
Ziegert, J. C., & Hanges, P. J. 2005. Employment discrimination: The role of implicit attitudes, motivation, and a climate for racial bias. Journal of Applied Psychology 90, 3: 553–62. https://doi.org/10.1037/0021-9010.90.3.553.
Zitelny, H., Shalom, M., & Bar-Anan, Y. 2017. What is the implicit gender-science stereotype? Exploring correlations between the gender-science IAT and self-report measures. Social Psychological and Personality Science 8, 7: 719–35. https://doi.org/10.1177/1948550616683017.
1 David Randall and Christopher Welser, The Irreproducibility Crisis of Modern Science: Causes, Consequences, and the Road to Reform (New York: National Association of Scholars, 2018), https://www.nas.org/reports/the-irreproducibility-crisis-of-modern-science.
2 “Fixing Science: Practical Solutions for the Irreproducibility Crisis,” National Association of Scholars and Independent Institute, February 21, 2020, YouTube, https://www.youtube.com/watch?v=eee6KloEUR4&list=PL-mariB2b6NugvvjAFeAjK-_-Y6wXCkvM; “Conference Follow-up: Fixing Science,” National Association of Scholars, February 19, 2020, https://www.nas.org/blogs/article/conference-follow-up-fixing-science.
3 “UPDATED: NAS Public Comment on Strengthening Transparency in Regulatory Science,” National Association of Scholars, June 19, 2018, https://www.nas.org/blogs/article/updated_nas_public_comment_on_strengthening_transparency_in_regulatory_scie; Peter Wood, “NAS Comments on EPA's Proposed Supplemental Notice of Proposed Rulemaking,” March 23, 2020, https://www.nas.org/blogs/article/nas-comment-on-epas-proposed-supplemental-notice-of-proposed-rulemaking; David Randall, “Comments on EPA’s Final Rule, ‘Strengthening Transparency,’” National Association of Scholars, January 12, 2021, https://www.nas.org/blogs/article/nas-comments-on-epas-final-rule-strengthening-transparency.
4 Peter Wood, “Episode #51: Rabble Rousing with Lee Jussim,” July 29, 2020, in Curriculum Vitae, MP3 audio, INSERT FULL LENGTH WHEN KNOWN, https://www.nas.org/blogs/media/episode-51-rabble-rousing-with-lee-jussim; David Randall, “Legally Wrong: When Courts and Science Meet with Nathan Schachtman,” in Curriculum Vitae, MP3 audio, 01:28:43, https://www.nas.org/blogs/media/legally-wrong-when-politics-and-science-meet-with-nathan-schactman; David Randall, “Bad Science Makes for Bad Government,” National Association of Scholars, September 19, 2019, https://www.nas.org/blogs/article/bad-science-makes-for-bad-government; Edward Reid, “Irreproducibility and Climate Science,” National Association of Scholars, May 17, 2018, https://www.nas.org/blogs/article/irreproducibility_and_climate_science.
5 S. Stanley Young, Warren Kindzierski, and David Randall, Shifting Sands: Unsound Science and Unsafe Regulation Series (National Association of Scholars, 2021–2024), https://www.nas.org/reports/shifting-sands-report-i.
6 E.g., J. Scott Turner, “To Rescue Science, We Must Turn Off the Funding Spigot,” Minding the Campus, June 25, 2024, https://www.mindingthecampus.org/2024/06/25/to-rescue-science-we-must-turn-off-the-funding-spigot/.
7 Schwarz (1998).
8 Mitchell (2017).
9 Greenwald (1995); WIPR (2020); Greenwald (2006).
10 Reber (1989).
11 Jussim (2020b).
12 Jussim (2020a).
13 Jussim (2020a).
14 Mitchell (2017).
15 Young (2021b); Young (2022b); Young (2023c).
16 IQA (2001); Kuhn (2016).
17 Young (2023c). See especially the “Technical Studies: Methods” section (https://www.nas.org/reports/shifting-sands-report-iii/full-report#TechnicalStudies:Methods) and the appendices (https://www.nas.org/reports/shifting-sands-report-iii/full-report#Appendices).
18 Benjamini (1995); Westfall (1993).
19 Young (2021b); and see Ryan (2011).
20 NASEM (2019); Rothman (1990).
21 Baker (2016); Sarewitz (2012).
22 Randall (2018); Young (2021b).
23 Al-Marzouki (2005); Couzin (2006); Redman (2013); Ritchie (2020).
24 Buchanan (2004); Young (2021b).
25 Baker (2016); Sarewitz (2012). See especially appendix three in Young (2023c) (https://www.nas.org/reports/shifting-sands-report-iii/full-report#Appendices).
26 Boos (2013).
27 Briggs (2017); Briggs (2019); Chambers (2017); Clyde (2000); Gelman (2014); Harris (2017); Hubbard (2015).
28 Benjamin (2018); Johnson (2013).
29 NASEM (2016); NASEM (2019).
30 Baker (2016).
31 Begley (2012); and see Diener (2018) [psychology]; Franco (2014) [social sciences]; Gerber (2008) [sociology]; and Michaels (2008) [climate science].
32 Gelman (2014).
33 NASEM (2016).
34 Young (2021b).
35 Young (2022b).
36 Young (2023c) Confounded Errors does not address p-hacking.
37 Greenwald (1995); WIPR (2020); Greenwald (2006).
38 Reber (1989).
39 Blanton (2023).
40 Mitchell (2017).
41 Banaji (2013).
42 Potier (2004).
43 Mitchell (2017).
44 Greenwald (2006).
45 TJMLLRG (N.d.); and see Elosiebo (2018); Joe (2020); Jolls (2006).
46 Kang (2014).
47 Grine (2017).
48 Grine (2017).
49 State of Vermont (N.d.).
50 Blevins (2017).
51 Kang (2014).
52 Cummins (2007).
53 EEOC (2008); WMSID (2011).
54 Borchelt (2019); Lieber (2007).
55 EEOC (2021).
56 USDJ (2016).
57 OAG (2020).
58 NCSC (N.d.).
59 CGC § 68088 (N.d.).
60 LegiScan (N.d.).
61 Bennett (2010); Elek (2013); Kang (2012); Nalty (2016).
62 Su (2020).
63 CGA SB22-128 (2022).
64 Bienias (2017).
65 Bienias (2017); and see Chivers (2022).
66 USDJ (N.d.).
67 CPC § 13519.4 (N.d.).
68 ILCS 50 ILCS 705/7 (N.d.)
69 New Jersey (2020).
70 Donyéa (2022).
71 LegiScan (N.d.). Also see Stark (2021).
72 USDE (2014).
73 IPA 100-0014 (N.d.).
74 Akbarzai (2021); N.J. Stat. § 18A:35-4.36a (N.d.).
75 IBAW (N.d.).
76 IBT (N.d.)
77 LegiScan (N.d.).
78 Biden (2021).
79 USM (2019).
80 CIA (2016).
81 Donyéa (2022).
82 Columbus (N.d.).
83 Ethics Commission (N.d.); Ordinance 71-19 (2019).
84 IM (2003); Ollove (2022).
85 CDCP (N.d.)
86 IM (2003); Ollove (2022).
87 Gaines (2021); NNU (2021).
88 ILCS 20 ILCS 2105/2105-15.7 (N.d.); IHHA (2022); IPA 102-0004 (N.d.); IPA 102-0604 (N.d.); 68 IAC 1130.500 (N.d.); Malecki Brooks Ford (N.d.); Sullivan (2022).
89 Bonessi (2021).
90 BRM (2021).
91 Egan (2020); MSMS (2021); Ollove (2022); Whitmer (2020).
92 Minnesota Statutes 144.1461 (2022); Ollove (2022).
93 Williams (2022).
94 Washington RCW 43.70.613 (N.d.).
95 Ollove (2022); IHHA (2022); Williams (2022).
96 LegiScan (N.d.); Ollove (2022).
97 HPIO (2022).
98 IPG (2016).
99 IPG (2016).
100 CSB 263 (2021); WBK (2022).
101 LegiScan (N.d.).
102 Onyeador (2021).
103 FHP (2020).
104 GSP (N.d.).
105 Chapter 2022-72 (2022); NSF (2022).
106 Aldrich (2021).
107 Aldrich (2023); TN HB0158 (2023).
108 LegiScan (N.d.).
109 Staddon (2019).
110 Danziger (1990); Lamiell (2019); Nuttgens (2023); Randall (2018); Smedslund (2021); Stigler (1992); Vankov (2014); Yong (2018).
111 Chin (2023).
112 Andreychik (2012).
113 Arkes (2004); and see Jussim (2020a).
114 Cone (2017).
115 Corneille (2020a).
116 Corneille (2020b); and see Hahn (2020).
117 Corneille (2022).
118 Cyrus-Lai (2022); and for the unjustified alignment of implicit bias theory with identity politics polemics, also see Mitchell (2017); Oswald (2015).
119 Jussim (2018).
120 Jussim (2020a).
121 Rubinstein (2018).
122 Skov (2020).
123 Blanton (2008); Jussim (2020b); Jussim (2023); Lai (2021); Mitchell (2017). Mitchell (2017) notes that, “to our knowledge, no research has sought to examine the many nontraditional implicit biases that may be implicated in an interaction and compare the behavioral influence of the nontraditional biases to that of the traditional implicit biases.”
124 Gawronski (2019).
125 Gawronski (2022).
126 Cesario (2022).
127 Anselmi (2011).
128 Blanton (2009); and see Carlsson (2016); Henry (2021); Oswald (2013a); Oswald (2015).
129 Blanton (2015a); and see Chequer (2021); Schimmack (2021).
130 Blanton (2015b).
131 Blanton (2017).
132 Bluemke (2009).
133 van Dessel (2020).
134 Fiedler (2006).
135 Forscher (2019).
136 Hahn (2014).
137 LeBel (2011); and see Rezaei (2011).
138 Hughes (2023).
139 Oswald (2015).
140 van Ravenzwaaij (2011).
141 Unkelbach (2020).
142 Blanton (2008); Blanton (2023); Jussim (2020a); Jussim (2020b); Jussim (2023); Lai (2021); Meissner (2019).
143 Blanton (2023).
144 Henry (2021).
145 Mitchell (2017).
146 Jussim (2020a).
147 Blanton (2023).
148 Machery (2022a); and see Machery (2022b).
149 Paluck (2021).
150 Lai (2023).
151 Cabinet Office (2020).
152 Payne (2018); Sulzer (2022).
153 Greenwald (2022).
154 Bartels (2021).
155 Chin (2023).
156 Young (2023c). See especially the “Technical Studies: Methods” section (https://www.nas.org/reports/shifting-sands-report-iii/full-report#TechnicalStudies:Methods) and the appendices (https://www.nas.org/reports/shifting-sands-report-iii/full-report#Appendices).
157 Su (2009).
158 Greenwald (1998).
159 Blanton (2009).
160 Wittenbrink (1997).
161 Oswald (2013a).
162 Google Scholar (2024b).
163 Maina (2018).
164 Harrison (2018).
165 Ziegert (2005).
166 Bass (2021).
167 E.g., RAND Corporation (2023).
168 Sailer (2023).
169 Randall (2023).
170 E.g., Blanton (2009); Fiedler (2006); Mitchell (2024); Oswald (2013a); Oswald (2015); Schimmack (2019); Schimmack (2021).
171 Schweder (1982).
172 Young (2023a).
173 Oswald (2013a).
174 Oswald (2013b). These consisted of correlation coefficient (r) values and sample sizes (n) for the individual studies used in their meta-analysis for: 1) IAT−real-world behavior correlations, and 2) explicit bias−real-world behavior correlations.
175 Egger (2001).
176 Boos (2013).
177 Young (2019); Kindzierski (2021); Young (2022a); Young (2023b).
178 We used Fisher’s test of a correlation method, also known as Fisher’s Z-transformation. Fisher’s Z-transformation is a statistical technique used to compute a p-value for a correlation coefficient r given sample size n. The formula for Fisher’s Z-transformation is: �� = ������������ℎ(��) = 0.5 ln [(1+��)/(1−��)], which is considered to follow a normal distribution with standard error (SE) of: ���� = �������� (1/(��−3)). We then converted the average ��−����������/SE back into a p-value using the standard normal distribution. Fisher (1921); Wicklin (2017).
179 Schweder (1982); Google Scholar (2024a).
180 Oswald (2013a).
181 Fisher (1925); Young (2021a).
182 Oswald (2013b).
183 Benjamini (1995).
184 After Benjamini (1995).
185 Oswald (2013a); Oswald (2015).
186 Benjamini (1995).
187 Fisher (1925); Young (2021a).
188 Oswald (2015).
189 Oswald (2013a).
190 Fisher (1925); Young (2021a).
191 Benjamini (1995).
192 Oswald (2013b).
193 Fisher (1925); Young (2021a).
194 Benjamini (1995).
195 Oswald (2013a).
196 Schimmack (2019); Schimmack (2021).
197 Dang (2020).
198 Dehon (2017).
199 Schimmack (2021).
200 Nissen (2016).
201 Stewart-Williams (2021).
202 Van den Brink (2011).
203 E.g., Bosak (2023); Hyde (2005); Hyde (2009); Hyde (2019).
204 Farrell (2017); Girod (2016); Hui (2020).
205 Greenwald (1998).
206 Aidman (2003); Greenwald (2000).
207 Zitelny (2017).
208 Young (2024a).
209 Kurdi (2019a); Kurdi (2019b).
210 Kurdi (2019a).
211 Schweder (1982).
212 Kurdi (2019a).
213 Rounds (2015).
214 Su (2009).
215 Young (2024b).
216 Egger (2001).
217 Boos (2013).
218 Kindzierski (2021); Young (2019); Young (2022a); Young (2023b).
219 Kurdi (2019a); Kurdi (2019b).
220 Fisher’s Z-transformation is a statistical technique used to compute a p-value for a correlation coefficient r given sample size n. The formula for Fisher’s Z-transformation is: �� = ������������ℎ(��) = 0.5 ln [(1+��)/(1−��)], which is considered to follow a normal distribution with standard error (SE) of: ���� = �������� (1/(��−3)). We then converted the average ��−����������/SE back into a p-value using the standard normal distribution. Fisher (1921); Wicklin (2017).
221 Su (2009).
222 Schweder (1982); Google Scholar (2024a).
223 E.g., Ioannidis (2005); Ioannidis (2022); Schimmack (2021).
224 E.g., Arkes (2004); Blanton (2006); Mitchell (2017); Oswald (2013a); Oswald (2015); Schimmack (2019); Schimmack (2021); Tetlock (2009).
225 Li (2014); Hur (2018). We constructed our volcano plot by graphing the negative log10 of the p-value on the y axis against the d value on the x axis. A consistent dataset in a volcano plot should resemble an erupting volcano. Data points with low p-values (highly statistically significant results) appear toward the top of the plot. Data points in the top-right and top-left areas of a volcano plot are of interest because they are the most different between the two conditions of interest—i.e., results with ds > 0 on the right versus those with ds < 0 on the left.
226 Kurdi (2019a); Kurdi (2019b).
227 Su (2009).
228 Blanton (2009); Fiedler (2006); Mitchell (2024); Oswald (2013a); Oswald (2015); Schimmack> (2019); Schimmack (2021).
229 Schimmack (2021).
230 Young (2024a).
231 Su (2009).
232 Wilms (2021); Hirukawa (2023); Basu (2024).
233 Linear regression is widely used to help understand real-world situations—for example, the association between one predictor variable and one outcome (or response) variable. Keep in mind that a regression model is just that: a model. Once the model is made, it is still a model, an approximation to the world.
First, let’s consider simple linear regression models; one model for females and another for males:
Female: Yf = β0 + β1X1f
Male: Ym = β0 + β1X1m
The above models say that an outcome, Y, is predictable using a linear additive function of an intercept, β0, and β1X1, and that there is a random error in the prediction captured by ε. Y is an outcome variable, and X is a predictor variable of interest. The subscripts “f” and “m” denote females and males, respectively.
Now let’s consider a specific example of the percentage of full professors in medical schools. Let Y be the percentage of full professors in medical schools; let X be a predictor variable. The subscripts “f” and “m” denote females and males, respectively. The regression models can be expanded by adding more variable (X) terms:
Female: Yf = β0+β1X1f+β2X2f +β3X3f +β4X4f+ … +βpXpf
Male: Ym = β0+β1X1m+β2X2m+β3X3m+β4X4m+ … +βpXpm
The above mathematical models indicate that the percentage of female/male full professors in medical schools, Y, is predictable using a linear additive function of the variables X1, X2, X3, X4,
…Xp, specific to females (or males) and a random error term ε. Here, X1 is the predictor variable of interest, and the other variables—X2, X3, X4, ...Xp—are said to be confounding factors. As before, the subscripts “f” and “m” denote females and males, respectively.
Let’s say we are interested in looking at differences between female and male full professors in medical schools, Yf – Ym. Using a difference between two multiple linear regression models, Young showed that there is the potential for residual bias in multiple linear regression if the models omit important terms (i.e., unknown confounders):
(Yf – Ym) − [known confounders, i.e., X2, X3, X4, ...Xp] = β1(X1f − X1m) + [unknown confounders]
Young (2008).
234 Carr (2018); Jena (2015).
235 NSF NCSES (2019).
236 Farrell (2017); Girod (2016); Hui (2020).
237 Haier (2009).
238 Coyle (2018); Gottfredson (2003); Levy (2009); Stewart-Williams (2018).
239 Su (2009).
240 Rounds (2014).
241 Su (2009).
242 Morris (2016). For our example in Figure 10, we assumed similar standard deviations for both female and male distributions for simplicity (i.e., these distributions are equally spread out). Males are used as the reference point in Figure 10, i.e., the distribution for males has mean, µ = 0 and standard deviation, σ = 1, while the distribution for females has µ = −0.93[242] and σ = 1.00 (assumed).
243 After Su (2009).
244 Stoet (2022); Su (2015).
245 Tan (2022).
246 Kyoung Ro (2017).
247 Hart (2009).
248 Gottfredson (2002); Kuncel (2010).
249 Hanushek (2015); Kahn (2018).
250 College Board (2016).
251 After College Board (2016).
252 College Board (2016).
253 Kurdi (2019a); Kurdi (2019b).
254 Su (2009).
255 USDE (2014).
256 Robstad (2019).
257 Fisher (1921); Wicklin (2017).
258 Freichel (2024).
- Preface and Acknowledgements
- Executive Summary
- Introduction
- The Career of Implicit Bias Theory
- The Emperor Has No Clothes: Critiques of Implicit Bias Theory
- Technical Studies: Contribution to the Scholarly Conversation
- Technical Study #1: The Reproducibility of the Implicit Association Test (IAT): Meta-Analysis of Racial Bias Research Claims
- Technical Study #2: The Reliability of the Sex Implicit Association Test (sIAT) for High-Ability Careers
- Recommendations
- Conclusion
- Appendix 1: The IAT and Real-World Behavior
- References